A Large-Scale Attribute Dataset for Zero-Shot Learning
2019 IEEE/CVF Conference on Computer Vision and Pattern Recognition Workshops (CVPRW)(2019)
摘要
Zero-Shot Learning (ZSL) has attracted huge research attention over the past few years; it aims to learn the new concepts that have never been seen before. Each concept (class) is embedded in two or more modalities, e.g., the image features and semantic embeddings. Attributes are introduced as the intermediate semantic representation to realize the knowledge transfer from seen to unseen classes. Previous ZSL algorithms are tested on several benchmark datasets, which are defective in terms of the image distribution and attribute diversity. In addition, we argue that the "co-occurrence bias problem" of existing datasets, which is caused by the biased co-occurrence of objects, significantly hinders models from correctly learning the concept. To overcome these problems, we propose a Large-scale Attribute Dataset (LAD) with 78,017 images of 230 classes. 359 attributes of visual, semantic and subjective properties are defined and annotated in instance-level. Seven state-of-the-art ZSL algorithms are tested on this new dataset. The experimental results reveal the challenge of implementing ZSL on our dataset. Based on the proposed dataset, Zero-shot Learning Competition of AI Challenger (>110 teams attended) has been organized for promoting ZSL research.
更多查看译文
关键词
large-scale attribute dataset,zero-shot learning competition,ZSL research,ZSL algorithms,subjective properties,semantic properties,visual properties,co-occurrence bias problem,attribute diversity,image distribution,benchmark datasets,intermediate semantic representation,semantic embeddings,image features
AI 理解论文
溯源树
样例
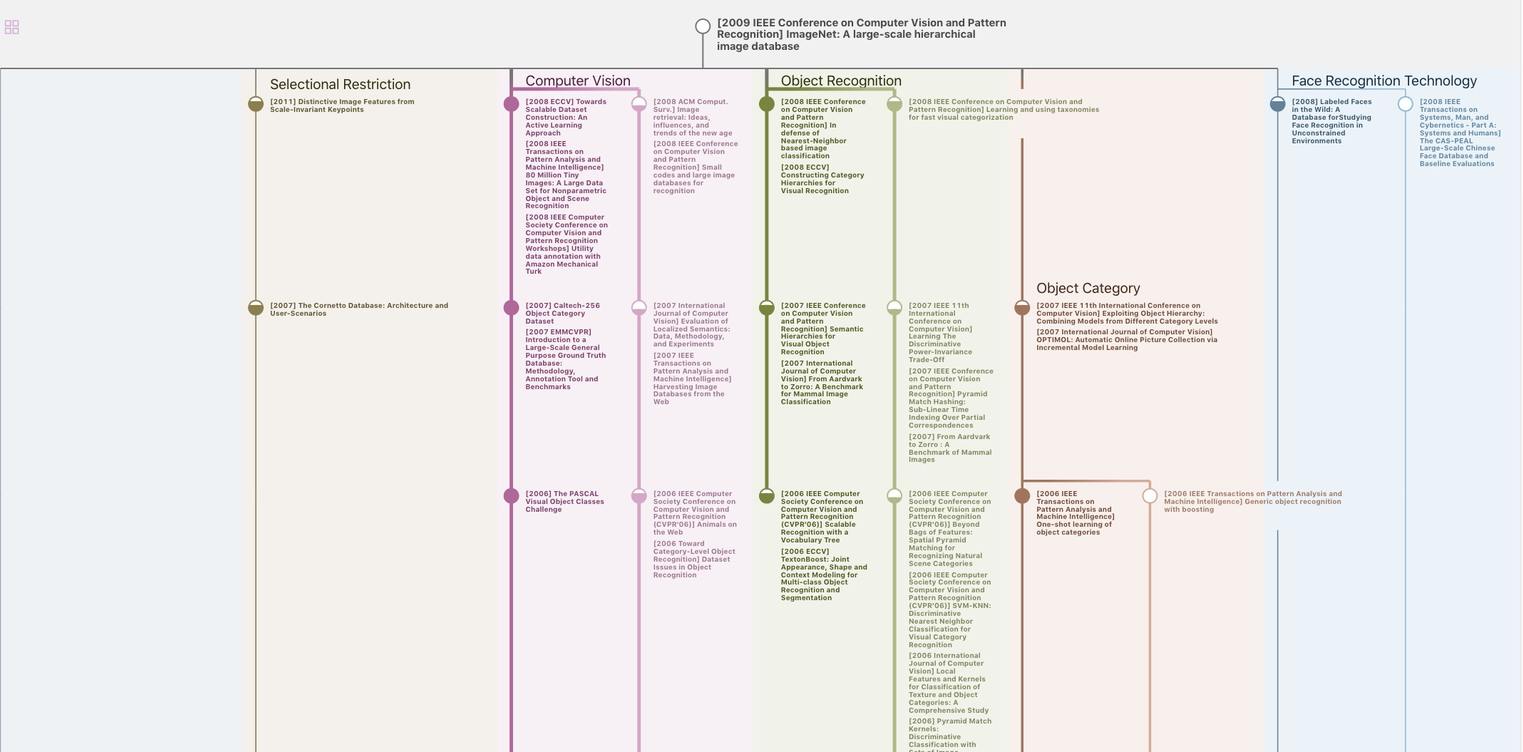
生成溯源树,研究论文发展脉络
Chat Paper
正在生成论文摘要