Defocus Blur Detection via Multi-Stream Bottom-Top-Bottom Network.
IEEE Transactions on Pattern Analysis and Machine Intelligence(2020)
摘要
Defocus blur detection (DBD) is aimed to estimate the probability of each pixel being in-focus or out-of-focus. This process has been paid considerable attention due to its remarkable potential applications. Accurate differentiation of homogeneous regions and detection of low-contrast focal regions, as well as suppression of background clutter, are challenges associated with DBD. To address these issues, we propose a multi-stream bottom-top-bottom fully convolutional network (BTBNet), which is the first attempt to develop an end-to-end deep network to solve the DBD problems. First, we develop a fully convolutional BTBNet to gradually integrate nearby feature levels of bottom to top and top to bottom. Then, considering that the degree of defocus blur is sensitive to scales, we propose multi-stream BTBNets that handle input images with different scales to improve the performance of DBD. Finally, a cascaded DBD map residual learning architecture is designed to gradually restore finer structures from the small scale to the large scale. To promote further study and evaluation of the DBD models, we construct a new database of 1100 challenging images and their pixel-wise defocus blur annotations. Experimental results on the existing and our new datasets demonstrate that the proposed method achieves significantly better performance than other state-of-the-art algorithms.
更多查看译文
关键词
Feature extraction,Image edge detection,Streaming media,Semantics,Clutter,Image restoration,Deep learning
AI 理解论文
溯源树
样例
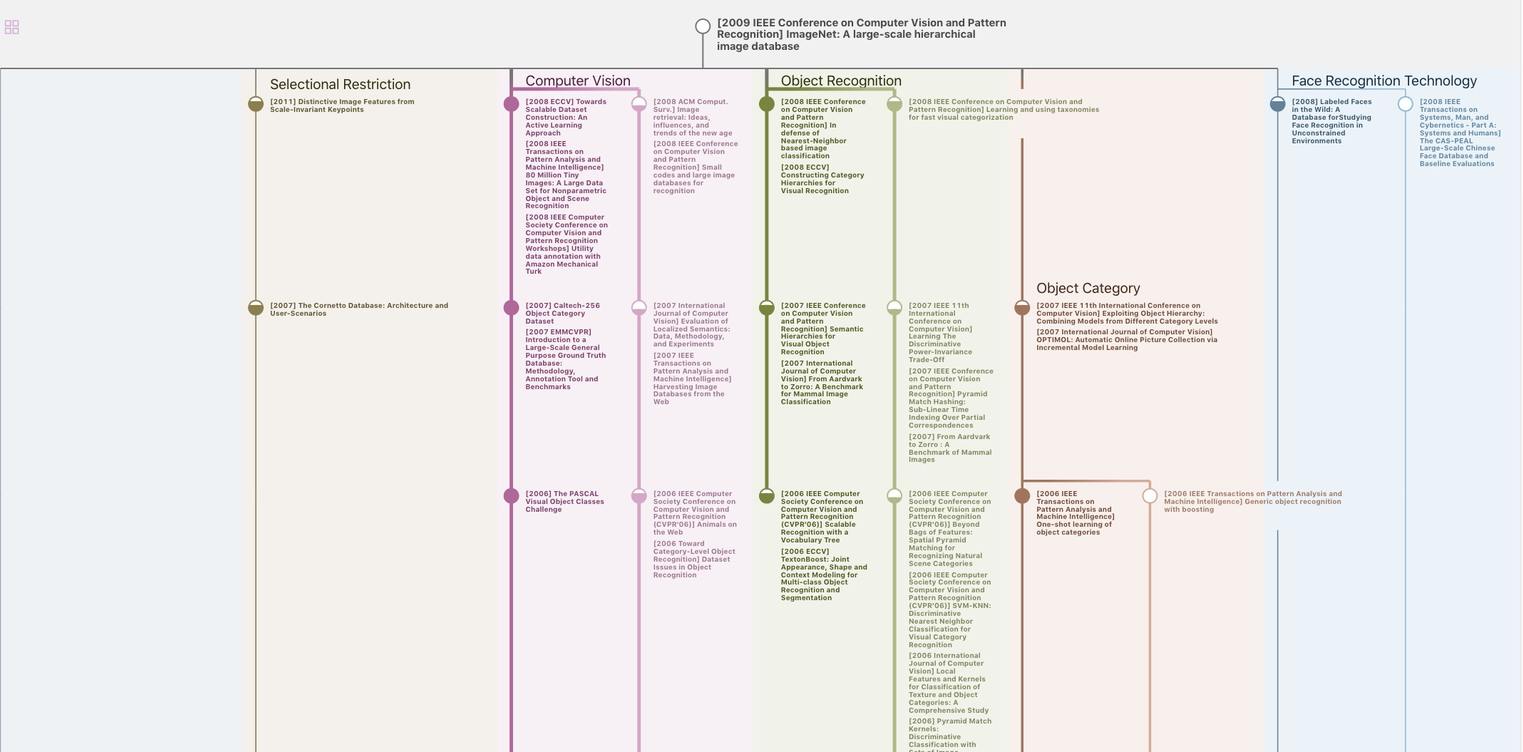
生成溯源树,研究论文发展脉络
Chat Paper
正在生成论文摘要