Learning to Super-Resolve Blurry Face and Text Images
2017 IEEE International Conference on Computer Vision (ICCV)(2017)
摘要
We present an algorithm to directly restore a clear highresolution image from a blurry low-resolution input. This problem is highly ill-posed and the basic assumptions for existing super-resolution methods (requiring clear input) and deblurring methods (requiring high-resolution input) no longer hold. We focus on face and text images and adopt a generative adversarial network (GAN) to learn a category-specific prior to solve this problem. However, the basic GAN formulation does not generate realistic high-resolution images. In this work, we introduce novel training losses that help recover fine details. We also present a multi-class GAN that can process multi-class image restoration tasks, i.e., face and text images, using a single generator network. Extensive experiments demonstrate that our method performs favorably against the state-of-the-art methods on both synthetic and real-world images at a lower computational cost.
更多查看译文
关键词
text images,generative adversarial network,high-resolution images,multiclass image restoration tasks,blurry low-resolution input,super-resolution methods,GAN formulation
AI 理解论文
溯源树
样例
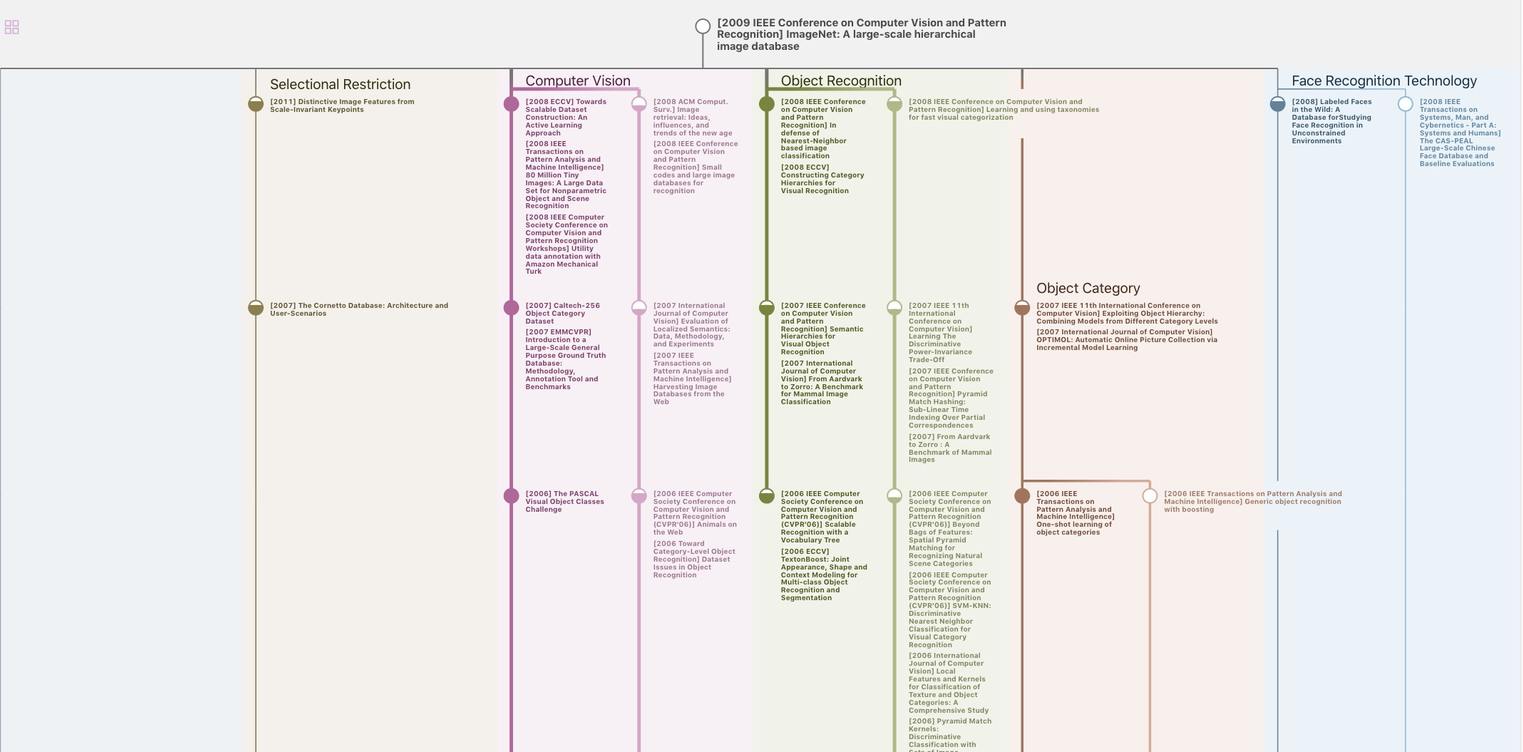
生成溯源树,研究论文发展脉络
Chat Paper
正在生成论文摘要