A Word Embeddings Training Method Based On Modified Skip-Gram And Align
CLOUD COMPUTING AND SECURITY, PT VI(2018)
摘要
To solve the problems that there is no sufficient annotated data in low-resource languages and it is hard to mine the deep semantic correspondence between languages via existing bilingual word embedding learning methods, this paper presents an effective text processing method based on transfer learning and bilingual word embedding model CWDR-BiGRU (Cross-context window of dynamic ratio bidirectional Gated Recurrent Unit) which contains an enhanced Skip-gram called cross-context window of dynamic ratio and encoder-decoder. The method can process low-resource language text effectively only using sentence-aligned corpus of bilingual resource languages and annotated data of high-resource language. The experimental results of semantic reasoning and word embedding visualization show that CWDR-BiGRU can effectively train bilingual word embeddings. In the task of Chinese-Tibetan cross-lingual document classification, the accuracy of transfer learning method based on CWDR-BiGRU is higher than the conventional method by 13.5%, and higher than the existing Bilingual Autoencoder, BilBOWA, BiCCV and BiSkip by 7.4%, 5.8%, 3.1% and 1.6% respectively, indicating CWDR-BiGRU which has reduced the difficulty of acquiring corpora for bilingual word embeddings can accurately excavate the deep alignment relationship and semantic properties.
更多查看译文
关键词
Bilingual word embeddings, Transfer learning, Low-resource language, Cross-context window of dynamic ratio
AI 理解论文
溯源树
样例
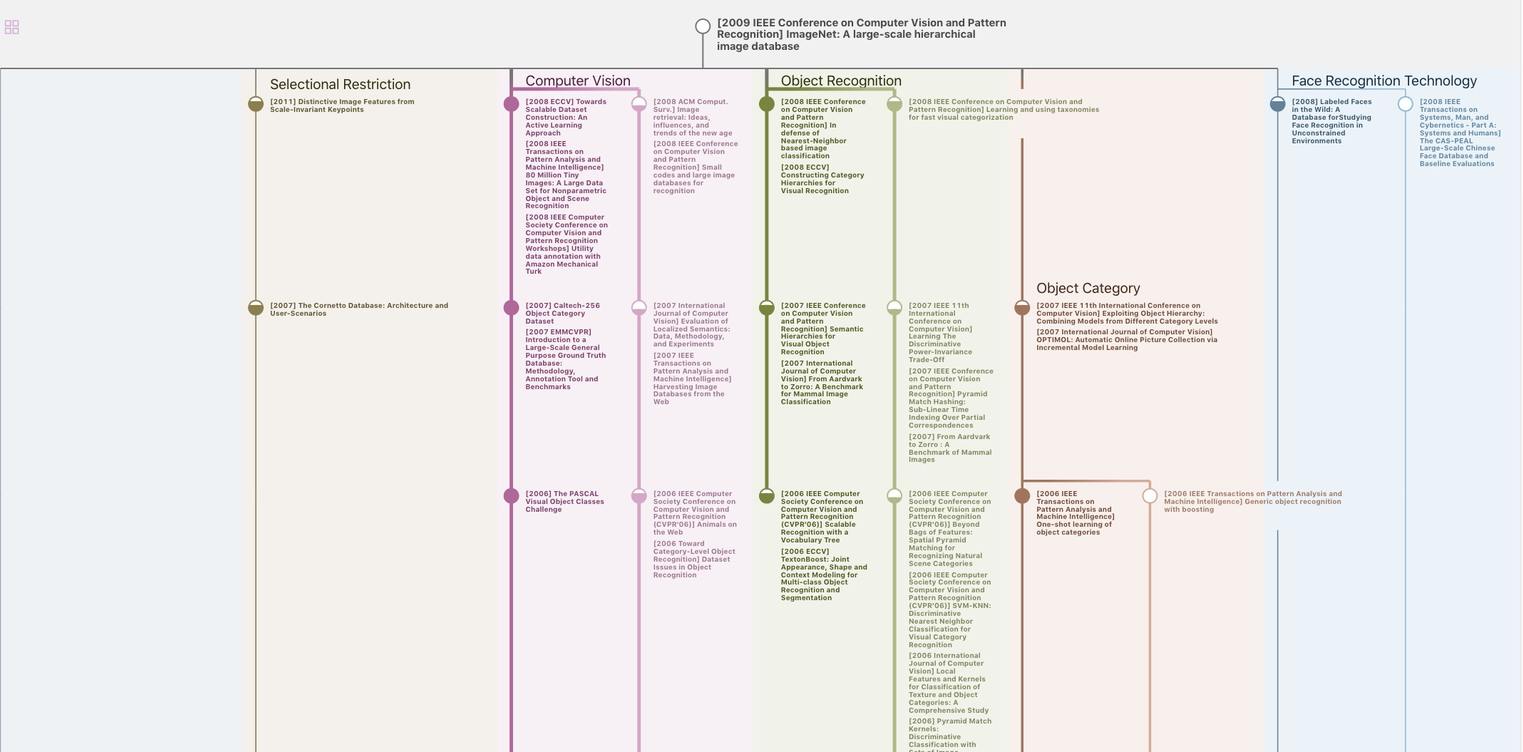
生成溯源树,研究论文发展脉络
Chat Paper
正在生成论文摘要