Recovering Nominal Tracking Performance in an Asymptotic Sense for Uncertain Linear Systems.
SIAM JOURNAL ON CONTROL AND OPTIMIZATION(2018)
摘要
In this paper, we consider the problem of recovering a (predefined) nominal output trajectory in the presence of model uncertainty and external disturbance. In particular, whereas the nominal performance recovery (NPR) has been studied in an approximate fashion in the literature, we extend the notion of the NPR in an asymptotic sense from the perspective of the internal model principle: that is, as long as the disturbance and reference signals are generated by an exogenous system, the actual output not only is kept close to the nominal trajectory as much as desired but also asymptotically converges to the nominal one as time elapses. It is shown via the singular perturbation theory that the asymptotic NPR can be achieved for uncertain minimum-phase systems under arbitrarily large (but bounded) model uncertainty. A disturbance observer (DOB) approach is employed in the controller design, with the internal model embedded into the so-called Q-filter, which is a key component of the DOB. Simulation results for mechanical positioning systems illustrate that the asymptotic NPR can enhance robust performance of control systems.
更多查看译文
关键词
nominal performance recovery,internal model principle,disturbance observer,robust control
AI 理解论文
溯源树
样例
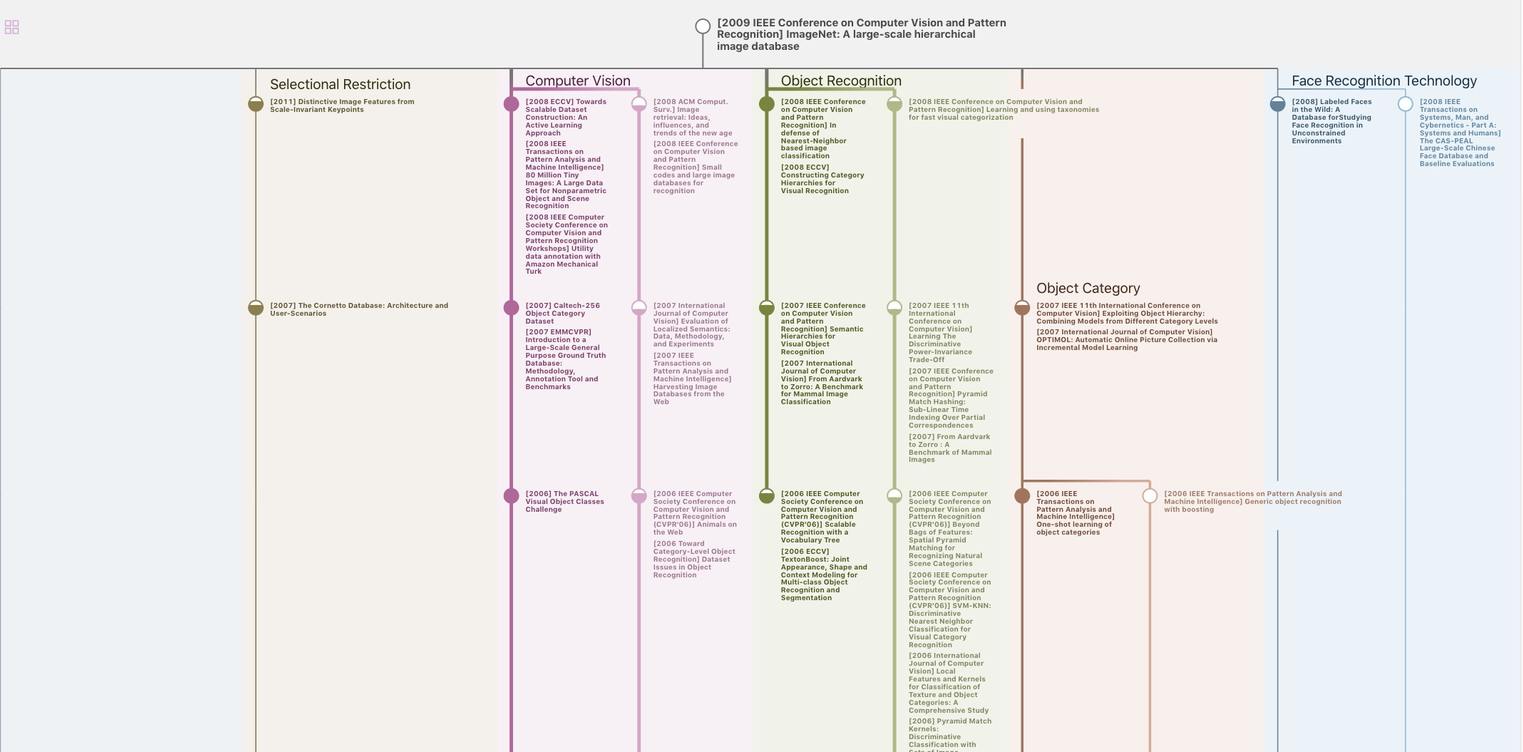
生成溯源树,研究论文发展脉络
Chat Paper
正在生成论文摘要