Discovering Progression Stages in Trillion-Scale Behavior Logs.
WWW '18: The Web Conference 2018 Lyon France April, 2018(2018)
摘要
User engagement is a key factor for the success of web services. Studying the following questions will help establishing business strategies leading to their success: How do the behaviors of users in a web service evolve over time? To reach a certain engagement level, what are the common stages that many users go through? How can we represent the stage that each individual user lies in? To answer these questions, we propose a behavior model that discovers the progressions of users' behaviors from a given starting point - such as a new subscription or first experience of certain features - to a particular target stage such as a predefined engagement level of interest. Under our model, transitions over stages represent progression of users where each stage in our model is characterized by probability distributions over types of actions, frequencies of actions, and next stages to move. Each user performs actions and moves to a next stage following the probability distributions characterizing the current stage. We also develop a fast and memory-efficient algorithm that fits our model to trillions of behavioral logs. Our algorithm scales linearly with the size of data. Especially, its distributed version implemented in the MapReduce framework successfully handles petabyte-scale data with one trillion actions. Lastly, we show the effectiveness of our model and algorithm by applying them to real-world data from LinkedIn. We discover meaningful stages that LinkedIn users go through leading to predefined target goals. In addition, our trained models are shown to be useful for downstream tasks such as prediction of future actions.
更多查看译文
关键词
progression stages,behavior,trillion-scale
AI 理解论文
溯源树
样例
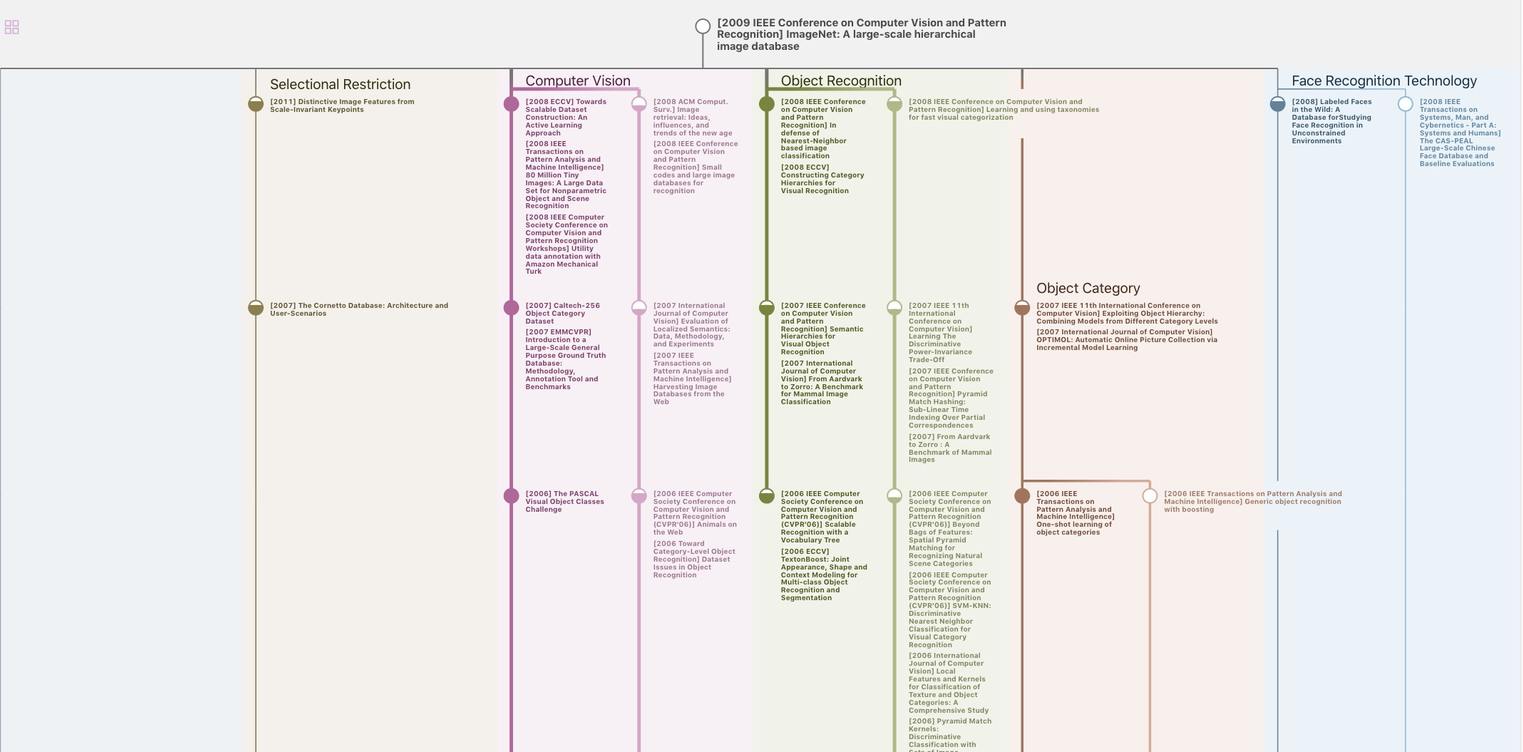
生成溯源树,研究论文发展脉络
Chat Paper
正在生成论文摘要