Combining auto-regression with exogenous variables in sequence-to-sequence recurrent neural networks for short-term load forecasting
2018 IEEE 16TH INTERNATIONAL CONFERENCE ON INDUSTRIAL INFORMATICS (INDIN)(2018)
摘要
In this paper we propose a sequence-to-sequence machine learning architecture for time-series forecasting based on recurrent neural networks. This architecture can be used as a general purpose forecasting method and is evaluated for the application of short-term electric load forecasting in this paper. The proposed sequence-to-sequence architecture
1
combines elements of auto-regressive forecasting techniques with multivariate regression by including exogenous variables for each forecasted time step as well as previous values when inferring forecasts. We assess the proposed architecture on a load data set provided by the Global Energy Forecasting Competition. The conclusion is that it outperforms other machine learning forecasting techniques as well as time-series analysis methods.
1
The implementation is available at: https://github.com/HenWil13/ieeeINDIN18
更多查看译文
关键词
Time-Series Forecasting, Load Forecasting, Regression, Auto-Regressive, Recurrent Neural Networks, STLF
AI 理解论文
溯源树
样例
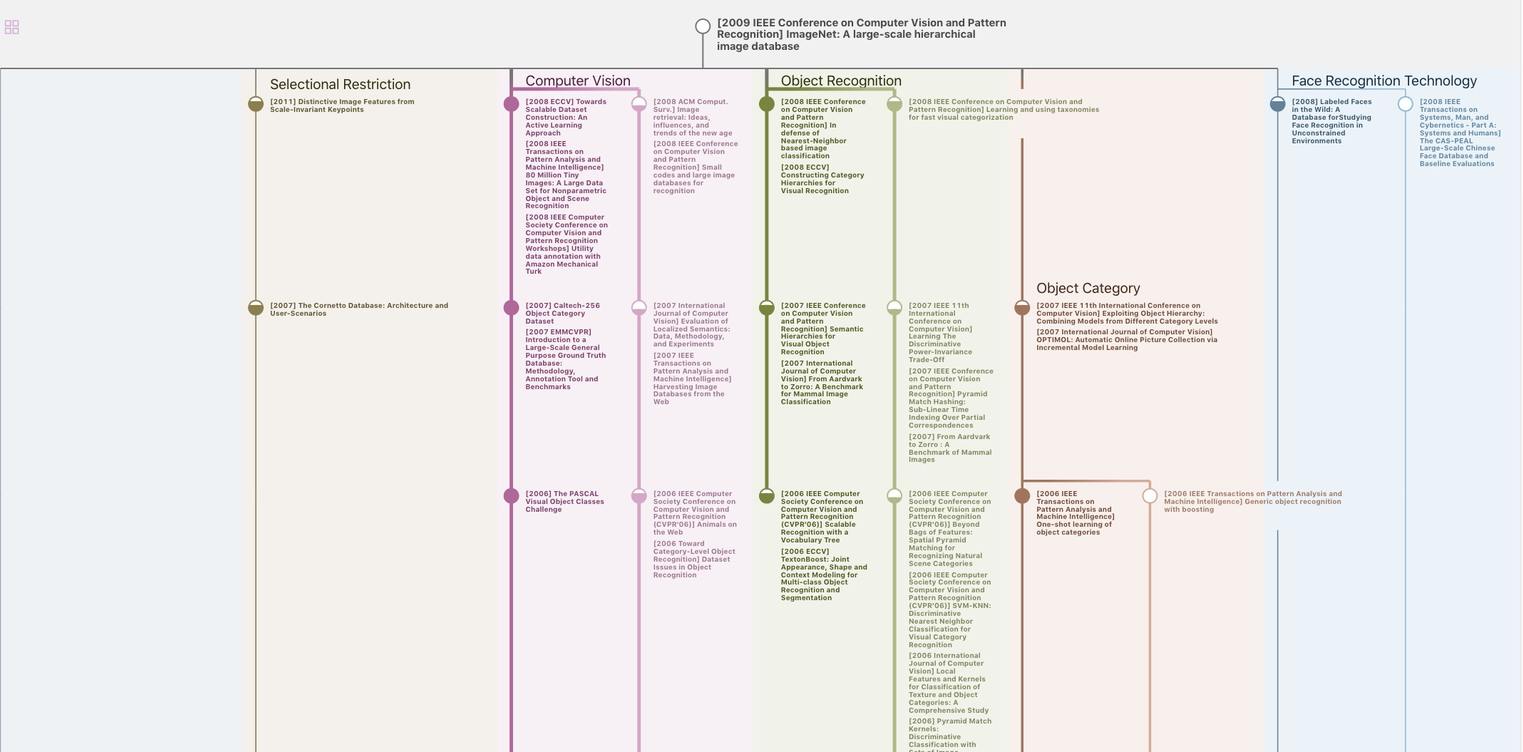
生成溯源树,研究论文发展脉络
Chat Paper
正在生成论文摘要