Multi-level Semantic Representation for Flower Classification.
ADVANCES IN MULTIMEDIA INFORMATION PROCESSING - PCM 2017, PT I(2017)
摘要
Fine-grained classification is challenging since sub-categories have little intra-class variances and large intra-class variations. The task of flower classification can be achieved through highlighting the discriminative parts. Most traditional methods trained Convolutional Neural Networks (CNN) to handle the variations of pose, color and rotation, which only utilize single-level semantic information. In this paper, we propose a fine-grained classification approach with multi-level semantic representation. With the complementary strengths of multi-level semantic representation, we attempt to capture the subtle differences between sub-categories. One object-level model and multiple part-level model are trained as a multi-scale classifier. We test our method on the Oxford Flower dataset with 102 categories, and our result achieves the best performance over other state-of-the-art approaches.
更多查看译文
关键词
Fine-grained classification,CNN,Multi-level representation
AI 理解论文
溯源树
样例
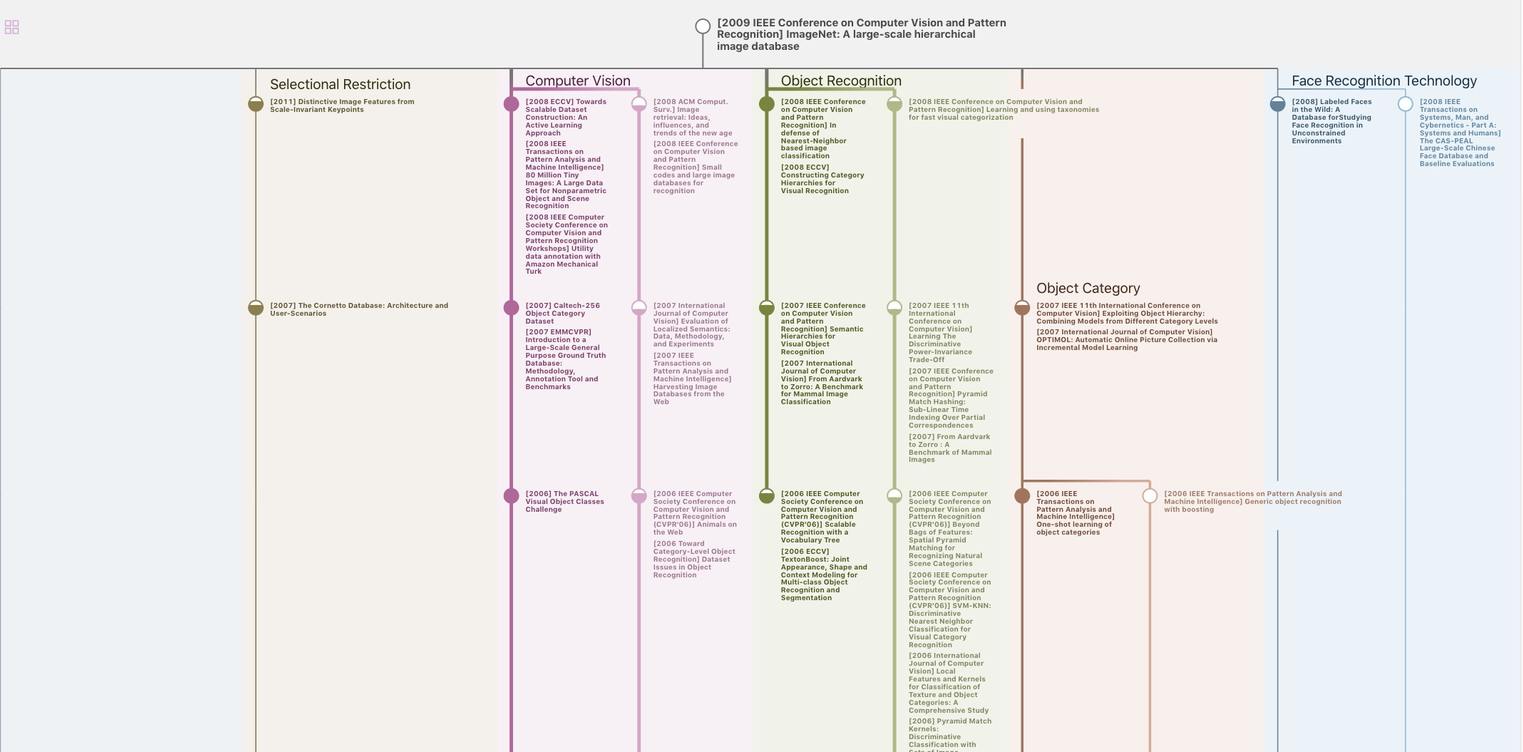
生成溯源树,研究论文发展脉络
Chat Paper
正在生成论文摘要