Support vector machine with Dirichlet feature mapping.
Neural Networks(2018)
摘要
The Support Vector Machine (SVM) is a supervised learning algorithm to analyze data and recognize patterns. The standard SVM suffers from some limitations in nonlinear classification problems. To tackle these limitations, the nonlinear form of the SVM poses a modified machine based on the kernel functions or other nonlinear feature mappings obviating the mentioned imperfection. However, choosing an efficient kernel or feature mapping function is strongly dependent on data structure. Thus, a flexible feature mapping can be confidently applied in different types of data structures without challenging a kernel selection and its tuning. This paper introduces a new flexible feature mapping approach based on the Dirichlet distribution in order to develop an efficient SVM for nonlinear data structures. To determine the parameters of the Dirichlet mapping, a tuning technique is employed based on the maximum likelihood estimation and Newton’s optimization method. The numerical results illustrate the superiority of the proposed machine in terms of the accuracy and relative error rate measures in comparison to the traditional ones.
更多查看译文
关键词
Supervised learning,Support vector machine,Nonlinear mapping,Kernel function,Dirichlet distribution
AI 理解论文
溯源树
样例
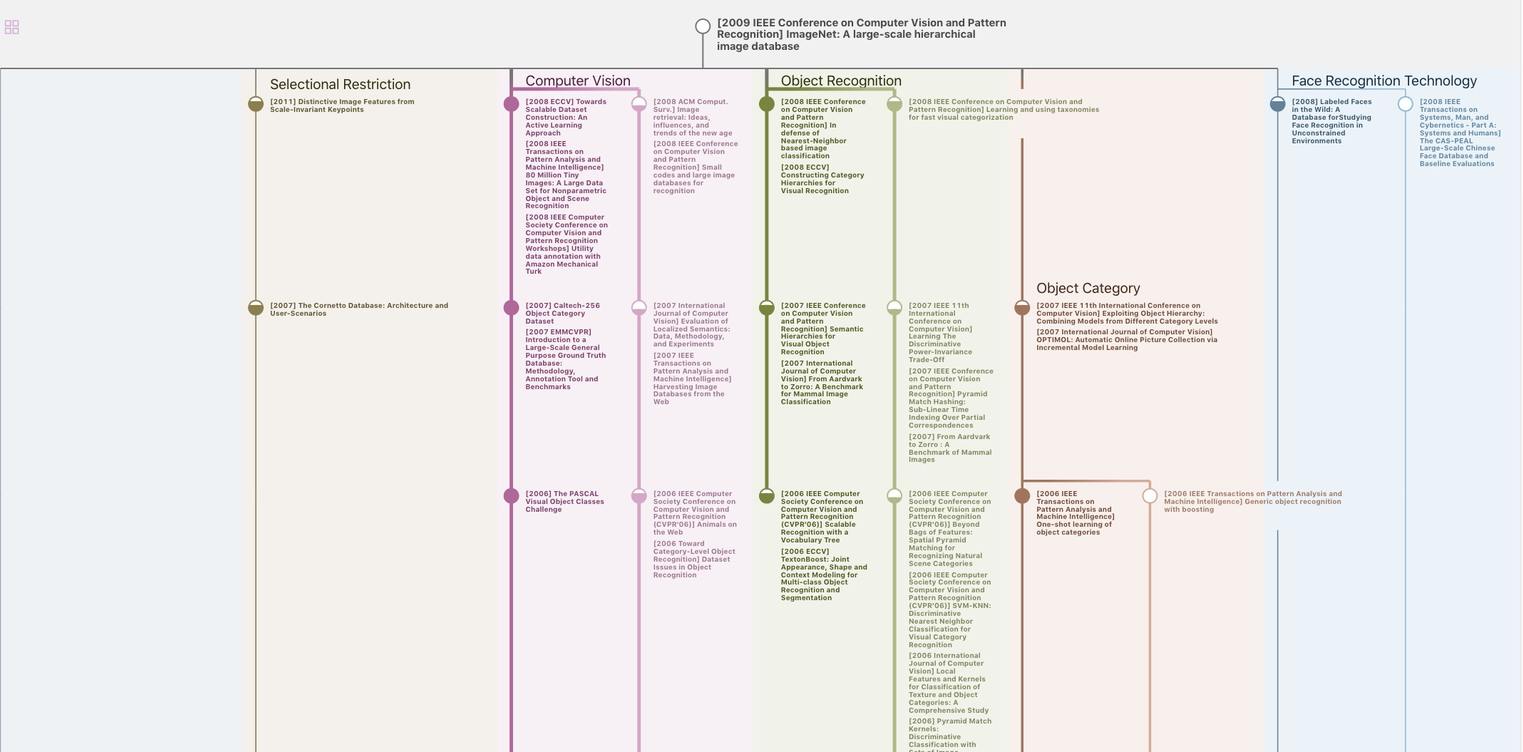
生成溯源树,研究论文发展脉络
Chat Paper
正在生成论文摘要