Bayesian Conditional Generative Adverserial Networks.
arXiv: Learning(2017)
摘要
Traditional GANs use a deterministic generator function (typically a neural network) to transform a random noise input $z$ to a sample $mathbf{x}$ that the discriminator seeks to distinguish. We propose a new GAN called Bayesian Conditional Generative Adversarial Networks (BC-GANs) that use a random generator function to transform a deterministic input $yu0027$ to a sample $mathbf{x}$. Our BC-GANs extend traditional GANs to a Bayesian framework, and naturally handle unsupervised learning, supervised learning, and semi-supervised learning problems. Experiments show that the proposed BC-GANs outperforms the state-of-the-arts.
更多查看译文
AI 理解论文
溯源树
样例
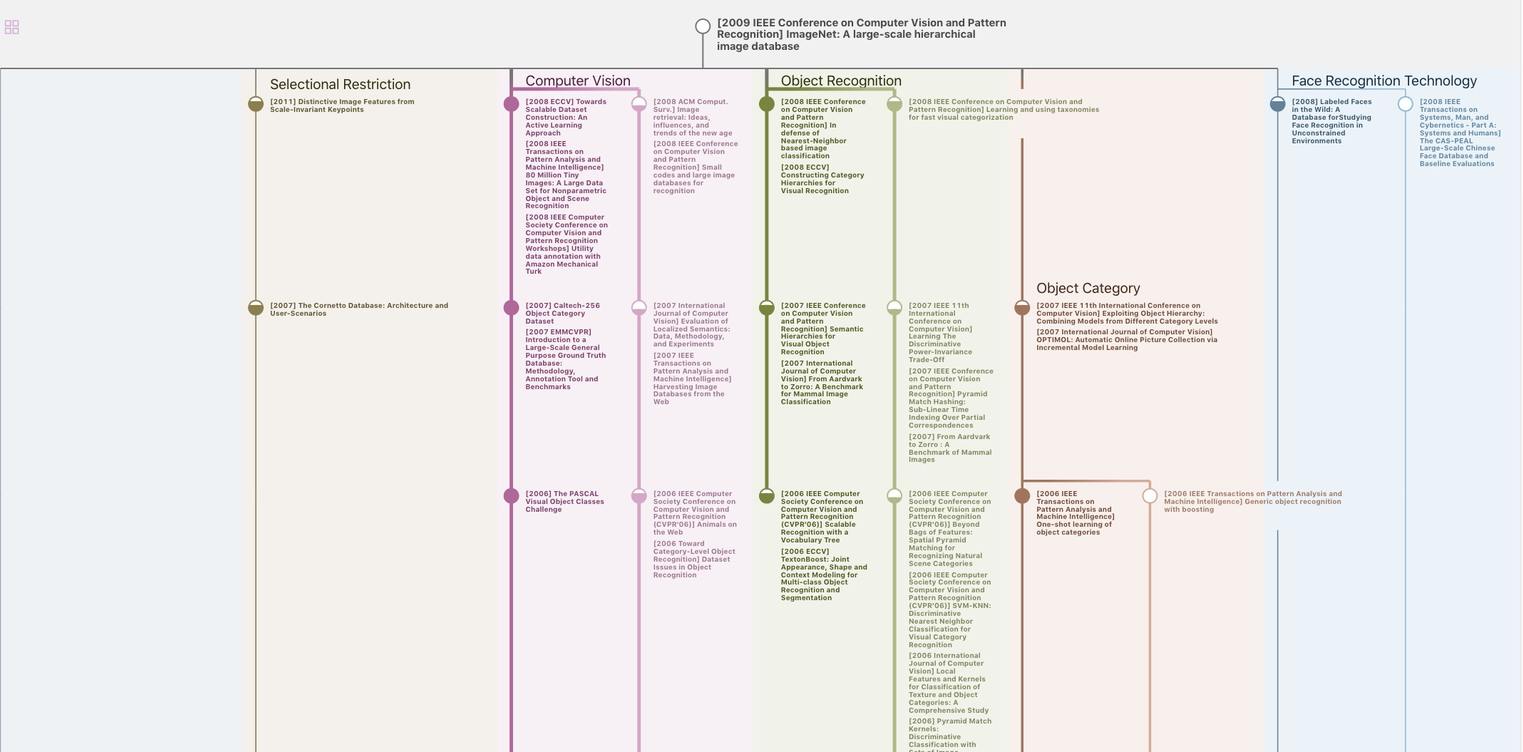
生成溯源树,研究论文发展脉络
Chat Paper
正在生成论文摘要