MIMIC: Using passive network measurements to estimate HTTP-based adaptive video QoE metrics.
TMA CONFERENCE 2017 - PROCEEDINGS OF THE 1ST NETWORK TRAFFIC MEASUREMENT AND ANALYSIS CONFERENCE(2017)
摘要
HTTP-based Adaptive Streaming (HAS) has seen a major growth in the cellular networks. As a key application and network demand driver, user-perceived Quality of Experience (QoE) of video streaming contributes to the overall user satisfaction. Therefore, it becomes critical for the cellular network operators to understand the QoE of video streams. It can help with long-term network planning and provisioning and QoE-aware traffic management. However, tracking QoE is challenging as network operators do not have direct access to the video streaming apps, user devices or servers. In this paper, we provide a methodology that uses passive network measurements of unencrypted HAS video streams to estimate three key video QoE metrics-average bitrate, re-buffering ratio and bitrate switches. Our approach relies on the semantics of HAS to model a video session on the client. We first develop and validate our methodology through controlled experiments in the lab. Then, we conduct a large-scale validation of our approach using network data from a major cellular operator and ground truth QoE metrics from a large video service. We accurately predict the value of average bitrate within a relative error of 10% for 70%-90% of video sessions and re-buffering ratio within 1 percentage point for 65-90% of sessions. We further quantify the network overhead due to video chunk replacement and observe that a significant number of sessions have a high overhead of 20% or more. Finally, we highlight several challenges with video QoE metrics estimation in a large-scale monitoring system.
更多查看译文
关键词
MIMIC,passive network measurements,HTTP-based adaptive video,QoE metrics,HTTP-based adaptive streaming,HAS,cellular networks,network demand driver,user-perceived quality of experience,video streaming,user satisfaction,QoE-aware traffic management
AI 理解论文
溯源树
样例
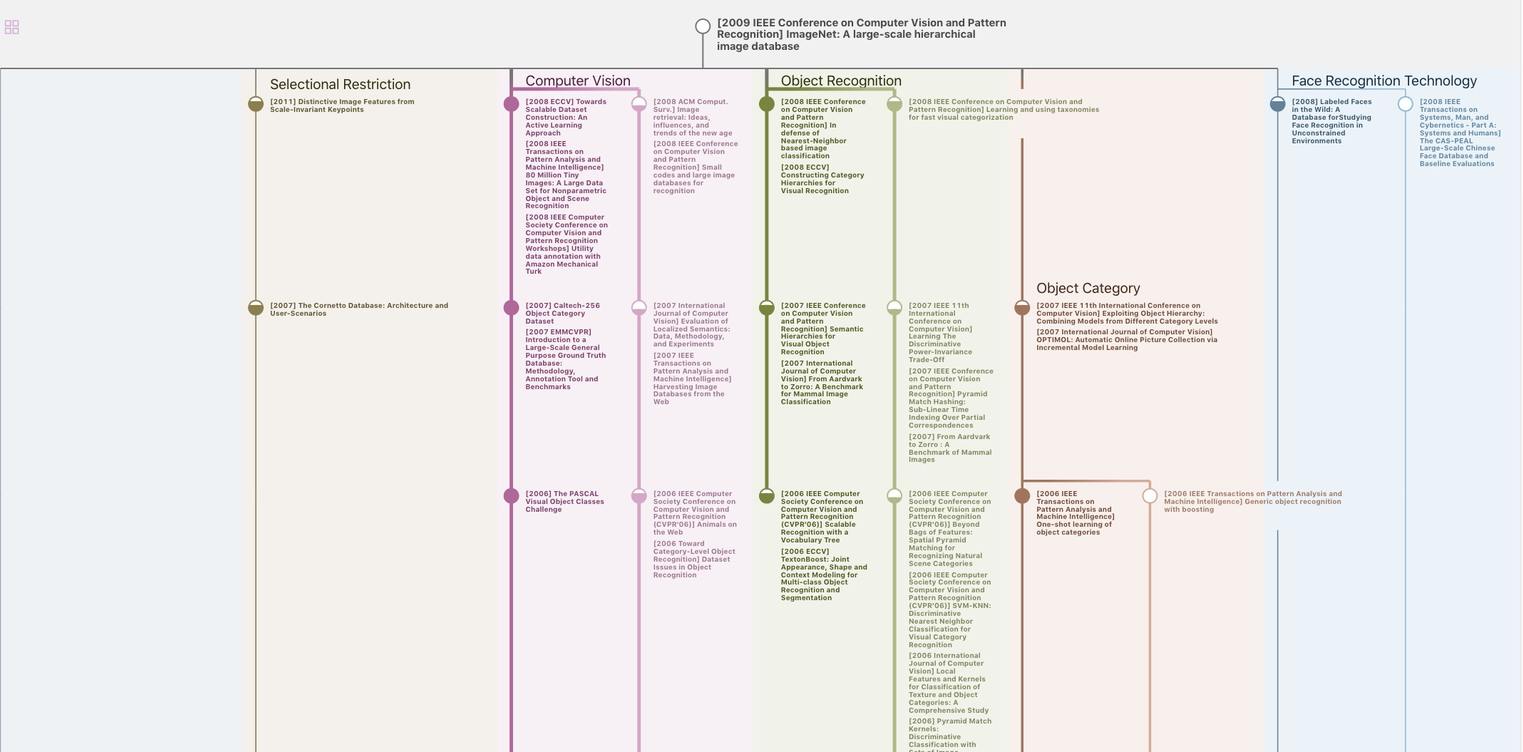
生成溯源树,研究论文发展脉络
Chat Paper
正在生成论文摘要