Application of wavelet scattering networks in classification of ultrasound image sequences
2017 IEEE International Ultrasonics Symposium (IUS)(2017)
摘要
Recently, ultrasound imaging of muscle contractions has been used by several research groups to infer volitional motor intent of the user, and has shown promise as a novel muscle computer interface. Learning spatiotemporal features from ultrasound image sequences is challenging because of deformations introduced by probe repositioning. The image features are sensitive to probe placement and even small displacements during cross-session donning and doffing of the probe could compromise the classification accuracy when using a model trained on a previous session. This requires frequent recalibration. Deep learning based feature extractors have been shown to be invariant to translation, rotation and slight deformations. In this study, we investigated the feasibility of wavelet-based deep scattering features to preserve motion classification accuracy across multiple donning and doffing sessions. It was demonstrated that scattering features, which do not require learning filter weights, can significantly improve the cross-session classification accuracy by 20-30%. It can be concluded that these features are robust to minor probe displacements. They need to be further investigated with a larger data set to investigate their robustness in accurately classifying different muscle movements.
更多查看译文
关键词
wavelet scattering networks,ultrasound image sequence classification,ultrasound imaging,muscle contractions,volitional motor intent,muscle computer interface,spatiotemporal features,probe repositioning,donning,doffing,deep learning,feature extractors,rotation,slight deformations,wavelet-based deep scattering features,motion classification accuracy
AI 理解论文
溯源树
样例
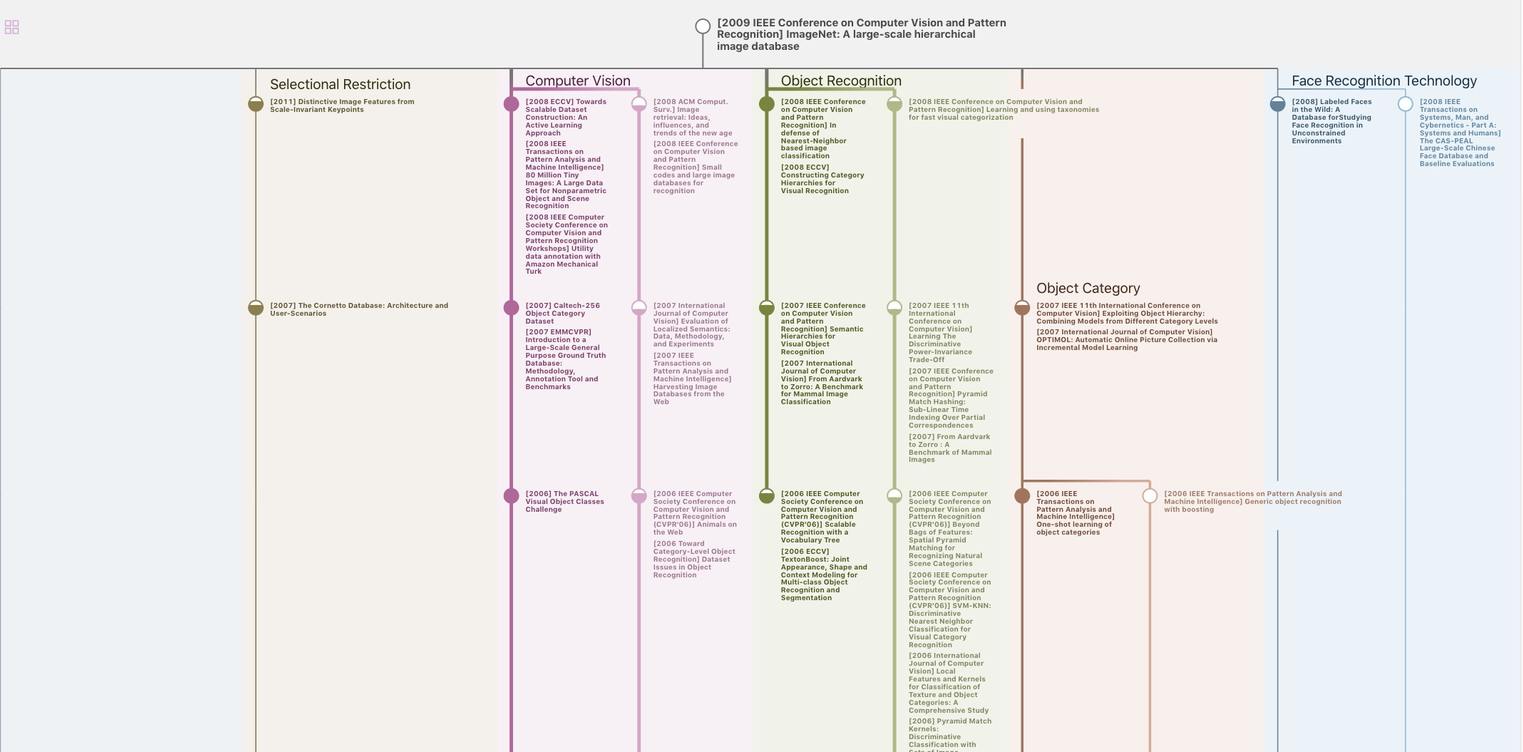
生成溯源树,研究论文发展脉络
Chat Paper
正在生成论文摘要