Parallel Direct Solution of the Covariance-Localized Ensemble Square Root Kalman Filter Equations with Matrix Functions
MONTHLY WEATHER REVIEW(2018)
摘要
Recently, the serial approach to solving the square root ensemble Kalman filter (ESRF) equations in the presence of covariance localization was found to depend on the order of observations. As shown previously, correctly updating the localized posterior covariance in serial requires additional effort and computational expense. A recent work by Steward et al. details an all-at-once direct method to solve the ESRF equations in parallel. This method uses the eigenvectors and eigenvalues of the forward observation covariance matrix to solve the difficult portion of the ESRF equations. The remaining assimilation is easily parallelized, and the analysis does not depend on the order of observations. While this allows for long localization lengths that would render local analysis methods inefficient, in theory, an eigenpair-based method scales as the cube number of observations, making it infeasible for large numbers of observations. In this work, we extend this method to use the theory of matrix functions to avoid eigenpair computations. The Arnoldi process is used to evaluate the covariance-localized ESRF equations on the reduced-order Krylov subspace basis. This method is shown to converge quickly and apparently regains a linear scaling with the number of observations. The method scales similarly to the widely used serial approach of Anderson and Collins in wall time but not in memory usage. To improve the memory usage issue, this method potentially can be used without an explicit matrix. In addition, hybrid ensemble and climatological covariances can be incorporated.
更多查看译文
关键词
Tropical cyclones,Data processing,Numerical analysis,modeling,Ensembles,Numerical weather prediction,forecasting,Data assimilation
AI 理解论文
溯源树
样例
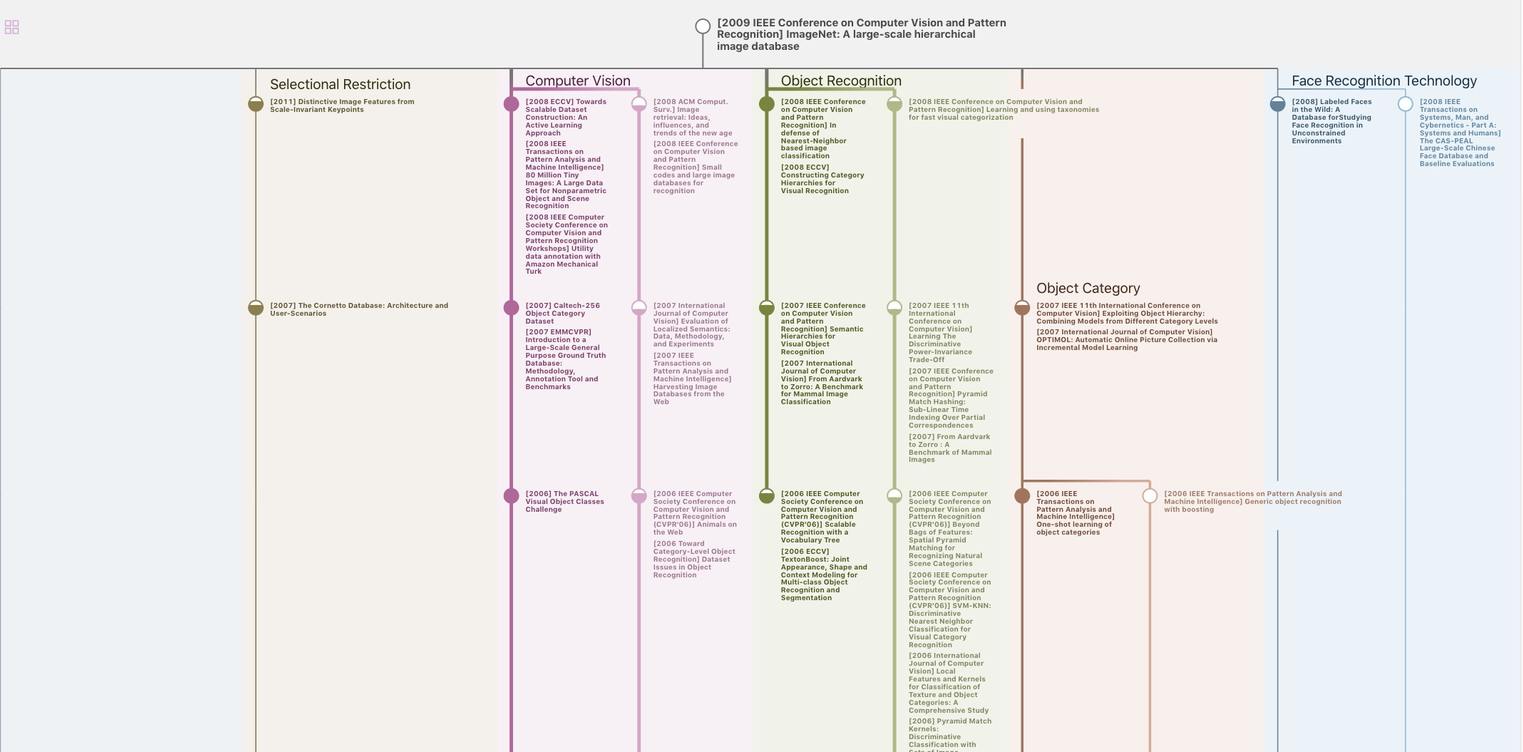
生成溯源树,研究论文发展脉络
Chat Paper
正在生成论文摘要