DriftInsight: Detecting Anomalous Behaviors in Large-Scale Cloud Platform
2017 IEEE 10th International Conference on Cloud Computing (CLOUD)(2017)
摘要
Detecting anomalous behaviors of cloud platforms is one of critical tasks for cloud providers. Every anomalous behavior potentially causes incidents, especially some unaware and/or unknown issues, which severely harm their SLA (Service Level Agreement). Existing solutions generally monitor cloud platform at different layers and then detect anomalies based on rules or learning algorithms on monitoring metrics. However, complexity of nowadays cloud platforms, high dynamics of cloud workloads and thousands of various types of metrics make anomalous behavior detection more challenging to be applied in production, especially in large scale cloud production environments. In this paper, we present a practical cloud anomalous behavior detection system called DriftInsight. It firstly analyzes multi-denominational metrics of each component and identifies a set of representative steady components based on the convergences of their states. Then it generates a state model and a state transit model for each steady cloud component. Finally, it detects behavior anomalies of these steady components in near real-time and meanwhile evolve behavior models on the fly. The evaluation results of this approach in a commercial large-scale PaaS (Platform-as-a-Service) cloud are demonstrated its capability and efficiency.
更多查看译文
关键词
cloud computing,behavior model,anomaly detection,unsupervised clustering
AI 理解论文
溯源树
样例
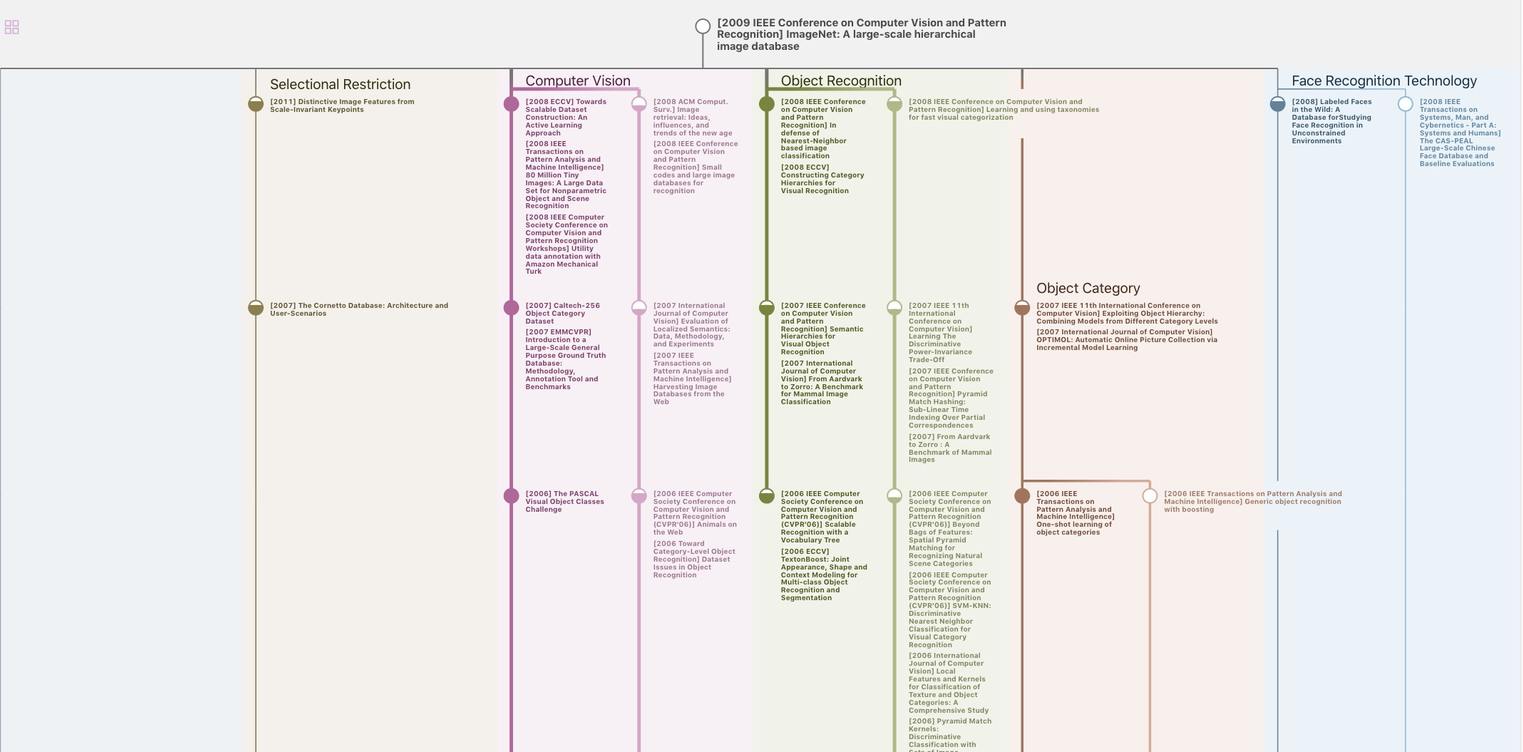
生成溯源树,研究论文发展脉络
Chat Paper
正在生成论文摘要