Text classification of student predicate use for automatic misconception categorization.
Frontiers in Education Conference(2018)
摘要
This Research Category Full Paper presents an approach for categorizing student misconceptions about dynamics and heat transfer using text classification. Research in educational engineering describes how science concepts can be ontologically categorized into two major categories (substances and processes) according to their nature. Students can acquire misconceptions by incorrectly categorizing concepts. Predicate tests help to determine when misconceptions have occurred and aid in customizing curriculum content. However predicate tests rely on time-consuming, manual labor. The main goal of this research was to show how predicate tests could be automated with text classification models using a previously annotated dataset. We compared classifier performance between WEKA's Support Vector Machines, Multinomial Naive Bayes, Logistic Regression, and Bayesian Logistic Regression implementations using the emergent process and sequential process ontological categories as labels. We compared model performance using WEKA's N-Gram tokenizer with 3-grams vs. using Java's WordTokenizer to convert our word dataset to numerical vectors. Models were evaluated using 10-fold cross-validation considering accuracy, F-measure, and kappa coefficient as measures of performance. We have shown the feasibility of using text classification for misconception assessment. Our implementation of predicate test automation can play an important role in speeding up misconception assessment research and curriculum design research.
更多查看译文
关键词
Data Science,Misconception Categorization,Assessment and Evaluation,Learning Analytics,Infrastructure and Technologies for Engineering Education,Computer-Based Learning and Courseware Technologies,Engineering Education Research
AI 理解论文
溯源树
样例
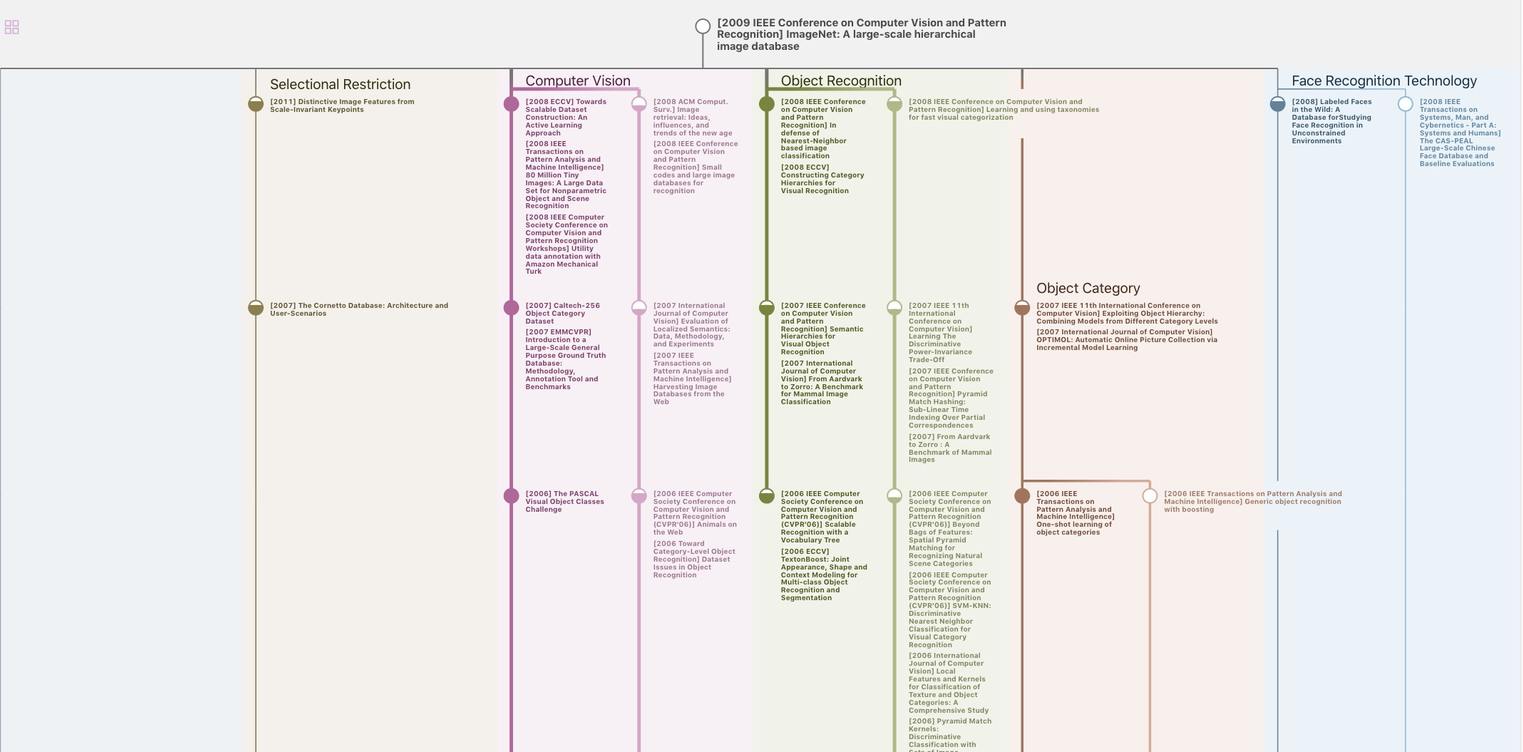
生成溯源树,研究论文发展脉络
Chat Paper
正在生成论文摘要