User Modelling for Avoiding Overfitting in Interactive Knowledge Elicitation for Prediction.
IUI(2018)
摘要
In human-in-the-loop machine learning, the user provides information beyond that in the training data. Many algorithms and user interfaces have been designed to optimize and facilitate this human--machine interaction; however, fewer studies have addressed the potential defects the designs can cause. Effective interaction often requires exposing the user to the training data or its statistics. The design of the system is then critical, as this can lead to double use of data and overfitting, if the user reinforces noisy patterns in the data. We propose a user modelling methodology, by assuming simple rational behaviour, to correct the problem. We show, in a user study with 48 participants, that the method improves predictive performance in a sparse linear regression sentiment analysis task, where graded user knowledge on feature relevance is elicited. We believe that the key idea of inferring user knowledge with probabilistic user models has general applicability in guarding against overfitting and improving interactive machine learning.
更多查看译文
关键词
Interactive machine learning, probabilistic modelling, Bayesian inference, overfitting, expert prior elicitation, human-in-the-loop machine learning
AI 理解论文
溯源树
样例
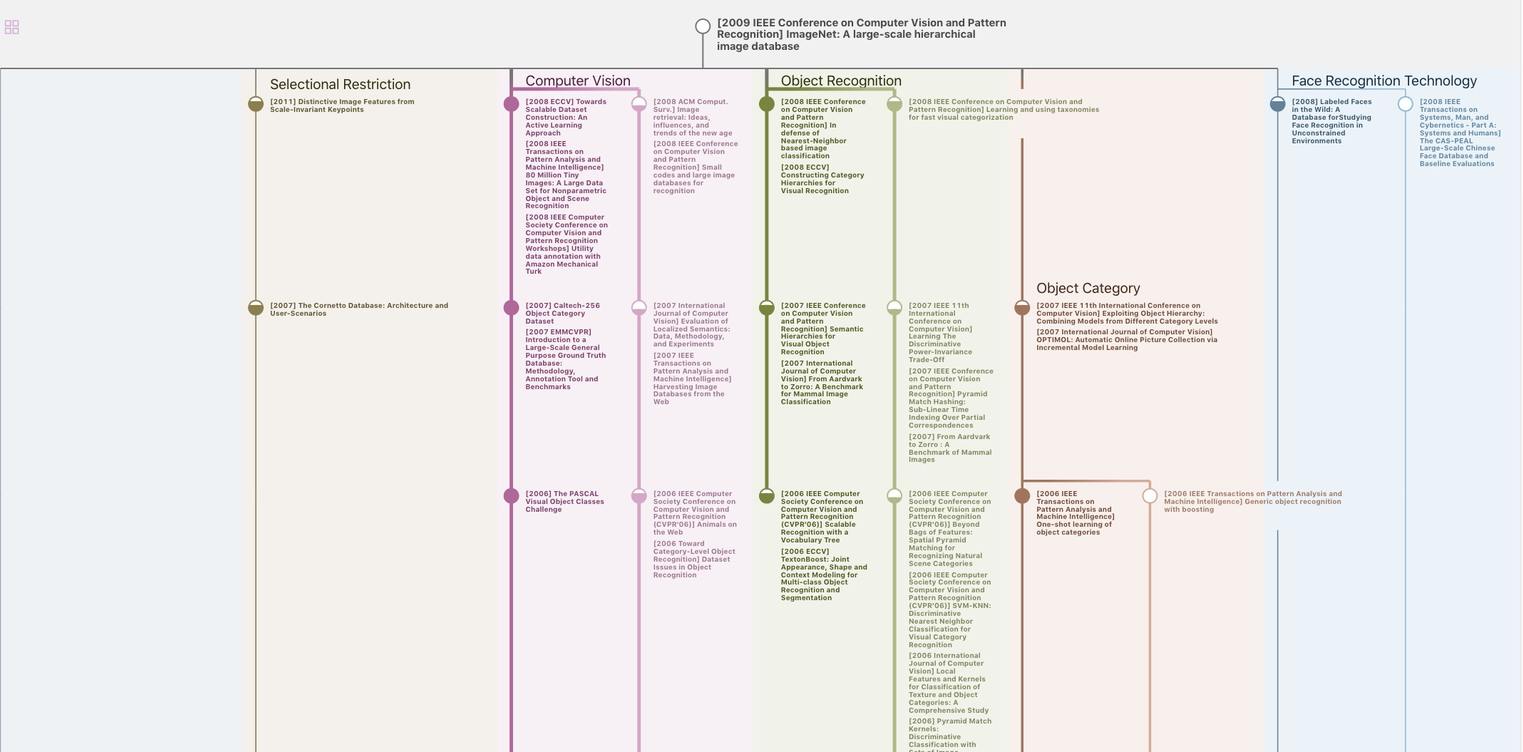
生成溯源树,研究论文发展脉络
Chat Paper
正在生成论文摘要