Bp Neural Network Based Bearing Fault Diagnosis With Differential Evolution & Eemd Denoise
2017 9TH INTERNATIONAL CONFERENCE ON MODELLING, IDENTIFICATION AND CONTROL (ICMIC 2017)(2017)
摘要
In mechanical equipment, rolling bearing is frequently used. Its running state directly affects the performance of the whole machine and it is also the main cause of mechanical equipment failure. This paper focuses on the fault diagnosis of the rolling bearings. The method of fault diagnosis based on the self-adaptive denoise of ensemble empirical mode decomposition (EEMD) and improved back propagation (BP) neural network with differential evolution (DE) algorithm is studied. Firstly, the signal is decomposed by EEMD, and then the reconstructed signal of adaptive noise reduction is acquired based on the threshold of distance measurement. Secondly, utilizing the error of BP neural network as the objective function, weights and thresholds of the network are optimized by DE algorithm. Finally, the optimized BP network is used for fault diagnosis. Experimental results show that the proposed method is more effective and accurate than the traditional BP neural network.
更多查看译文
关键词
BP Neural Network,Differential Evolution Algorithm,EEMD,Bearing Fault Diagnosis
AI 理解论文
溯源树
样例
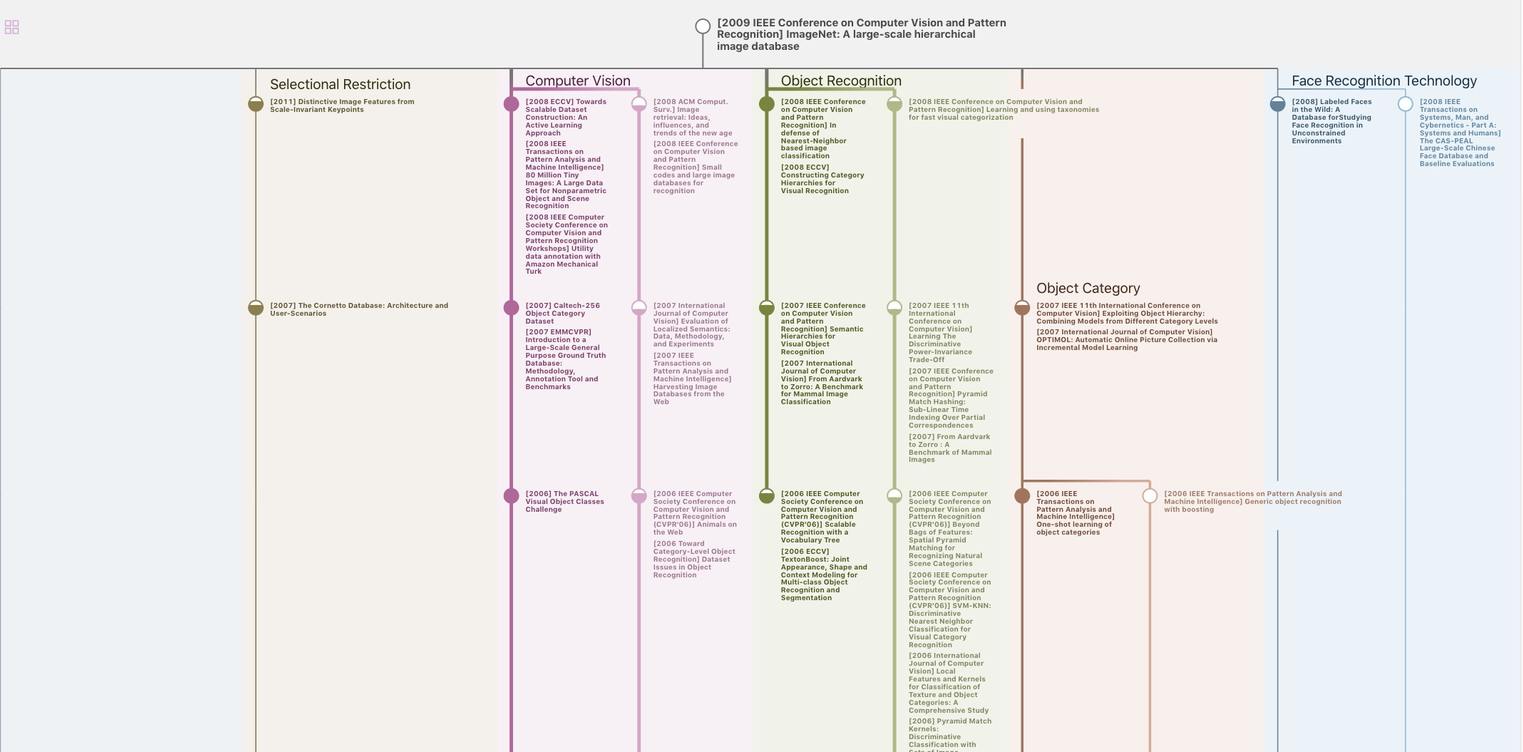
生成溯源树,研究论文发展脉络
Chat Paper
正在生成论文摘要