A Convolutional Neural Network approach for classification of dementia stages based on 2D-spectral representation of EEG recordings.
Neurocomputing(2019)
摘要
A data-driven machine deep learning approach is proposed for differentiating subjects with Alzheimer’s Disease (AD), Mild Cognitive Impairment (MCI) and Healthy Control (HC), by only analyzing noninvasive scalp EEG recordings. The methodology here proposed consists of evaluating the power spectral density (PSD) of the 19-channels EEG traces and representing the related spectral profiles into 2-d gray scale images (PSD-images). A customized Convolutional Neural Network with one processing module of convolution, Rectified Linear Units (ReLu) and pooling layer (CNN1) is designed to extract from PSD-images some suitable features and to perform the corresponding two and three-ways classification tasks. The resulting CNN is shown to provide better classification performance when compared to more conventional learning machines; indeed, it achieves an average accuracy of 89.8% in binary classification and of 83.3% in three-ways classification. These results encourage the use of deep processing systems (here, an engineered first stage, namely the PSD-image extraction, and a second or multiple CNN stage) in challenging clinical frameworks.
更多查看译文
关键词
Deep learning,Convolutional Neural Network,Power spectral density,Alzheimer’s disease,Mild Cognitive Impairment
AI 理解论文
溯源树
样例
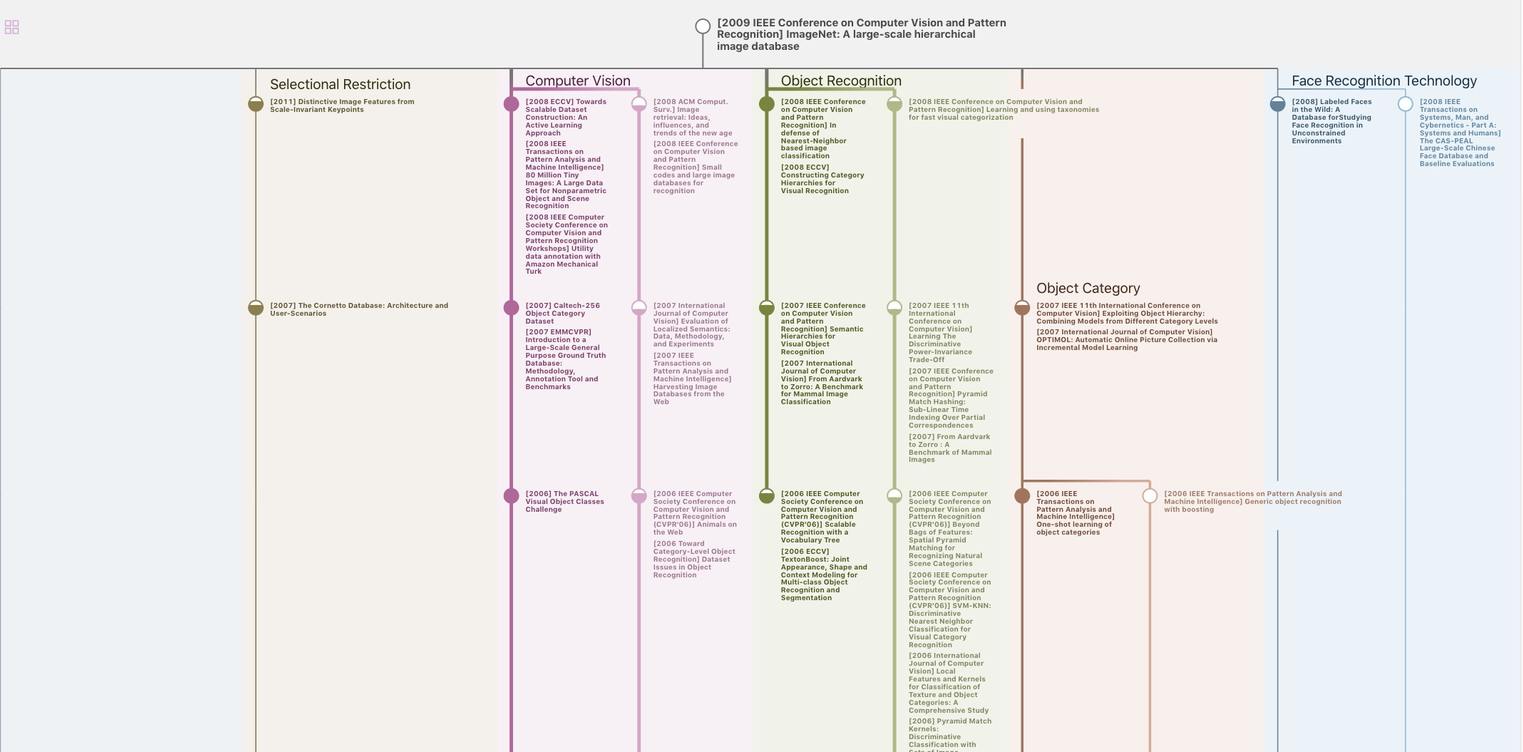
生成溯源树,研究论文发展脉络
Chat Paper
正在生成论文摘要