CID Models on Real-world Social Networks and Goodness of Fit Measurements.
arXiv: Methodology(2018)
摘要
Assessing the model fit quality of statistical models for network data is an ongoing and under-examined topic in statistical network analysis. Traditional metrics for evaluating model fit on tabular data such as the Bayesian Information Criterion are not suitable for models specialized for network data. We propose a novel self-developed goodness of fit (GOF) measure, the `stratified-sampling cross-validationu0027 (SCV) metric, that uses a procedure similar to traditional cross-validation via stratified-sampling to select dyads in the networku0027s adjacency matrix to be removed. SCV is capable of intuitively expressing different modelsu0027 ability to predict on missing dyads. Using SCV on real-world social networks, we identify the appropriate statistical models for different network structures and generalize such patterns. In particular, we focus on conditionally independent dyad (CID) models such as the Erdos Renyi model, the stochastic block model, the sender-receiver model, and the latent space model.
更多查看译文
关键词
cid models,fit measurements,networks,real-world
AI 理解论文
溯源树
样例
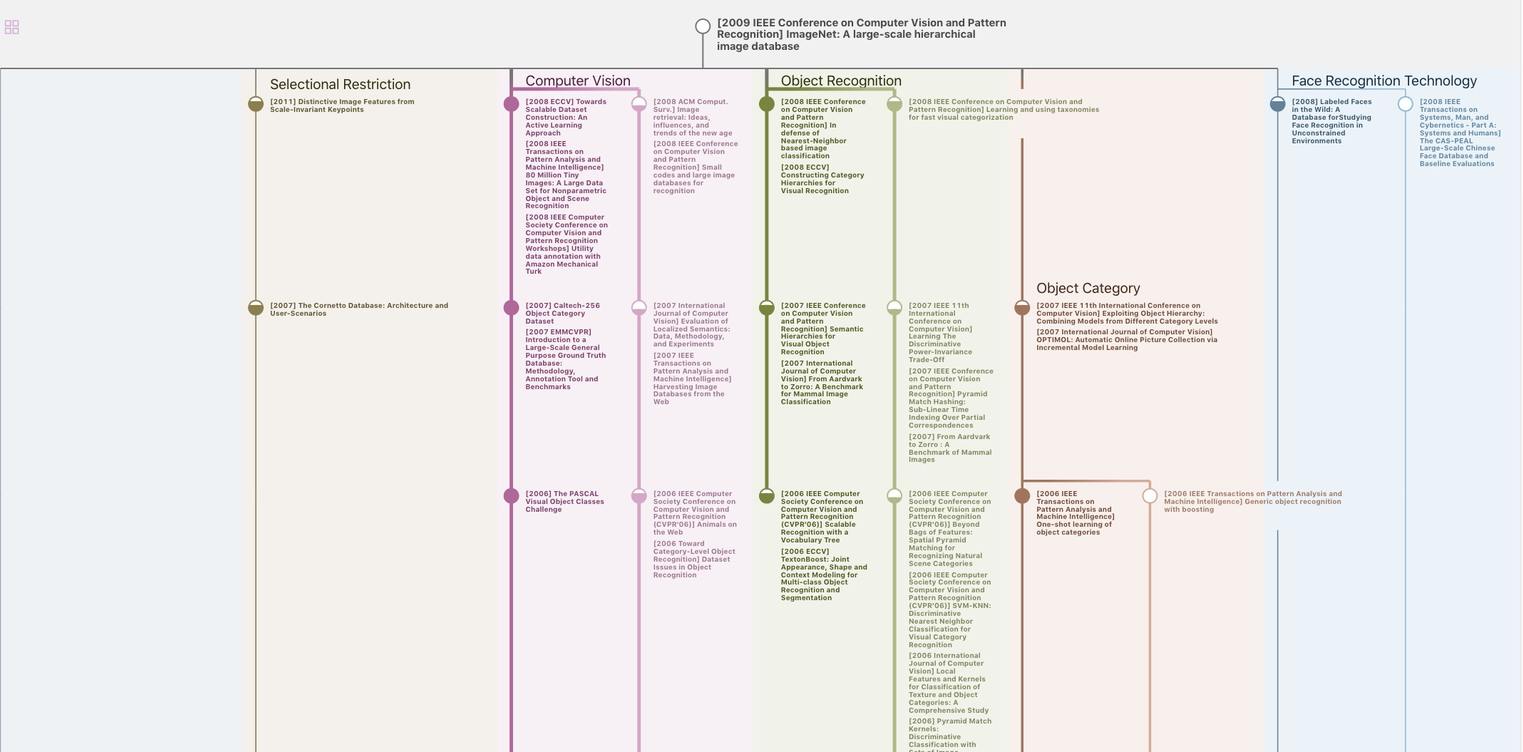
生成溯源树,研究论文发展脉络
Chat Paper
正在生成论文摘要