Feature selection using firefly optimization for classification and regression models.
Decision Support Systems(2018)
摘要
In this research, we propose a variant of the Firefly Algorithm (FA) for discriminative feature selection in classification and regression models for supporting decision making processes using data-based learning methods. The FA variant employs Simulated Annealing (SA)-enhanced local and global promising solutions, chaotic-accelerated attractiveness parameters and diversion mechanisms of weak solutions to escape from the local optimum trap and mitigate the premature convergence problem in the original FA algorithm. A total of 29 classification and 11 regression benchmark data sets have been used to evaluate the efficiency of the proposed FA model. It shows statistically significant improvements over other state-of-the-art FA variants and classical search methods for diverse feature selection problems. In short, the proposed FA variant offers an effective method to identify optimal feature subsets in classification and regression models for supporting data-based decision making processes.
更多查看译文
关键词
Feature selection,Dimensionality reduction,Classification,Regression,Firefly algorithm
AI 理解论文
溯源树
样例
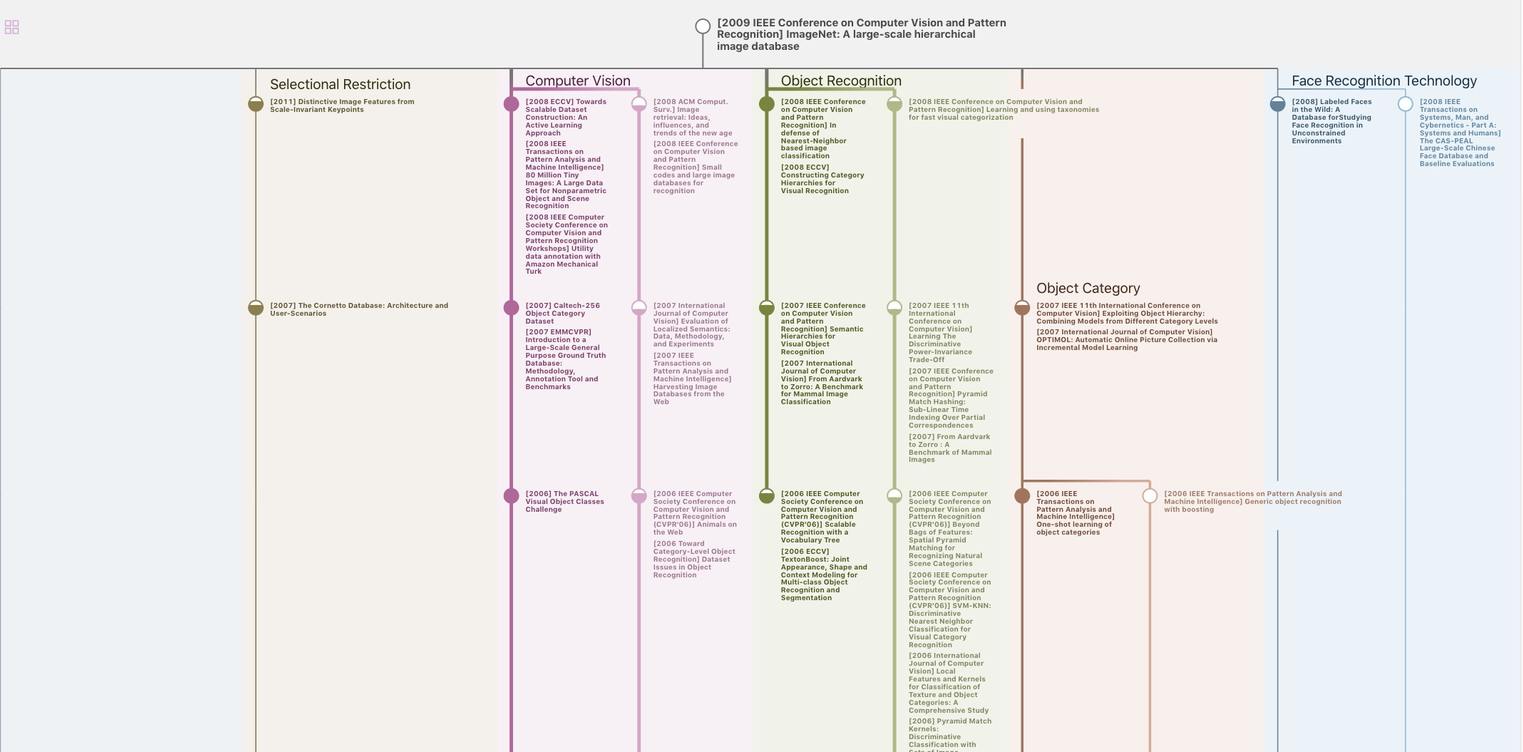
生成溯源树,研究论文发展脉络
Chat Paper
正在生成论文摘要