Adversarial Attacks on Deep Neural Networks for Time Series Classification
2019 INTERNATIONAL JOINT CONFERENCE ON NEURAL NETWORKS (IJCNN)(2019)
摘要
Time Series Classification (TSC) problems are encountered in many real life data mining tasks ranging from medicine and security to human activity recognition and food safety. With the recent success of deep neural networks in various domains such as computer vision and natural language processing, researchers started adopting these techniques for solving time series data mining problems. However, to the best of our knowledge, no previous work has considered the vulnerability of deep learning models to adversarial time series examples, which could potentially make them unreliable in situations where the decision taken by the classifier is crucial such as in medicine and security. For computer vision problems, such attacks have been shown to be very easy to perform by altering the image and adding an imperceptible amount of noise to trick the network into wrongly classifying the input image. Following this line of work, we propose to leverage existing adversarial attack mechanisms to add a special noise to the input time series in order to decrease the network's confidence when classifying instances at test time. Our results reveal that current state-of-the-art deep learning time series classifiers are vulnerable to adversarial attacks which can have major consequences in multiple domains such as food safety and quality assurance.
更多查看译文
关键词
food safety,quality assurance,adversarial attacks,deep neural networks,medicine,security,human activity recognition,natural language processing,deep learning models,computer vision problems,time series classification,data mining tasks
AI 理解论文
溯源树
样例
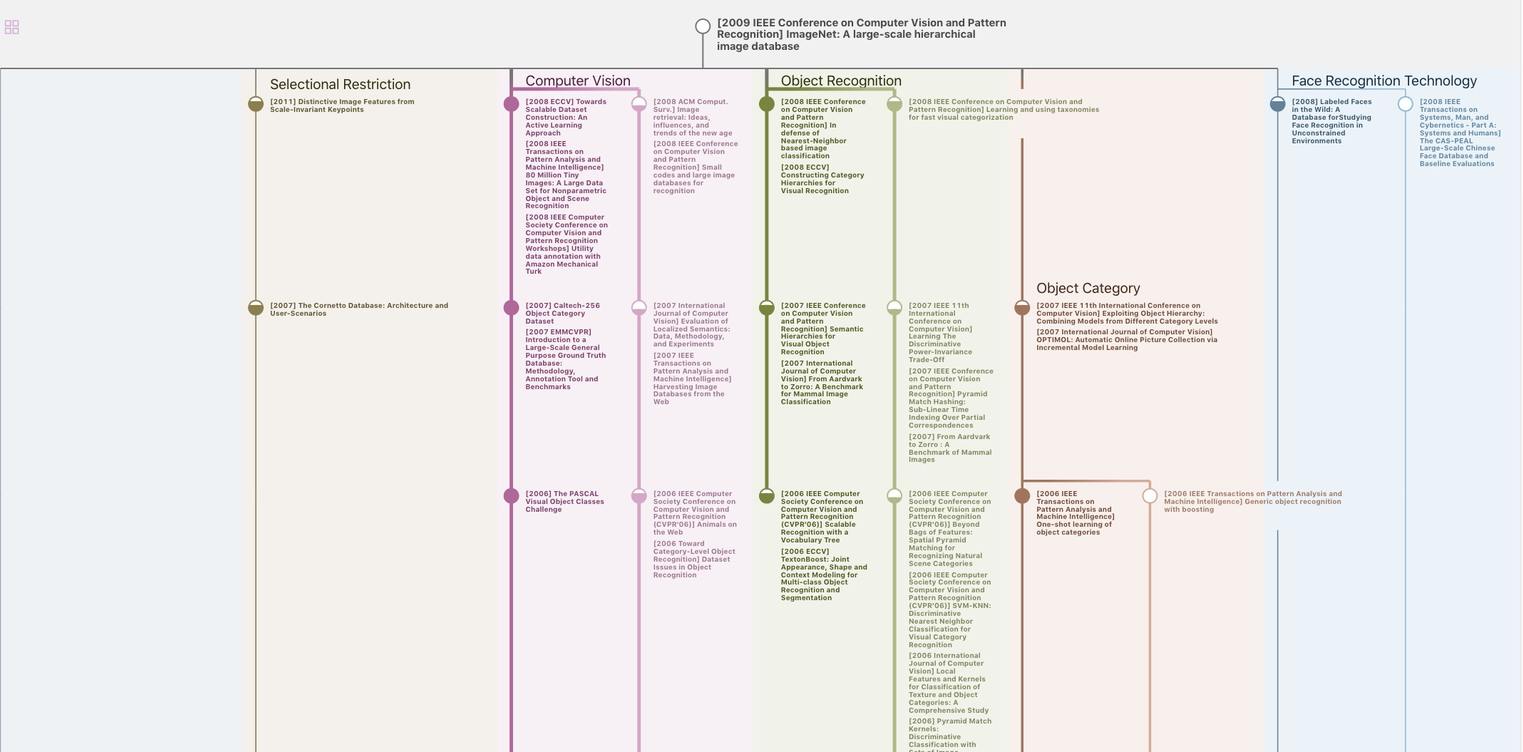
生成溯源树,研究论文发展脉络
Chat Paper
正在生成论文摘要