Simulation optimization approach for solving stochastic programming
2017 2nd IEEE International Conference on Computational Intelligence and Applications (ICCIA)(2017)
摘要
Simulation Optimization is computationally expensive, especially in large-scale stochastic problem solving, where the computational budget is considered as an important factor. A higher computational budget attempts to generate highly accurate solutions while a lower budget might result in biased or unrealistic solutions. In this paper, the effect of computational budget on the quality of the solution, in the context of Simulation Optimization, has been studied. The study is conducted by combining a Multi-operator Differential Evolutionary Algorithm with Monte-Carlo simulation. For experimentation, the stochastic test problems were generated based on the IEEE-CEC'2006 constrained optimization competition test problems. The experimental results provide interesting insights about the behavior of simulation optimization that would allow to reduce the computational time not compromising the quality of solutions.
更多查看译文
关键词
simulation optimization,differential evolutionary,optimal computing budget allocation,multi-fidelity simulation optimization
AI 理解论文
溯源树
样例
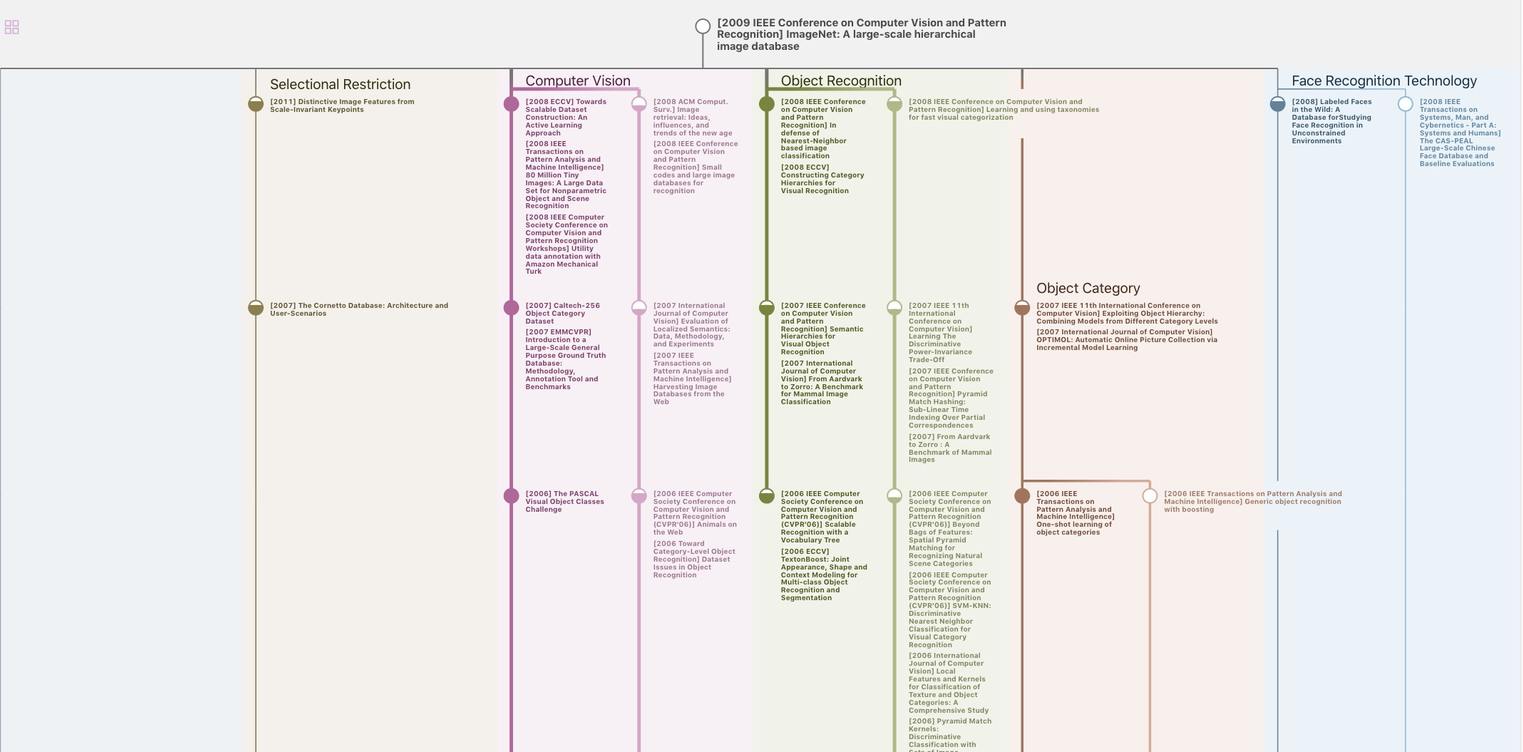
生成溯源树,研究论文发展脉络
Chat Paper
正在生成论文摘要