One-at-a-time: A Meta-Learning Recommender-System for Recommendation-Algorithm Selection on Micro Level.
arXiv: Information Retrieval(2018)
摘要
The effectiveness of recommendation algorithms is typically assessed with evaluation metrics such as root mean square error, F1, or click through rates, calculated over entire datasets. The best algorithm is typically chosen based on these overall metrics. However, there is no single-best algorithm for all users, items, and contexts. Choosing a single algorithm based on overall evaluation results is not optimal. In this paper, we propose a meta-learning-based approach to recommendation, which aims to select the best algorithm for each user-item pair. We evaluate our approach using the MovieLens 100K and 1M datasets. Our approach (RMSE, 100K: 0.973; 1M: 0.908) did not outperform the single-best algorithm, SVD++ (RMSE, 100K: 0.942; 1M: 0.887). We also develop a distinction between meta-learners that operate per-instance (micro-level), per-data subset (mid-level), and per-dataset (global level). Our evaluation shows that a hypothetically perfect micro-level meta-learner would improve RMSE by 25.5% for the MovieLens 100K and 1M datasets, compared to the overall-best algorithms used.
更多查看译文
AI 理解论文
溯源树
样例
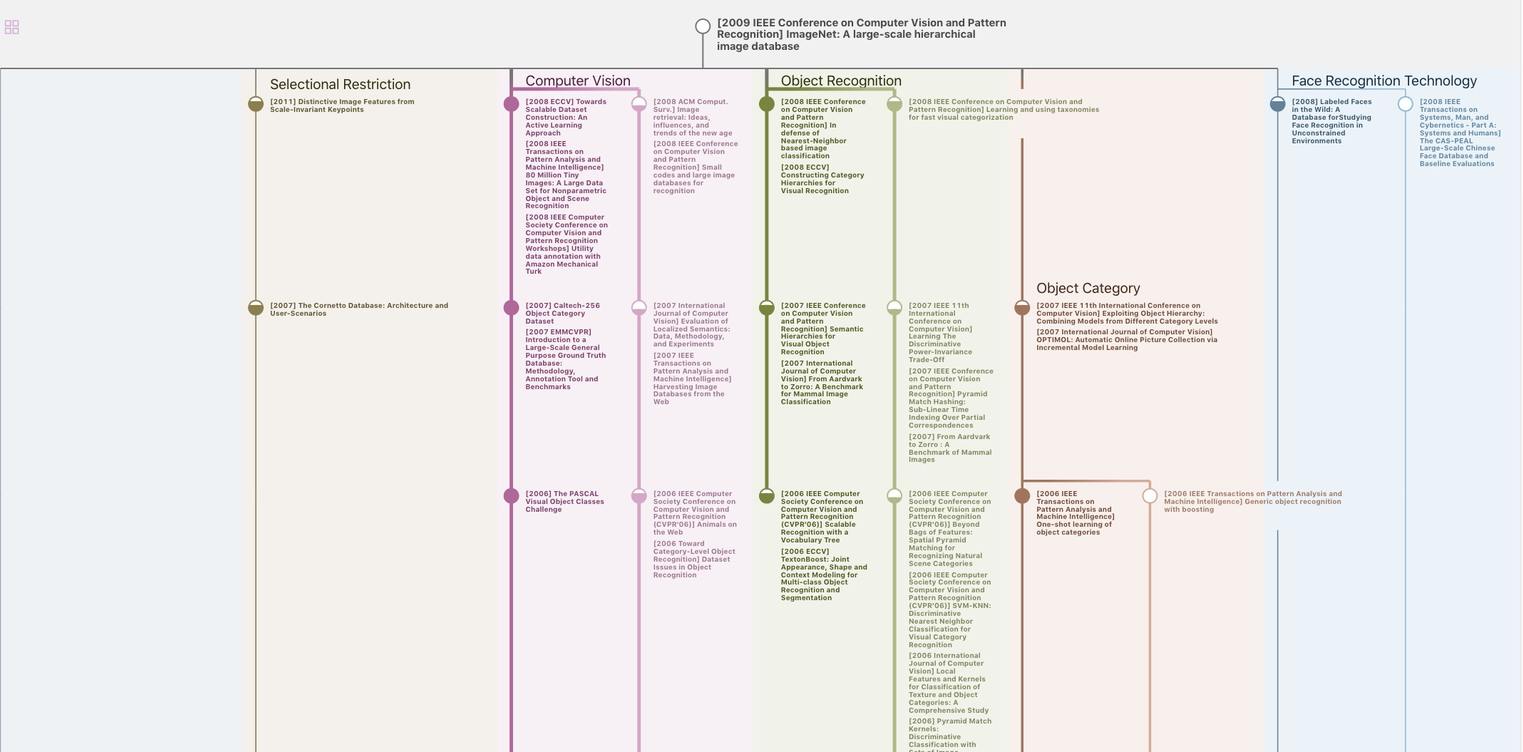
生成溯源树,研究论文发展脉络
Chat Paper
正在生成论文摘要