To Index or Not to Index: Optimizing Exact Maximum Inner Product Search
2019 IEEE 35th International Conference on Data Engineering (ICDE)(2019)
摘要
Exact Maximum Inner Product Search (MIPS) is an important task that is widely pertinent to recommender systems and high-dimensional similarity search. The brute-force approach to solving exact MIPS is computationally expensive, thus spurring recent development of novel indexes and pruning techniques for this task. In this paper, we show that a hardware-efficient brute-force approach, blocked matrix multiply (BMM), can outperform the state-of-the-art MIPS solvers by over an order of magnitude, for some—but not all—inputs. In this paper we also present a novel MIPS solution, MAX-IMUS, that takes advantage of hardware efficiency and pruning of the search space. Like BMM, MAXIMUS is faster than other solvers by up to an order of magnitude, but again only for some inputs. Since no single solution offers the best runtime performance for all inputs, we introduce a new data-dependent optimizer, OPTIMUS, that selects online with minimal overhead the best MIPS solver for a given input. Together, OPTIMUS and MAXIMUS outperform state-of-the-art MIPS solvers by 3.2× on average, and up to 10.9×, on widely studied MIPS datasets.
更多查看译文
关键词
Indexes,Runtime,Hardware,Computational modeling,Integrated circuits,Radio frequency,Linear algebra
AI 理解论文
溯源树
样例
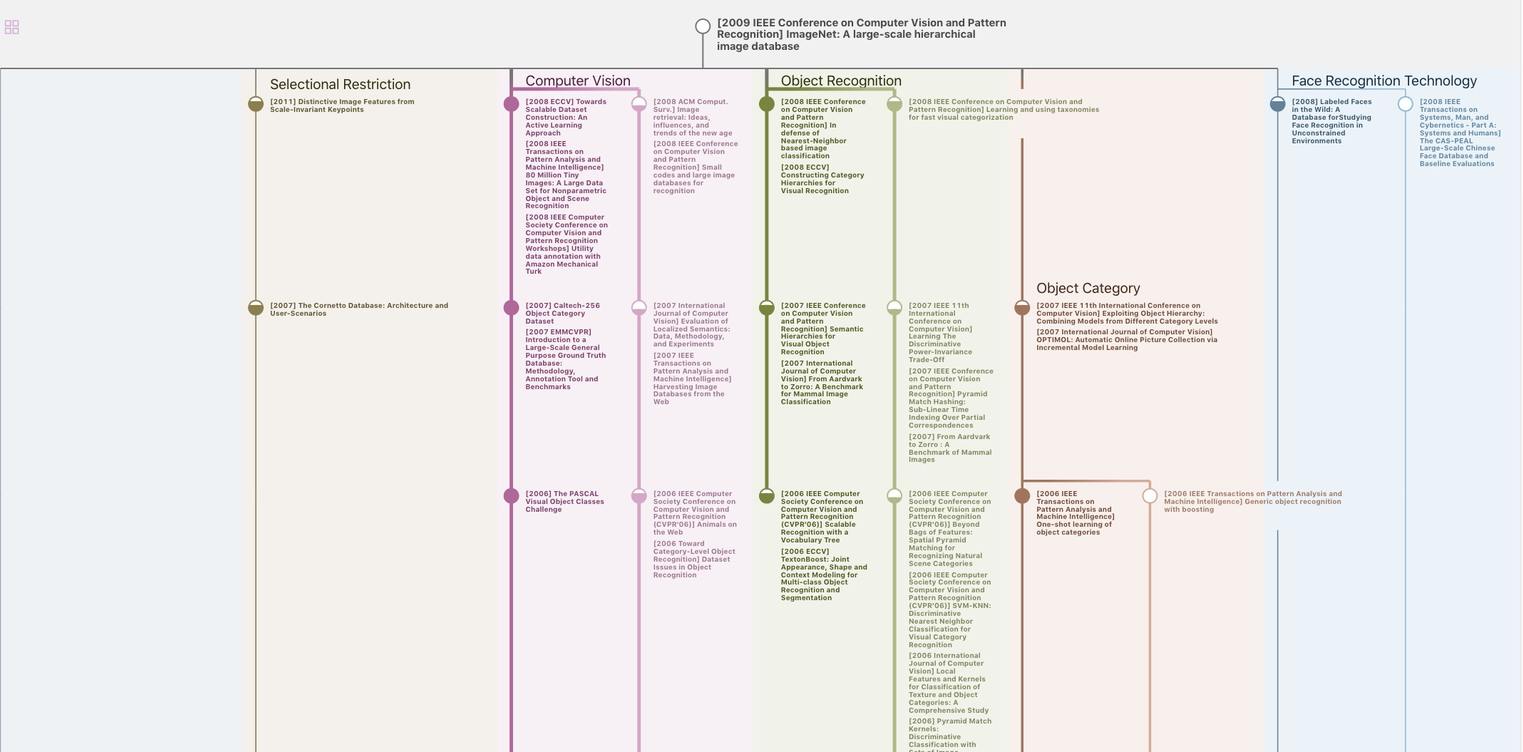
生成溯源树,研究论文发展脉络
Chat Paper
正在生成论文摘要