An Introduction to Wishart Matrix Moments
Periodicals(2018)
摘要
AbstractThese lecture notes provide a comprehensive, self-contained introduction to the analysis of Wishart matrix moments. This study may act as an introduction to some particular aspects of random matrix theory, or as a self-contained exposition of Wishart matrix moments. Random matrix theory plays a central role in statistical physics, computational mathematics and engineering sciences, including data assimilation, signal processing, combinatorial optimization, compressed sensing, econometrics and mathematical finance, among numerous others. The mathematical foundations of the theory of random matrices lies at the intersection of combinatorics, non-commutative algebra, geometry, multivariate functional and spectral analysis, and of course statistics and probability theory. As a result, most of the classical topics in random matrix theory are technical, and mathematically difficult to penetrate for non-experts and regular users and practitioners. The technical aim of these notes is to review and extend some important results in random matrix theory in the specific context of real random Wishart matrices. This special class of Gaussian-type sample covariance matrix plays an important role in multivariate analysis and in statistical theory.We derive non-asymptotic formulae for the full matrix moments of real valued Wishart random matrices. As a corollary, we derive and extend a number of spectral and trace-type results for the case of non-isotropic Wishart random matrices. We also derive the full matrix moment analogues of some classic spectral and trace-type moment results. For example, we derive semi-circle and Marchencko–Pastur-type laws in the non-isotropic and full matrix cases. Laplace matrix transforms and matrix moment estimates are also studied, along with new spectral and trace concentration-type inequalities.
更多查看译文
关键词
Machine Learning,Signal Processing,Communications and Information Theory,Spectral methods,Statistical/machine learning,Information theory and statistics
AI 理解论文
溯源树
样例
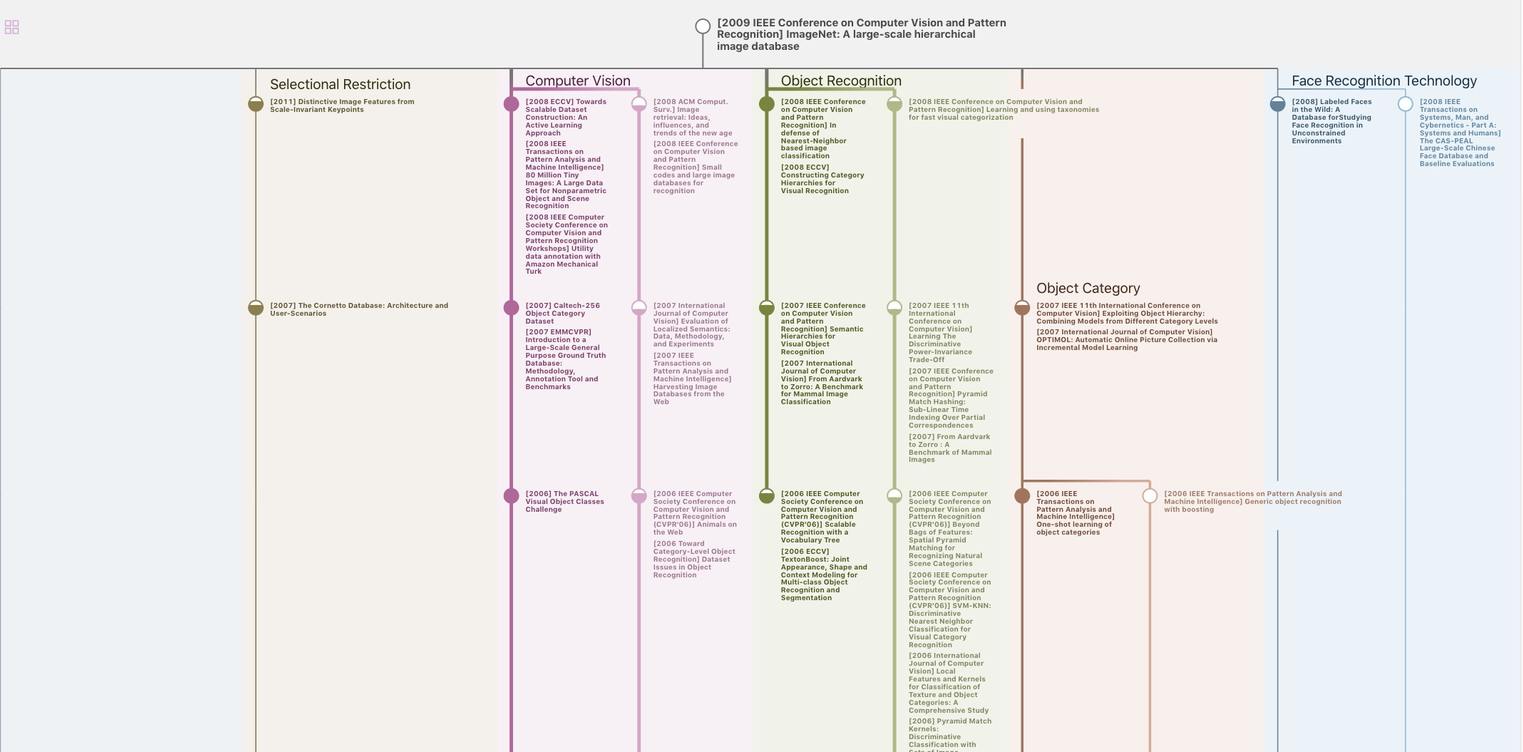
生成溯源树,研究论文发展脉络
Chat Paper
正在生成论文摘要