TETRIS: Template Transformer Networks for Image Segmentation with Shape Priors.
IEEE transactions on medical imaging(2019)
摘要
In this paper we introduce and compare different approaches for incorporating shape prior information into neural network based image segmentation. Specifically, we introduce the concept of template transformer networks where a shape template is deformed to match the underlying structure of interest through an end-to-end trained spatial transformer network. This has the advantage of explicitly enforcing shape priors and is free of discretisation artefacts by providing a soft partial volume segmentation. We also introduce a simple yet effective way of incorporating priors in state-of-the-art pixel-wise binary classification methods such as fully convolutional networks and U-net. Here, the template shape is given as an additional input channel, incorporating this information significantly reduces false positives. We report results on synthetic data and sub-voxel segmentation of coronary lumen structures in cardiac computed tomography showing the benefit of incorporating priors in neural network based image segmentation.
更多查看译文
关键词
Shape,Neural networks,Image segmentation,Strain,Deformable models,Training,Task analysis
AI 理解论文
溯源树
样例
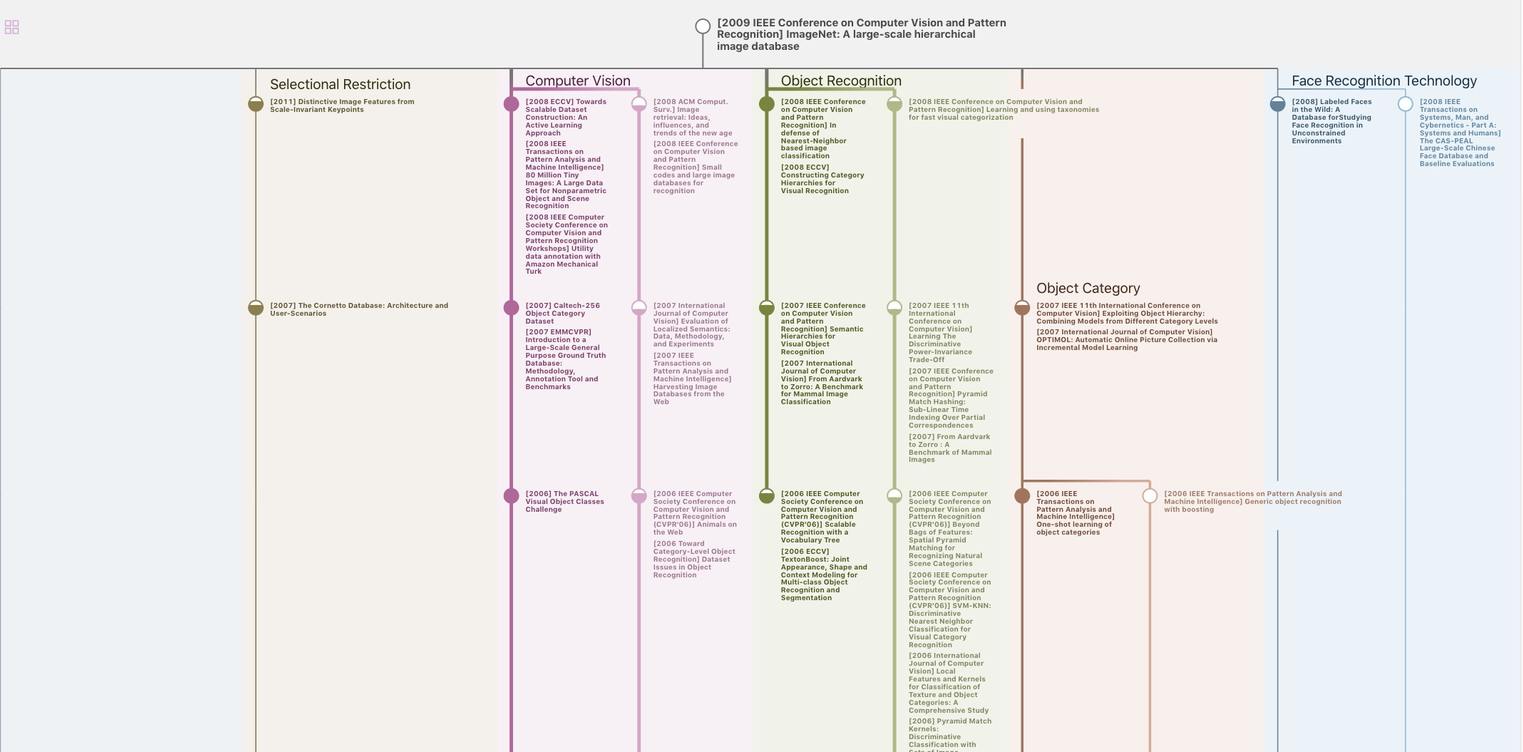
生成溯源树,研究论文发展脉络
Chat Paper
正在生成论文摘要