A Novel Framework For Face Recognition Using Robust Local Representation-Based Classification
INTERNATIONAL JOURNAL OF DISTRIBUTED SENSOR NETWORKS(2019)
摘要
Face recognition via representation-based classification is a trending technique in the recent years. However, the recognition performance of the systems using such a technique degrades in an unconstrained environment. In this article, a novel framework is proposed for representation-based face recognition. To deal with the unconstrained environment, a pre-process is used to frontalize face images, and aligned downsampling local binary pattern features of the frontalized images are used for classification. A dimension reduction is then adopted in order to reduce the computation complexity via an optimized projection matrix. The recognition is carried out using an improved robust sparse coding algorithm. Such an algorithm is expected to avoid the overfitting problem. The open-universe test on labeled faces in the wild data sets shows that the recognition rate of the proposed system can reach 95% with a recall rate of 80%, which is best among those representation-based classification face recognition systems.
更多查看译文
关键词
Face recognition, alignment downsampling local binary pattern, robust sparse coding, projection matrix optimization, nonnegative sparse representation
AI 理解论文
溯源树
样例
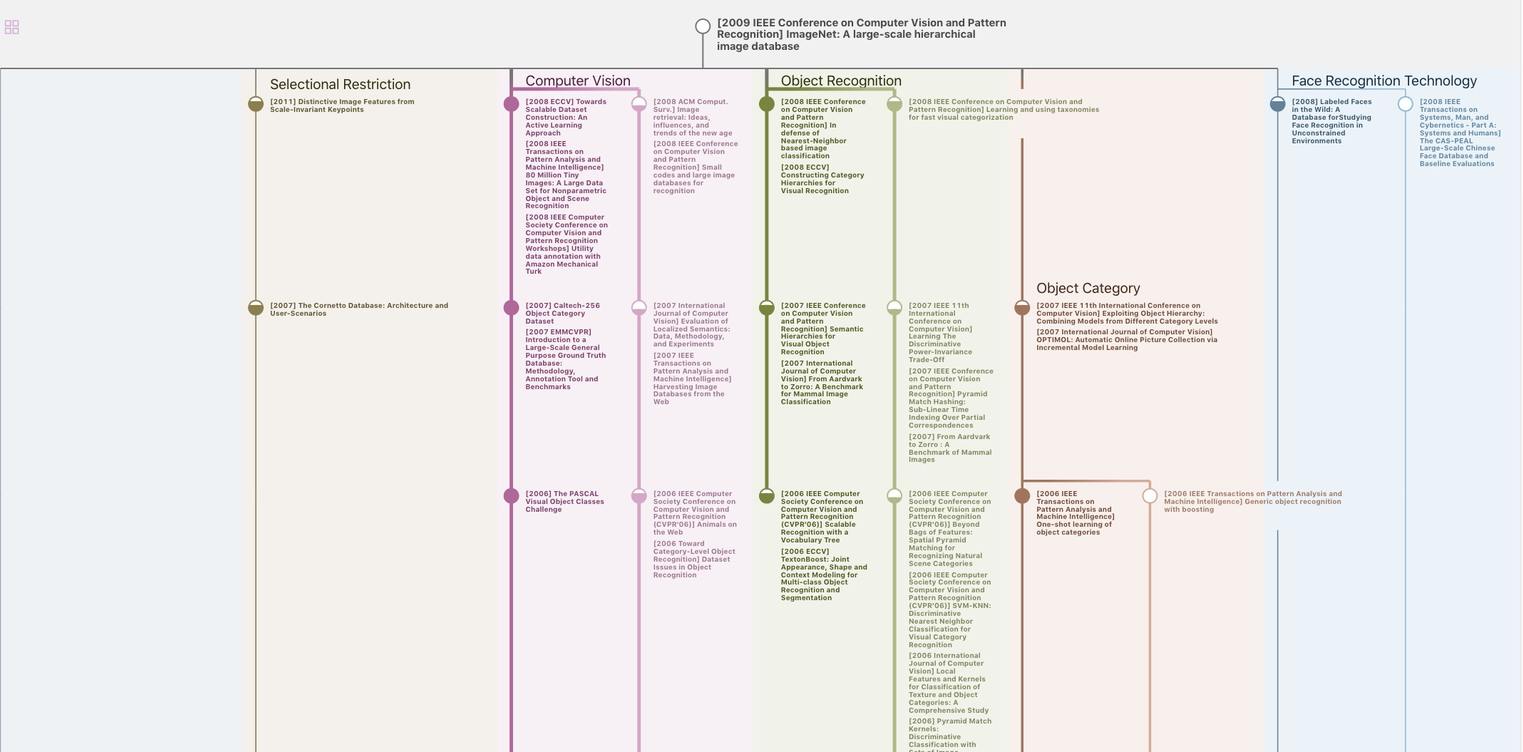
生成溯源树,研究论文发展脉络
Chat Paper
正在生成论文摘要