Accelerated Block Coordinate Proximal Gradients with Applications in High Dimensional Statistics
arXiv (Cornell University)(2017)
摘要
Nonconvex optimization problems arise in different research fields and arouse lots of attention in signal processing, statistics and machine learning. In this work, we explore the accelerated proximal gradient method and some of its variants which have been shown to converge under nonconvex context recently. We show that a novel variant proposed here, which exploits adaptive momentum and block coordinate update with specific update rules, further improves the performance of a broad class of nonconvex problems. In applications to sparse linear regression with regularizations like Lasso, grouped Lasso, capped $\ell_1$ and SCAP, the proposed scheme enjoys provable local linear convergence, with experimental justification.
更多查看译文
关键词
gradients,block
AI 理解论文
溯源树
样例
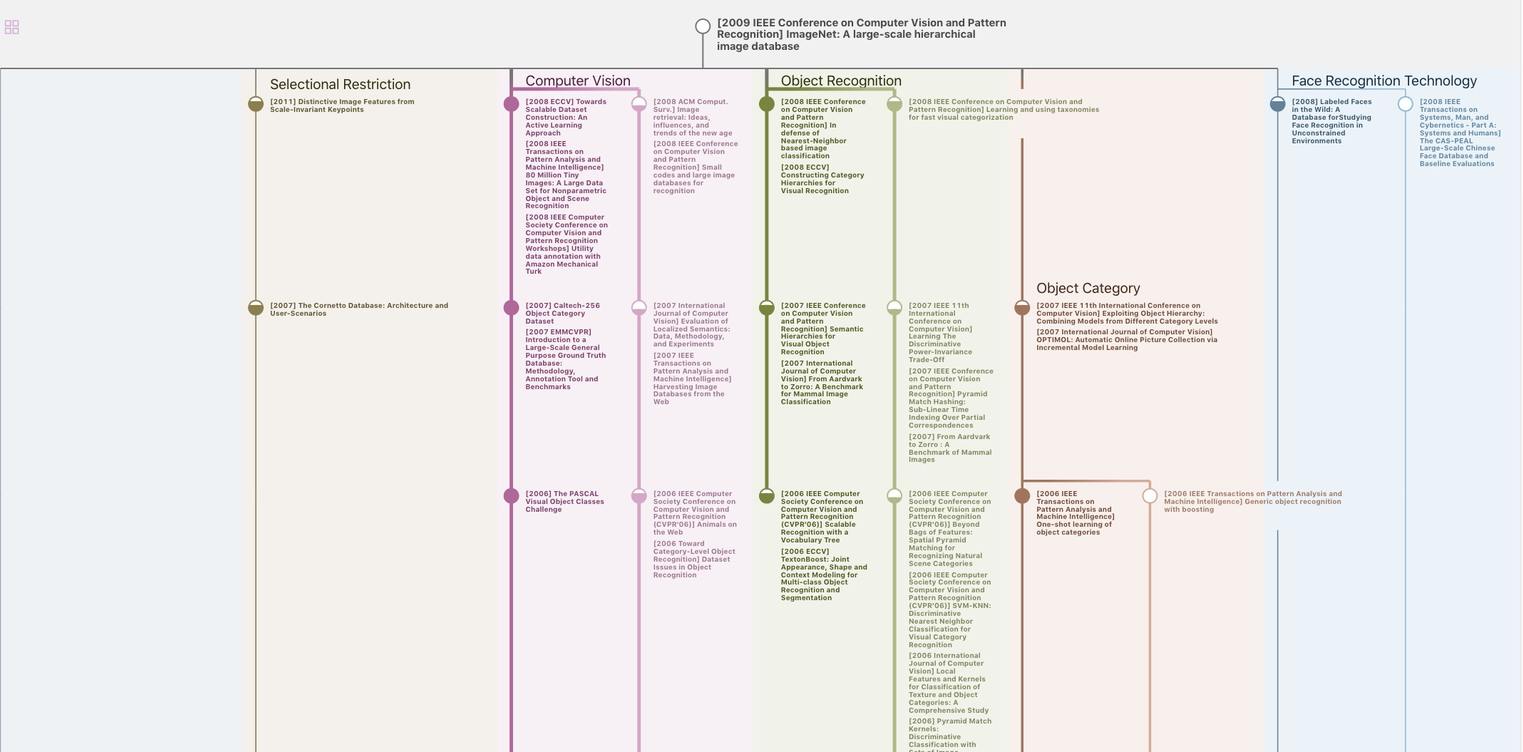
生成溯源树,研究论文发展脉络
Chat Paper
正在生成论文摘要