On Multi-Armed Bandit Designs For Dose-Finding Clinical Trials
JOURNAL OF MACHINE LEARNING RESEARCH(2021)
摘要
We study the problem of finding the optimal dosage in early stage clinical trials through the multi-armed bandit lens. We advocate the use of the Thompson Sampling principle, a flexible algorithm that can accommodate different types of monotonicity assumptions on the toxicity and efficacy of the doses. For the simplest version of Thompson Sampling, based on a uniform prior distribution for each dose, we provide finite-time upper bounds on the number of sub-optimal dose selections, which is unprecedented for dose-finding algorithms. Through a large simulation study, we then show that variants of Thompson Sampling based on more sophisticated prior distributions outperform state-of-the-art dose identification algorithms in different types of dose-finding studies that occur in phase I or phase I/II trials.
更多查看译文
关键词
Multi-Armed Bandits, Adaptive Clinical Trials, Phase I Clinical Trials, Phase I/II Clinical Trials, Thompson Sampling, Bayesian methods
AI 理解论文
溯源树
样例
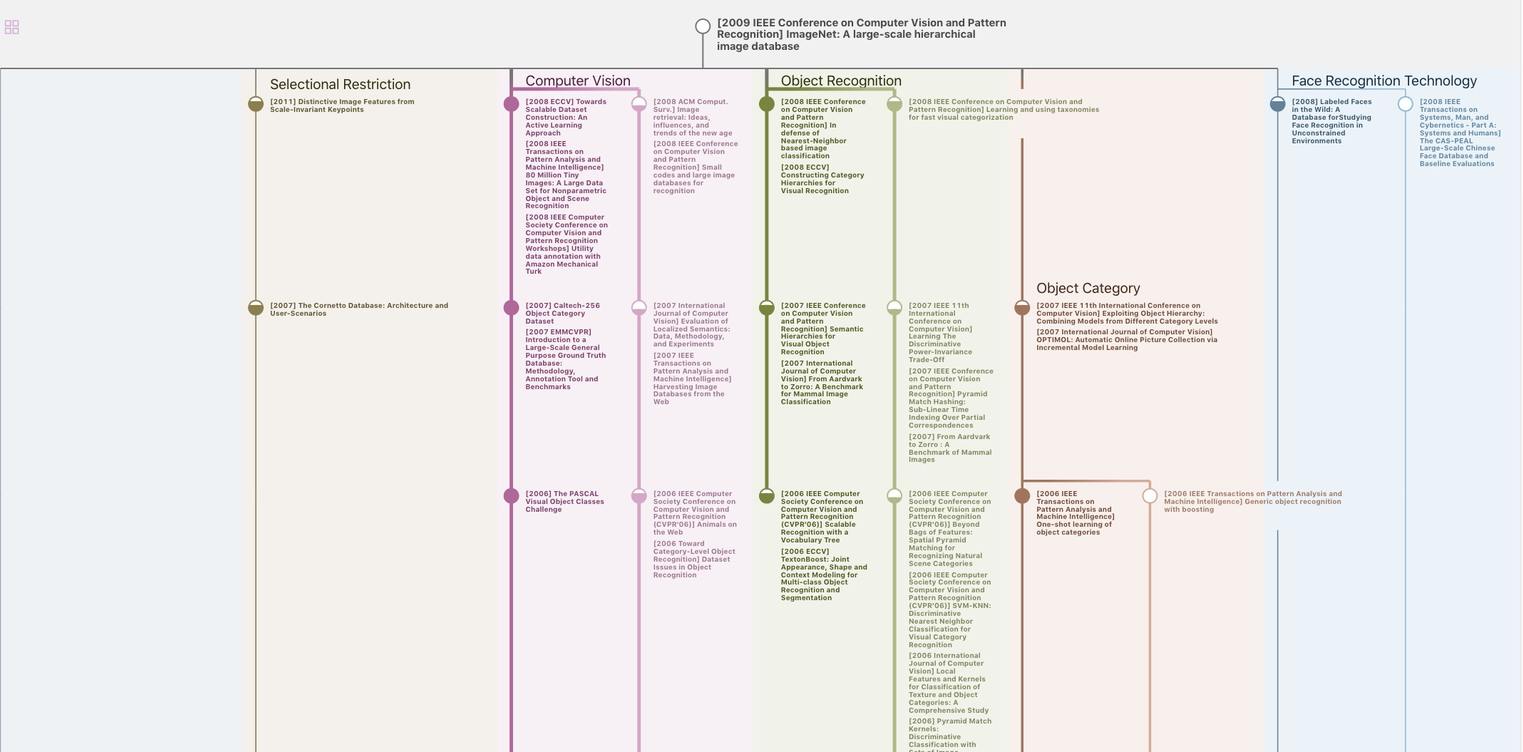
生成溯源树,研究论文发展脉络
Chat Paper
正在生成论文摘要