Testing for Marginal Linear Effects in Quantile Regression.
JOURNAL OF THE ROYAL STATISTICAL SOCIETY SERIES B-STATISTICAL METHODOLOGY(2018)
摘要
The paper develops a new marginal testing procedure to detect significant predictors that are associated with the conditional quantiles of a scalar response. The idea is to fit the marginal quantile regression on each predictor one at a time, and then to base the test on the t-statistics that are associated with the most predictive predictors. A resampling method is devised to calibrate this test statistic, which has non-regular limiting behaviour due to the selection of the most predictive variables. Asymptotic validity of the procedure is established in a general quantile regression setting in which the marginal quantile regression models can be misspecified. Even though a fixed dimension is assumed to derive the asymptotic results, the test proposed is applicable and computationally feasible for large dimensional predictors. The method is more flexible than existing marginal screening test methods based on mean regression and has the added advantage of being robust against outliers in the response. The approach is illustrated by using an application to a human immunodeficiency virus drug resistance data set.
更多查看译文
关键词
Bootstrap calibration,Inference,Marginal regression,Non-standard asymptotics,Quantile regression
AI 理解论文
溯源树
样例
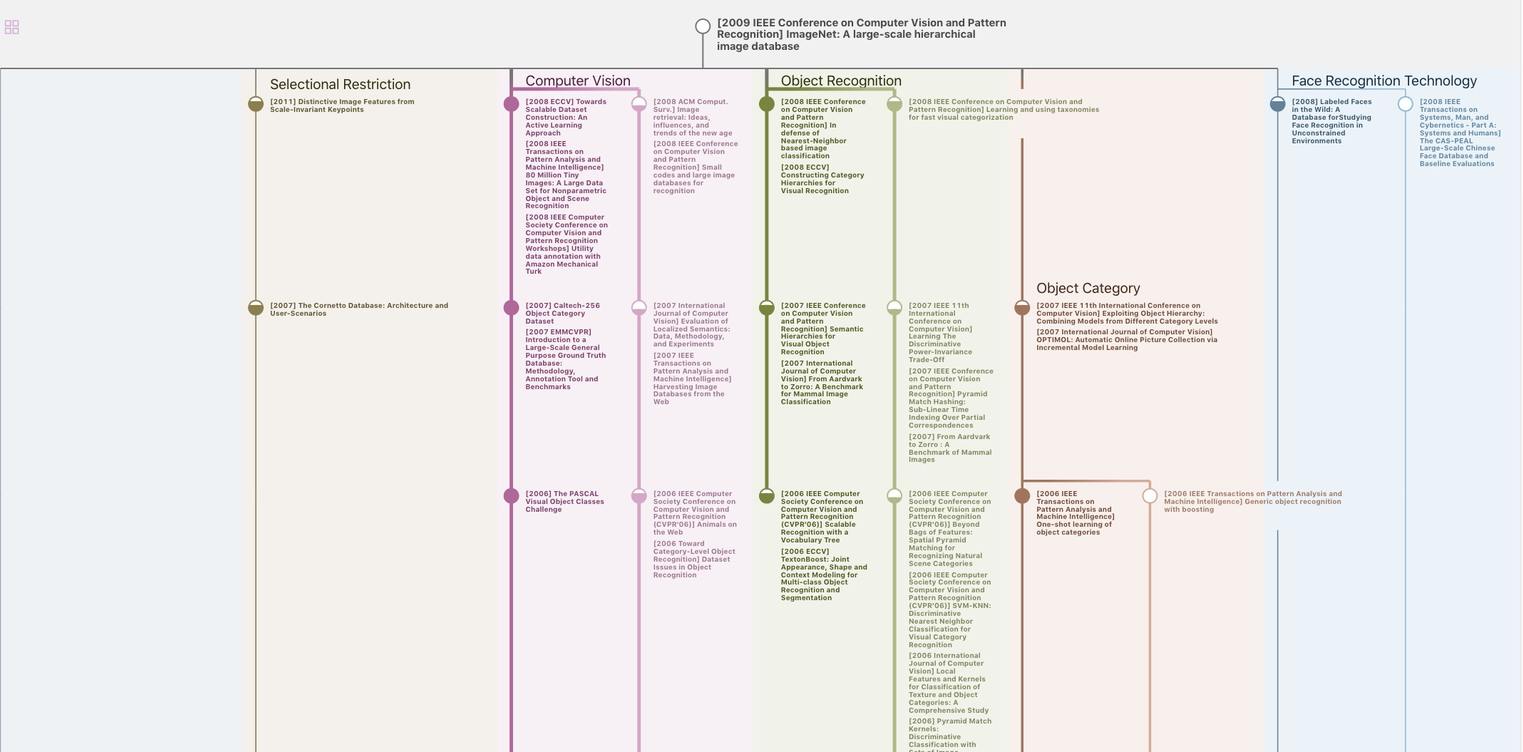
生成溯源树,研究论文发展脉络
Chat Paper
正在生成论文摘要