Leveraging Tree Statistics for Extracting Anatomical Trees from 3D Medical Images
2017 14th Conference on Computer and Robot Vision (CRV)(2017)
摘要
Using different priors (e.g. shape and appearance) have proven critical for robust image segmentation of different types of target objects. Many existing methods for extracting trees (e.g. vascular or airway trees) from medical images have leveraged appearance priors (e.g. tubular-ness and bifurcationness) and the knowledge of the cross-sectional geometry (e.g. circles or ellipses) of the tree-forming tubes. In this work, we present the first method for 3D tree extraction from 3D medical images (e.g. CT or MRI) that, in addition to appearance and cross-sectional geometry priors, utilizes prior tree statistics collected from the training data. Our tree extraction method collects and leverages topological tree prior and geometrical statistics, including tree hierarchy, branch angle and length statistics. Our implementation takes the form of a Bayesian tree centerline tracking method combining the aforementioned tree priors with observed image data. We evaluated our method on both synthetic 3D datasets and real clinical CT chest datasets. For synthetic data, our method's key feature of incorporating tree priors resulted in at least 13% increase in correctly detected branches under different noise levels. For real clinical scans, the mean distance from ground truth centerlines to the detected centerlines by our method was improved by 12% when utilizing tree priors. Both experiments validate that, by incorporating tree statistics, our tree extraction method becomes more robust to noise and provides more accurate branch localization.
更多查看译文
关键词
segmentation,machine learning,statistical analysis,tree structure,centerline tracking
AI 理解论文
溯源树
样例
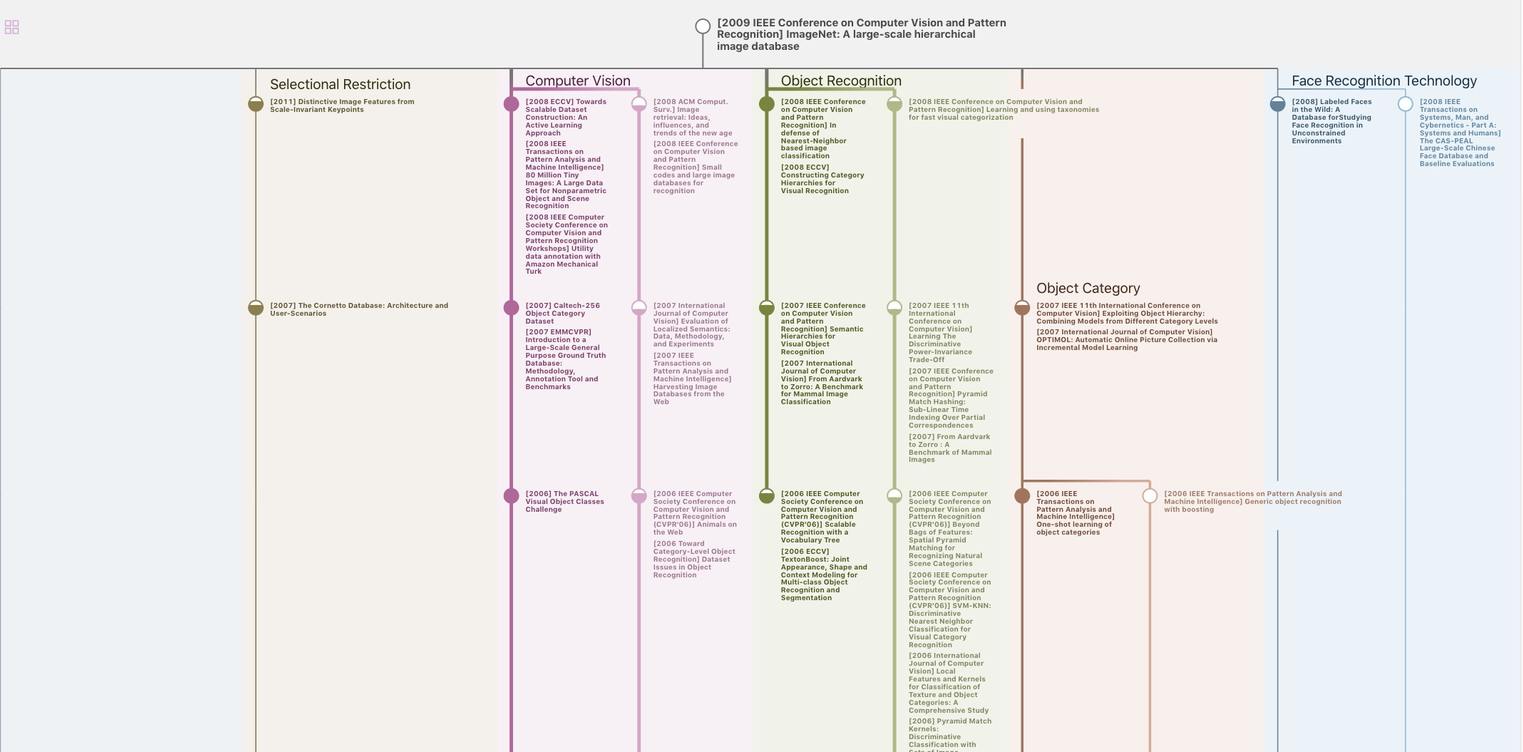
生成溯源树,研究论文发展脉络
Chat Paper
正在生成论文摘要