Study on Feasibility for Artificial Intelligence (AI) Noise Reduction Algorithm with Various Parameters in Pediatric Abdominal Radio-Magnetic Computed Tomography (CT)
JOURNAL OF MAGNETICS(2017)
摘要
The importance of radiation-based images has been increasing due to their ability to provide rapid diagnosis and facilitate treatment of lesions. Among them, the frequency of examination using computed tomography (CT) has been increasing because of this technique's fast examination time and high diagnostic power. However, although the criteria for screening have been presented based on many previous studies on the CT exposure dose for adults, the criteria for children remain inadequate. Especially, relaxing the conditions to reduce the exposure dose of CT will lead to generation of noises. To address this problem, many noise removal algorithms have been developed. Among developed algorithms, a particularly strong interest has been focused on deep learning methods, which are a sort of artificial intelligence (AI)-based machine learning. Therefore, the present study aimed to develop a noise removal algorithm using the Gaussian Mixture Model (GMM), an AI based deep learning method, and to apply the algorithm to pediatric abdominal CT so that to evaluate the usefulness of the approach. PMMA phantoms with different diameters of 12, 16, 20, 24, and 32 cm, which can express pediatric abdomen, were manufactured and used. To evaluate dose and image quality, the tube current was fixed to 200 mAs and the tube voltage was changed from 80 to 120, and 140 kVp; thereafter, the tube voltage was fixed to 120 kVp and the tube currents were changed from 50 to 100, 150, 200, and 250 mAs. According to the results, CTDIw showed a tendency to increase alongside with increases in the tube voltage and the tube currents, while noise decreased proportionally. In addition, the contrast decreased as the tube voltage increased, but was shown to be almost unrelated to the tube currents. Finally, the excellent CNRD result was measured in lowest exposure condition at 80 kVp and 50 mAs. Also, the average of CNRD with AI noise reduction algorithm was 1.6-4.2 times higher than before the application. In conclusion, the doses and characteristics of the pediatric abdominal CT scan according to various image acquisition conditions could be successfully identified and the efficiency of the AI noise removal algorithm developed in the present study was demonstrated.
更多查看译文
关键词
pediatric abdominal computed tomography (CT),Artificial intelligence (AI)-based machine learning,deep learning,image processing,noise reduction algorithm,dose and image quality evaluation
AI 理解论文
溯源树
样例
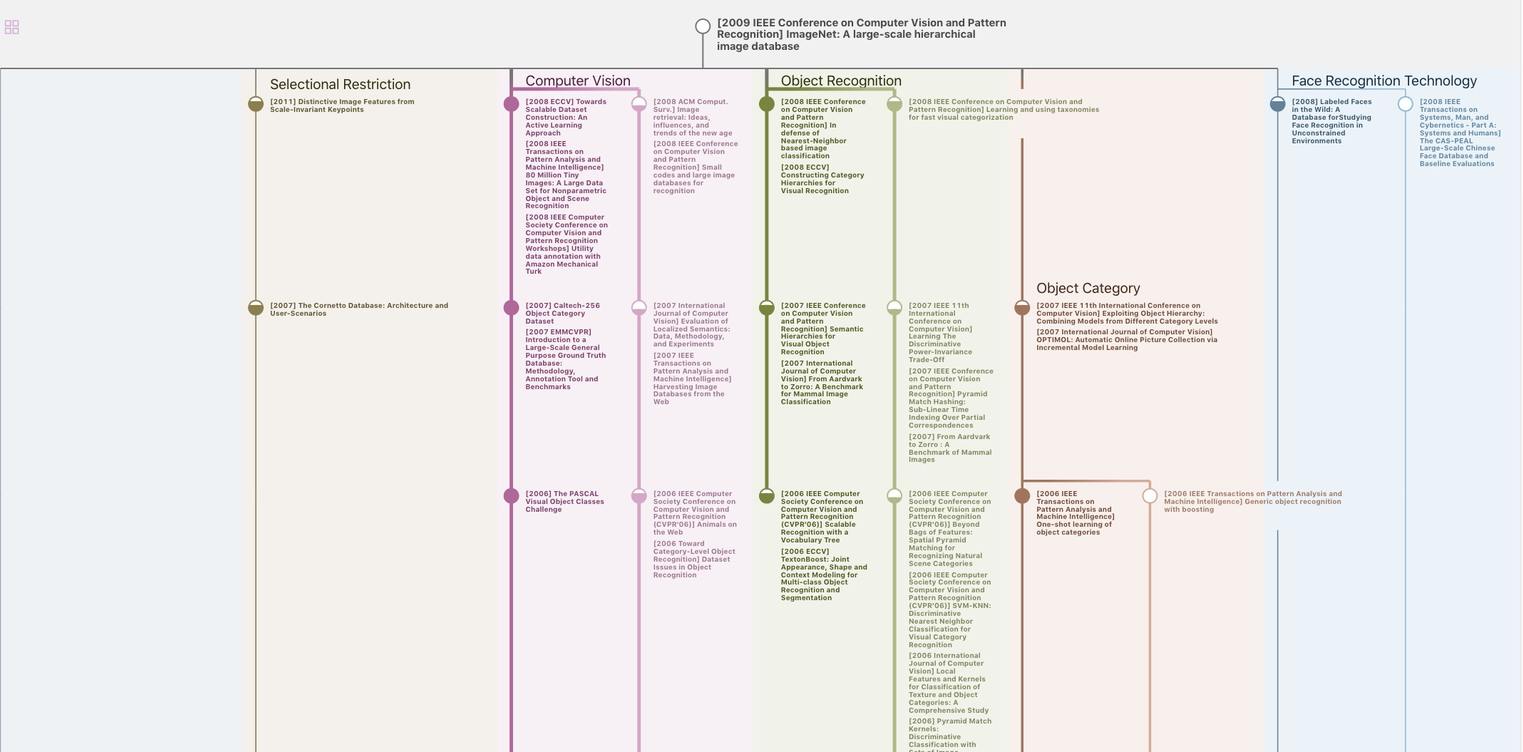
生成溯源树,研究论文发展脉络
Chat Paper
正在生成论文摘要