Personalized Neural Embeddings for Collaborative Filtering with Text
NAACL-HLT (1)(2019)
摘要
Collaborative filtering (CF) is a core technique for recommender systems. Traditional CF approaches exploit user-item relations (e.g., clicks, likes, and views) only and hence they suffer from the data sparsity issue. Items are usually associated with unstructured text such as article abstracts and product reviews. We develop a Personalized Neural Embedding (PNE) framework to exploit both interactions and words seamlessly. We learn such embeddings of users, items, and words jointly, and predict user preferences on items based on these learned representations. PNE estimates the probability that a user will like an item by two terms---behavior factors and semantic factors. On two real-world datasets, PNE shows better performance than four state-of-the-art baselines in terms of three metrics. We also show that PNE learns meaningful word embeddings by visualization.
更多查看译文
关键词
personalized neural embeddings,collaborative filtering
AI 理解论文
溯源树
样例
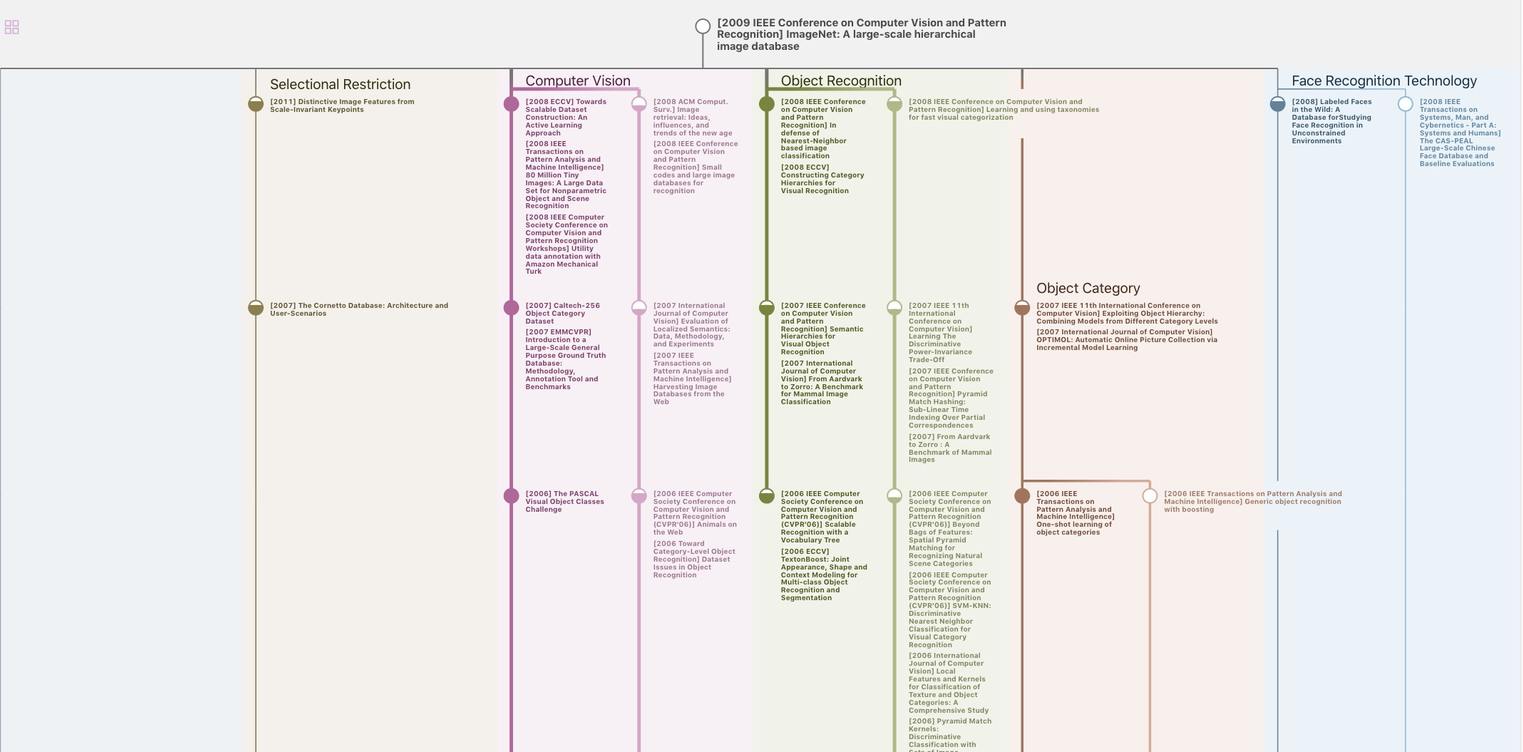
生成溯源树,研究论文发展脉络
Chat Paper
正在生成论文摘要