Community Exploration: From Offline Optimization to Online Learning.
ADVANCES IN NEURAL INFORMATION PROCESSING SYSTEMS 31 (NIPS 2018)(2018)
摘要
We introduce the community exploration problem that has many real-world applications such as online advertising. In the problem, an explorer allocates limited budget to explore communities so as to maximize the number of members he could meet. We provide a systematic study of the community exploration problem, from offline optimization to online learning. For the offline setting where the sizes of communities are known, we prove that the greedy methods for both of non-adaptive exploration and adaptive exploration are optimal. For the online setting where the sizes of communities are not known and need to be learned from the multi-round explorations, we propose an "upper confidence" like algorithm that achieves the logarithmic regret bounds. By combining the feedback from different rounds, we can achieve a constant regret bound.
更多查看译文
关键词
online learning,exploration problem,online advertising
AI 理解论文
溯源树
样例
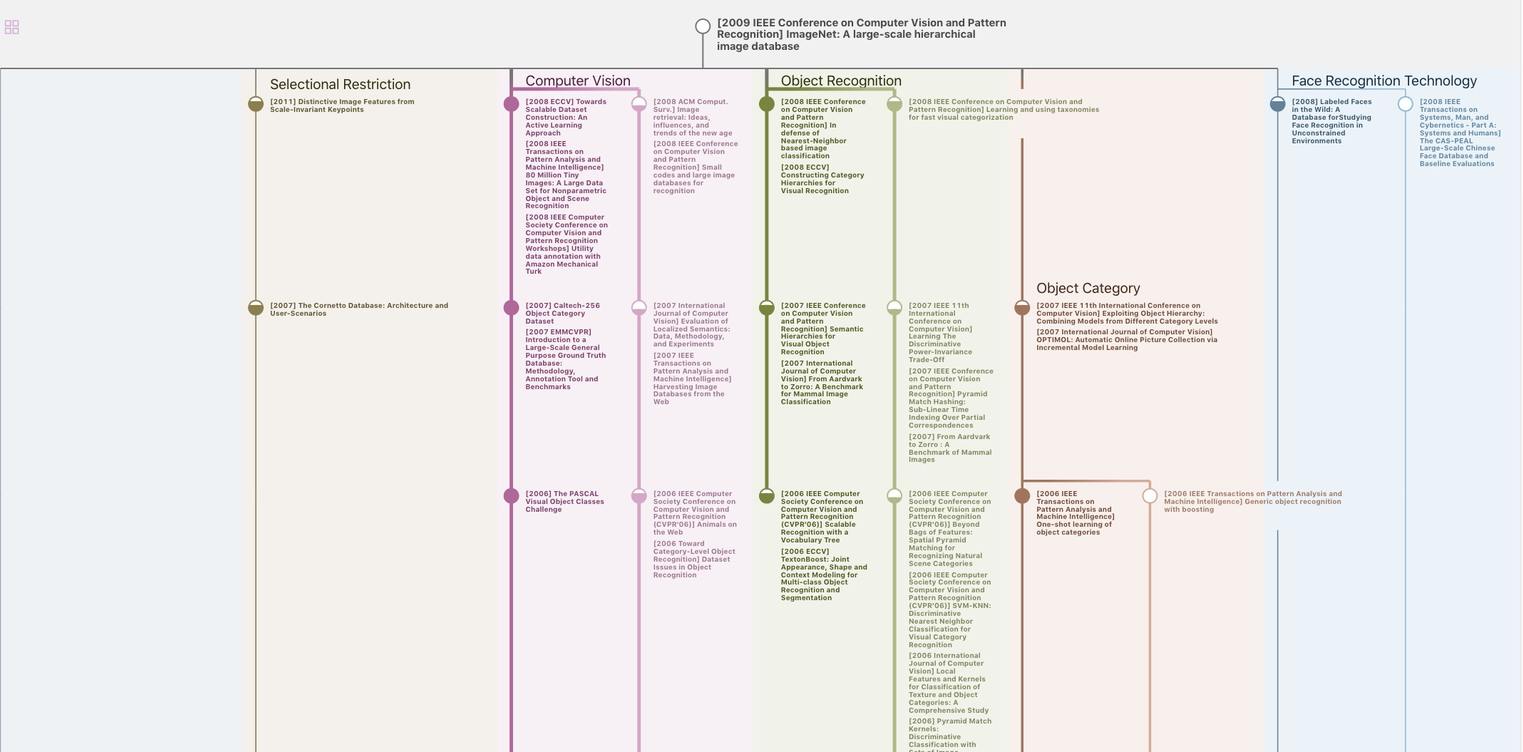
生成溯源树,研究论文发展脉络
Chat Paper
正在生成论文摘要