STExNMF: Spatio-Temporally Exclusive Topic Discovery for Anomalous Event Detection
2017 IEEE International Conference on Data Mining (ICDM)(2017)
摘要
Understanding newly emerging events or topics associated with a particular region of a given day can provide deep insight on the critical events occurring in highly evolving metropolitan cities. We propose herein a novel topic modeling approach on text documents with spatio-temporal information (e.g., when and where a document was published) such as location-based social media data to discover prevalent topics or newly emerging events with respect to an area and a time point. We consider a map view composed of regular grids or tiles with each showing topic keywords from documents of the corresponding region. To this end, we present a tilebased spatio-temporally exclusive topic modeling approach called STExNMF, based on a novel nonnegative matrix factorization (NMF) technique. STExNMF mainly works based on the two following stages: (1) first running a standard NMF of each tile to obtain general topics of the tile and (2) running a spatiotemporally exclusive NMF on a weighted residual matrix. These topics likely reveal information on newly emerging events or topics of interest within a region. We demonstrate the advantages of our approach using the geo-tagged Twitter data of New York City. We also provide quantitative comparisons in terms of the topic quality, spatio-temporal exclusiveness, topic variation, and qualitative evaluations of our method using several usage scenarios. In addition, we present a fast topic modeling technique of our model by leveraging parallel computing.
更多查看译文
关键词
Topic modeling,social network analysis,matrix factorization,event detection,anomaly detection
AI 理解论文
溯源树
样例
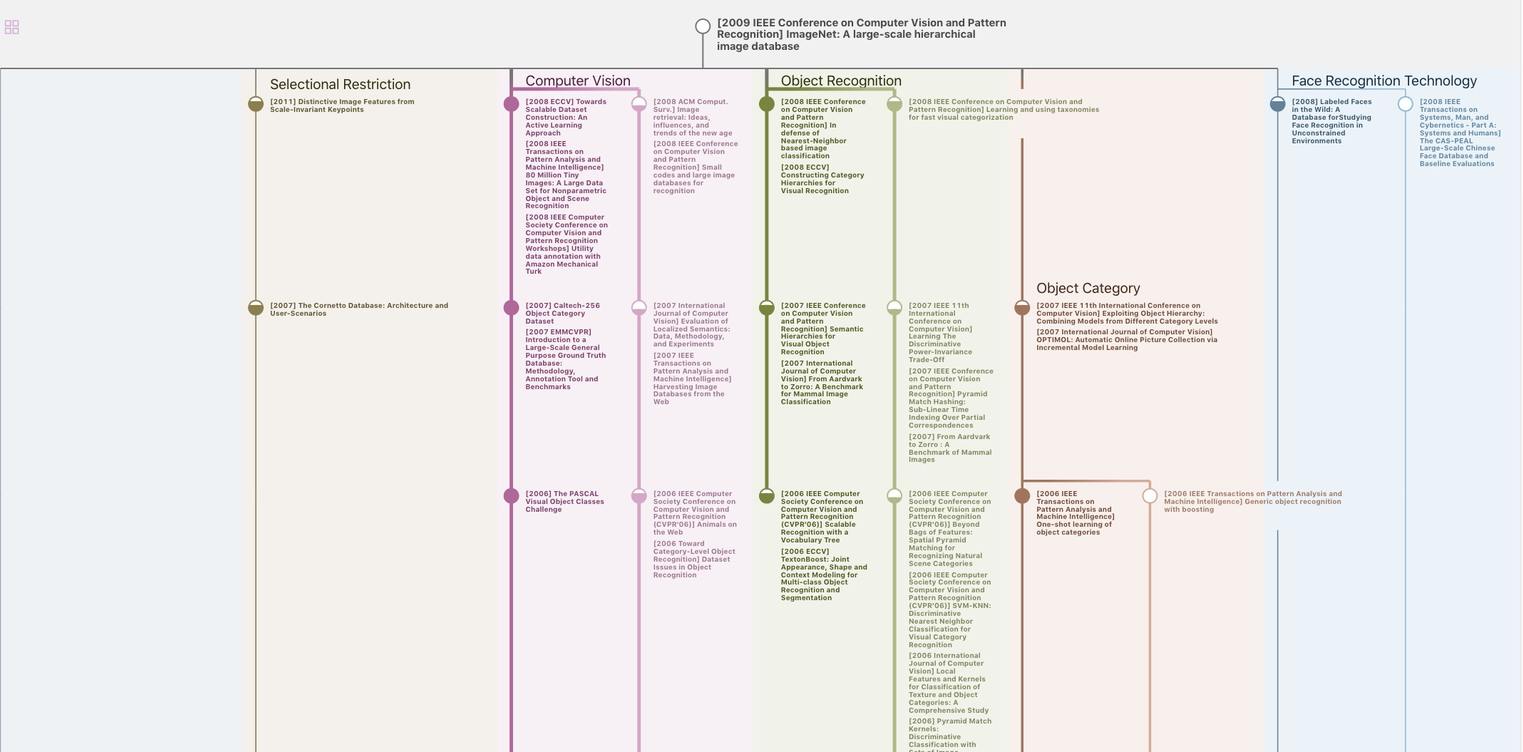
生成溯源树,研究论文发展脉络
Chat Paper
正在生成论文摘要