Deep Learning Can Reverse Photon Migration for Diffuse Optical Tomography.
arXiv: Computer Vision and Pattern Recognition(2017)
摘要
Can artificial intelligence (AI) learn complicated non-linear physics? Here we propose a novel deep learning approach that learns non-linear photon scattering physics and obtains accurate 3D distribution of optical anomalies. In contrast to the traditional black-box deep learning approaches to inverse problems, our deep network learns to invert the Lippmann-Schwinger integral equation which describes the essential physics of photon migration of diffuse near-infrared (NIR) photons in turbid media. As an example for clinical relevance, we applied the method to our prototype diffuse optical tomography (DOT). We show that our deep neural network, trained with only simulation data, can accurately recover the location of anomalies within biomimetic phantoms and live animals without the use of an exogenous contrast agent.
更多查看译文
AI 理解论文
溯源树
样例
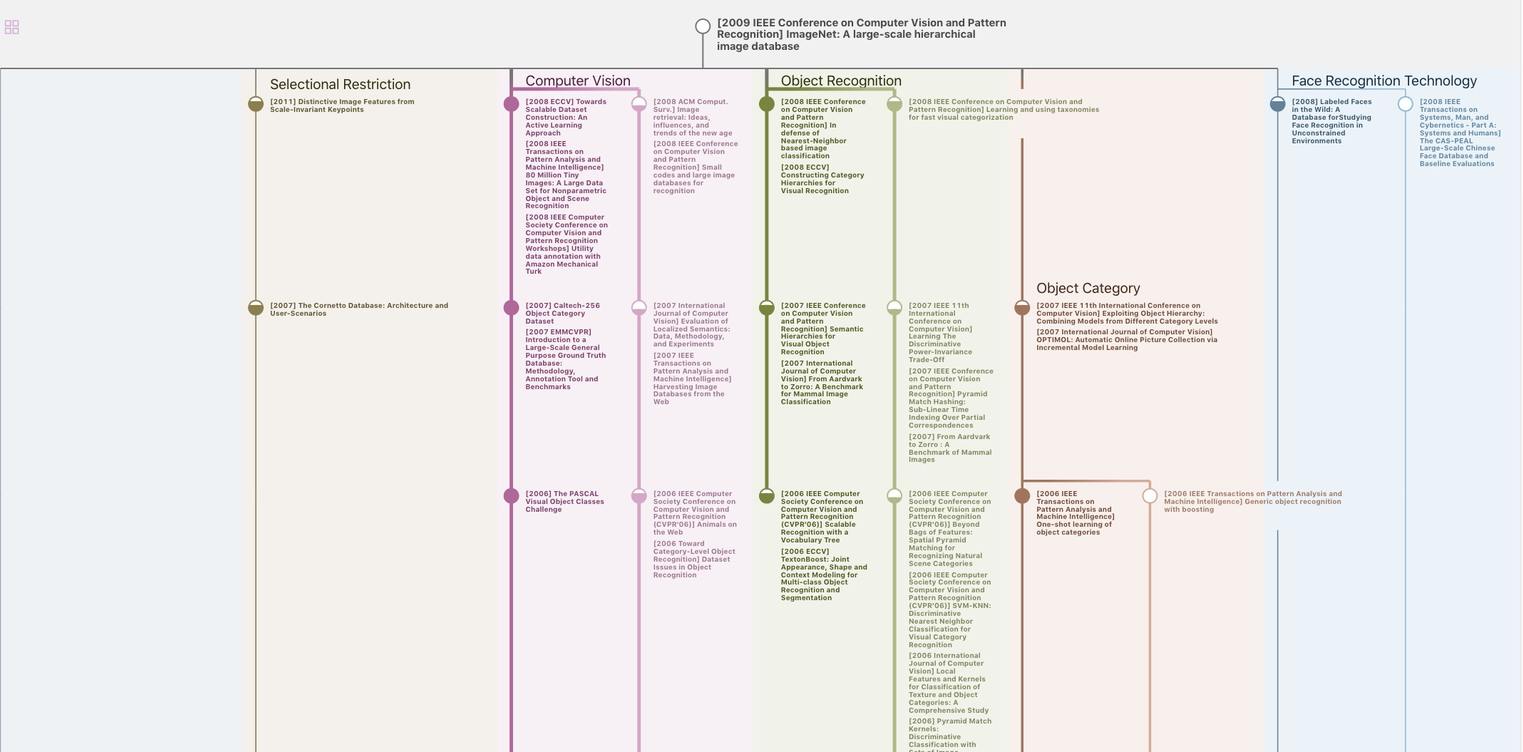
生成溯源树,研究论文发展脉络
Chat Paper
正在生成论文摘要