Differentially Private Regression for Discrete-Time Survival Analysis
CIKM(2017)
摘要
In survival analysis, regression models are used to understand the effects of explanatory variables (e.g., age, sex, weight, etc.) to the survival probability. However, for sensitive survival data such as medical data, there are serious concerns about the privacy of individuals in the data set when medical data is used to fit the regression models. The closest work addressing such privacy concerns is the work on Cox regression which linearly projects the original data to a lower dimensional space. However, the weakness of this approach is that there is no formal privacy guarantee for such projection. In this work, we aim to propose solutions for the regression problem in survival analysis with the protection of differential privacy which is a golden standard of privacy protection in data privacy research. To this end, we extend the Output Perturbation and Objective Perturbation approaches which are originally proposed to protect differential privacy for the Empirical Risk Minimization (ERM) problems. In addition, we also propose a novel sampling approach based on the Markov Chain Monte Carlo (MCMC) method to practically guarantee differential privacy with better accuracy. We show that our proposed approaches achieve good accuracy as compared to the non-private results while guaranteeing differential privacy for individuals in the private data set.
更多查看译文
关键词
survival analysis, discrete-time models, differential privacy, regression models
AI 理解论文
溯源树
样例
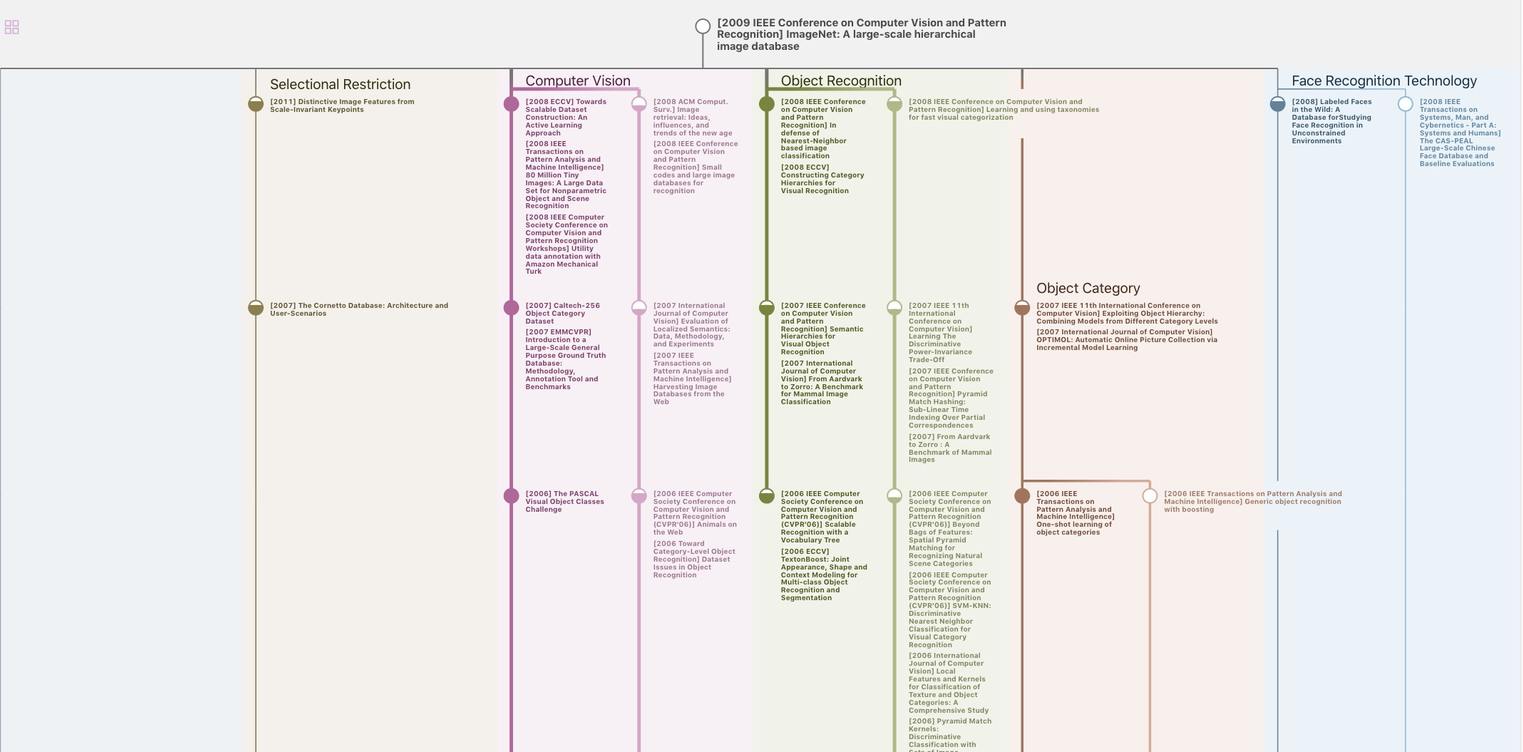
生成溯源树,研究论文发展脉络
Chat Paper
正在生成论文摘要