DL-GSA: A Deep Learning Metaheuristic Approach to Missing Data Imputation
ADVANCES IN SWARM INTELLIGENCE, ICSI 2018, PT II(2018)
摘要
Incomplete data has emerged as a prominent problem in the fields of machine learning, big data and various other academic studies. Due to the surge in deep learning techniques for problem-solving, in this paper, authors have proposed a deep learning-metaheuristic approach to combat the problem of imputing missing data. The proposed approach (DL-GSA) makes use of the nature inspired metaheuristic, Gravitational search algorithm, in combination with a deep-autoencoder and performs better than existing methods in terms of both accuracy and time. Owing to these improvements, DL-GSA has wider applications in both time and accuracy sensitive areas like imputation of scientific and research datasets, data analysis, machine learning and big data.
更多查看译文
关键词
Autoencoder,Missing at random,Missing data imputation,Gravitational search algorithm,Missing completely at random
AI 理解论文
溯源树
样例
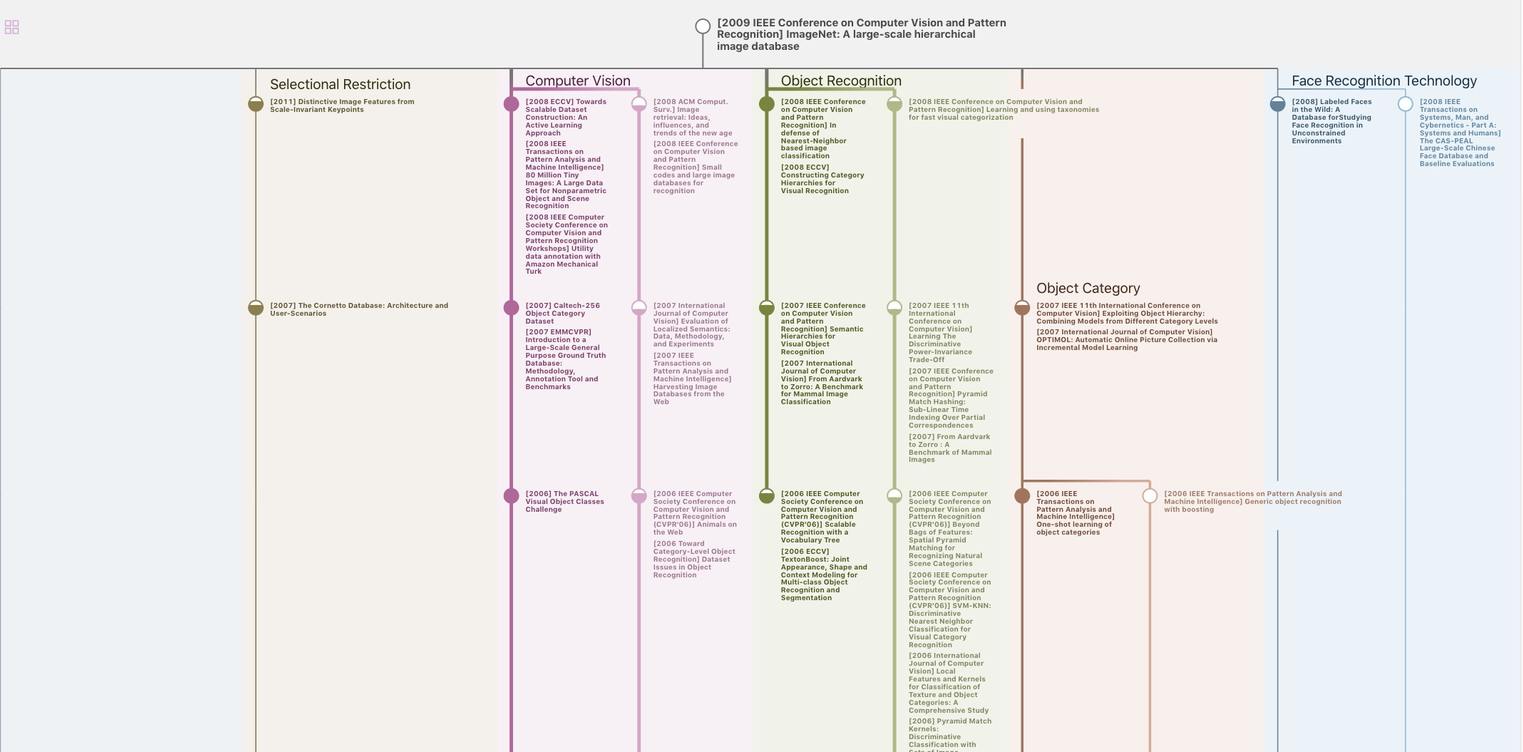
生成溯源树,研究论文发展脉络
Chat Paper
正在生成论文摘要