A Distribution Free Formulation Of The Total Variability Model
18TH ANNUAL CONFERENCE OF THE INTERNATIONAL SPEECH COMMUNICATION ASSOCIATION (INTERSPEECH 2017), VOLS 1-6: SITUATED INTERACTION(2017)
摘要
The Total Variability Model (TVM) [1] has been widely used in audio signal processing as a framework for capturing differences in feature space distributions across variable length sequences by mapping them into a fixed-dimensional representation. Its formulation requires making an assumption about the source data distribution being a Gaussian Mixture Model (GMM). In this paper, we show that it is possible to arrive at the same model formulation without requiring such an assumption about distribution of the data, by showing asymptotic normality of the statistics used to estimate the model. We highlight some connections between TVM and heteroscedastic Principal Component Analysis (PCA), as well as the matrix completion problem, which lead to a computationally efficient formulation of the Maximum Likelihood estimation problem for the model.
更多查看译文
关键词
Total Variability Model, ivector
AI 理解论文
溯源树
样例
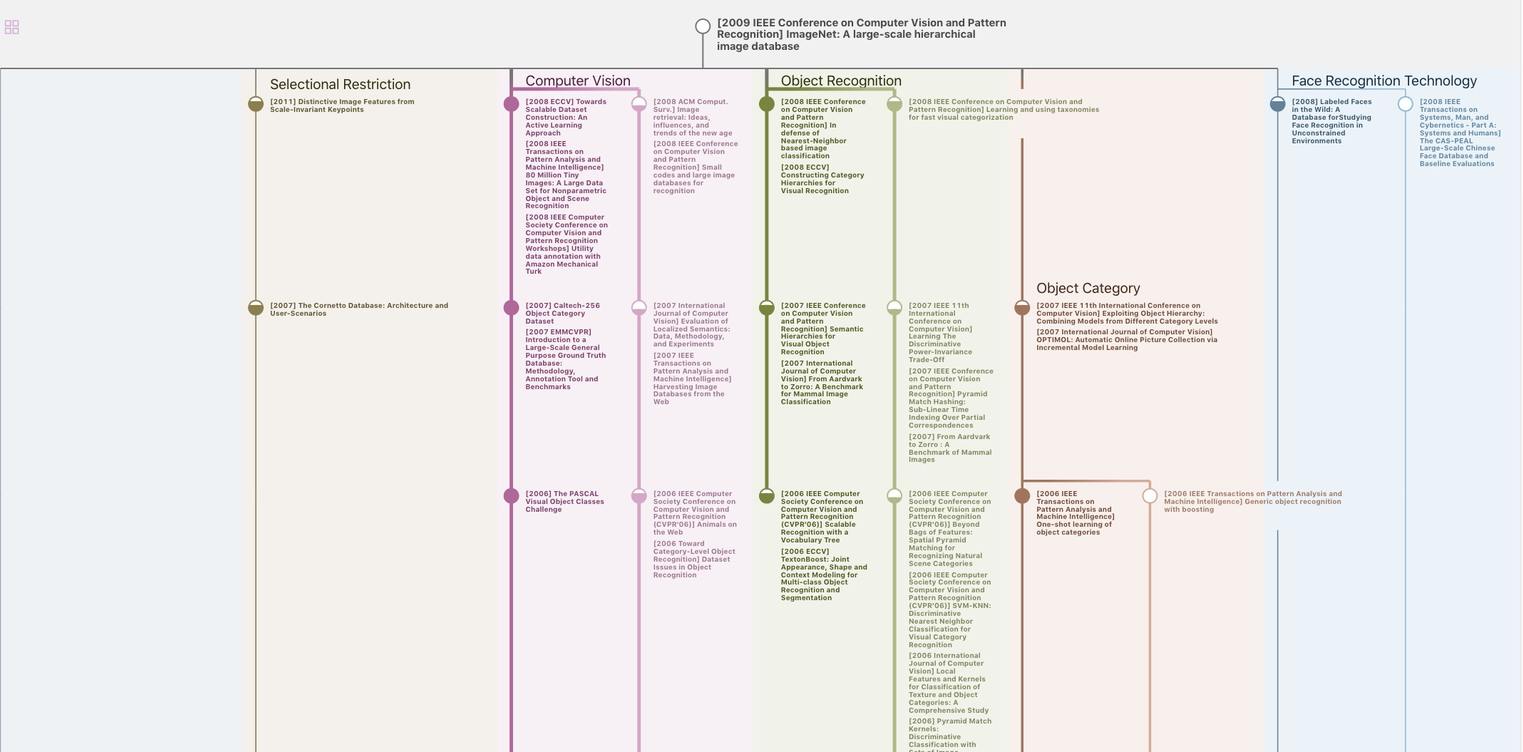
生成溯源树,研究论文发展脉络
Chat Paper
正在生成论文摘要