Estimating soil organic matter content from Hyperion reflectance images using PLSR, PCR, MinR and SWR models in semi-arid regions of Iran
Environmental Development(2018)
摘要
Soil organic matter is highly pivotal as it can improve physical, chemical and biological properties of soil through various functions. Direct measurement of soil organic matter at large scales requires a great number of soil samples which is time consuming, tedious and costly. Consequently, alternative methods must be developed to provide a rapid overview of soil organic matter with reasonable accuracy at large scales. Remote sensing can be considered as a non-destructive, rapid and inexpensive method for such purpose. Among different remote sensing features, hyperspectral spectroscopy may produce inexpensive, quick and accurate way of producing soil organic matter maps at large scales. This study aimed to assess the feasibility of providing accurate soil organic matter distribution maps for large semi-arid areas of Iran. Consequently, some Hyperion images were used to develop relationships between spectral bands and soil organic matter with several methods including Stepwise Regression (SWR), Minimum Regression (MinR), Partial Least Square Regression (PLSR) and Principle Component Regression (PCR) models. Models were first calibrated with Hyperion images of the Ivanekey region and then verified by using 9 random samples from the Ivanekey and 23 samples from the Uromia semi-arid regions. Results indicated that of the applied models, SWR and PLSR can provide reasonable accuracy (RMSE) to predict soil organic matter in entire semi-arid region. However, more investigations are needed to improve the accuracy of such predictive models for arid and semi-arid regions with relatively low organic matter content.
更多查看译文
关键词
Hyperion,Principle component analysis,Soil organic matter
AI 理解论文
溯源树
样例
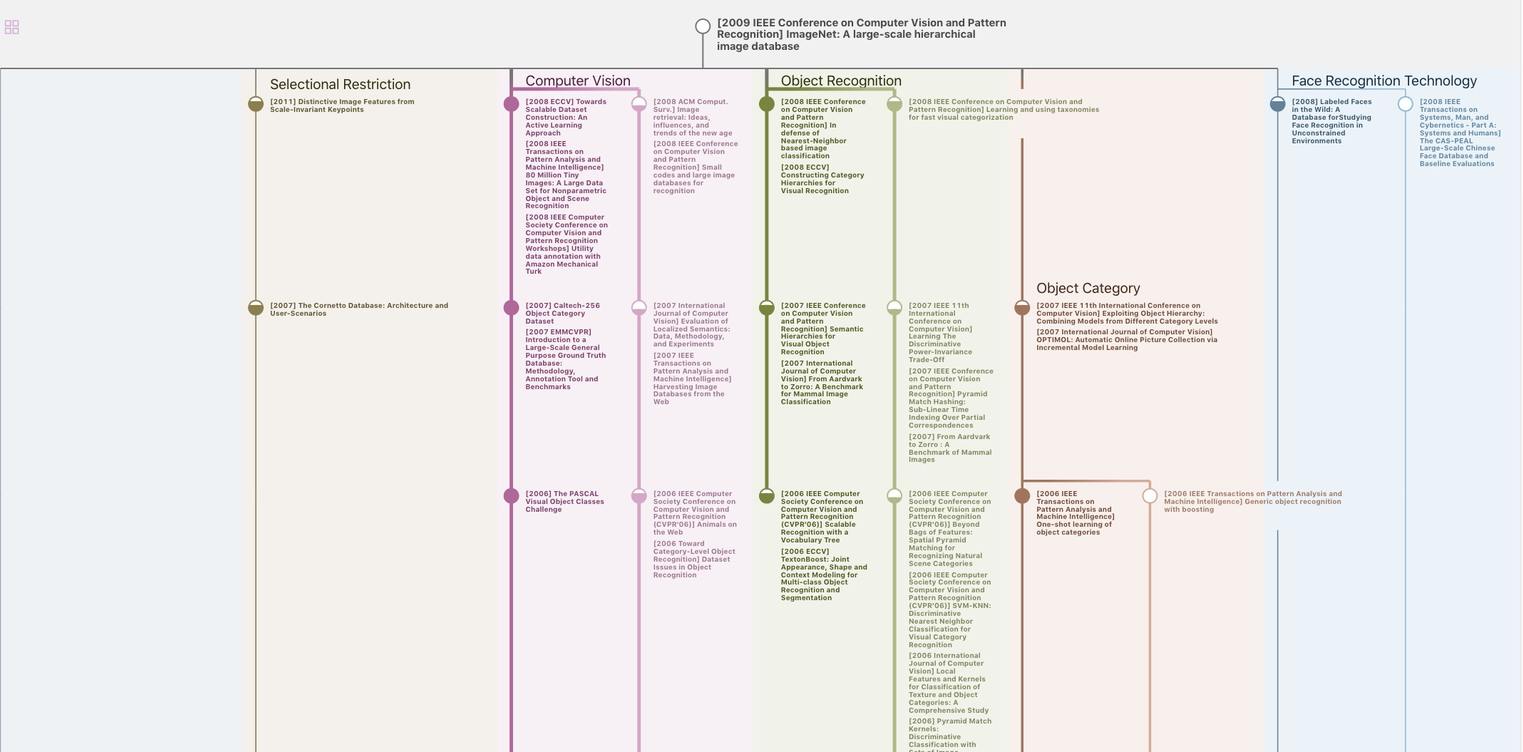
生成溯源树,研究论文发展脉络
Chat Paper
正在生成论文摘要