Performance evaluation of time-frequency image feature sets for improved classification and analysis of non-stationary signals: Application to newborn EEG seizure detection.
Knowledge-Based Systems(2017)
摘要
This study demonstrates that a time-frequency (TF) image pattern recognition approach offers significant advantages over standard signal classification methods that use t-domain only or f-domain only features. Two approaches are considered and compared. The paper describes the significance of the standard TF approach for non-stationary signals; TF signal (TFS) features are defined by extending t-domain or f-domain features to a joint (t, f) domain resulting in e.g. TF flatness and TF flux. The performance of the extended TFS features is comparatively assessed using Receiver Operating Characteristic (ROC) analysis Area Under the Curve (AUC) measure. Experimental results confirm that the extended TFS features generally yield improved performance (up to 19%) when compared to the corresponding t-domain and f-domain features.
更多查看译文
关键词
Machine learning,Non-Stationary signal analysis,Hu invariant moments,Haralick features,Local Binary Patterns,Time-frequency distributions,Random forests
AI 理解论文
溯源树
样例
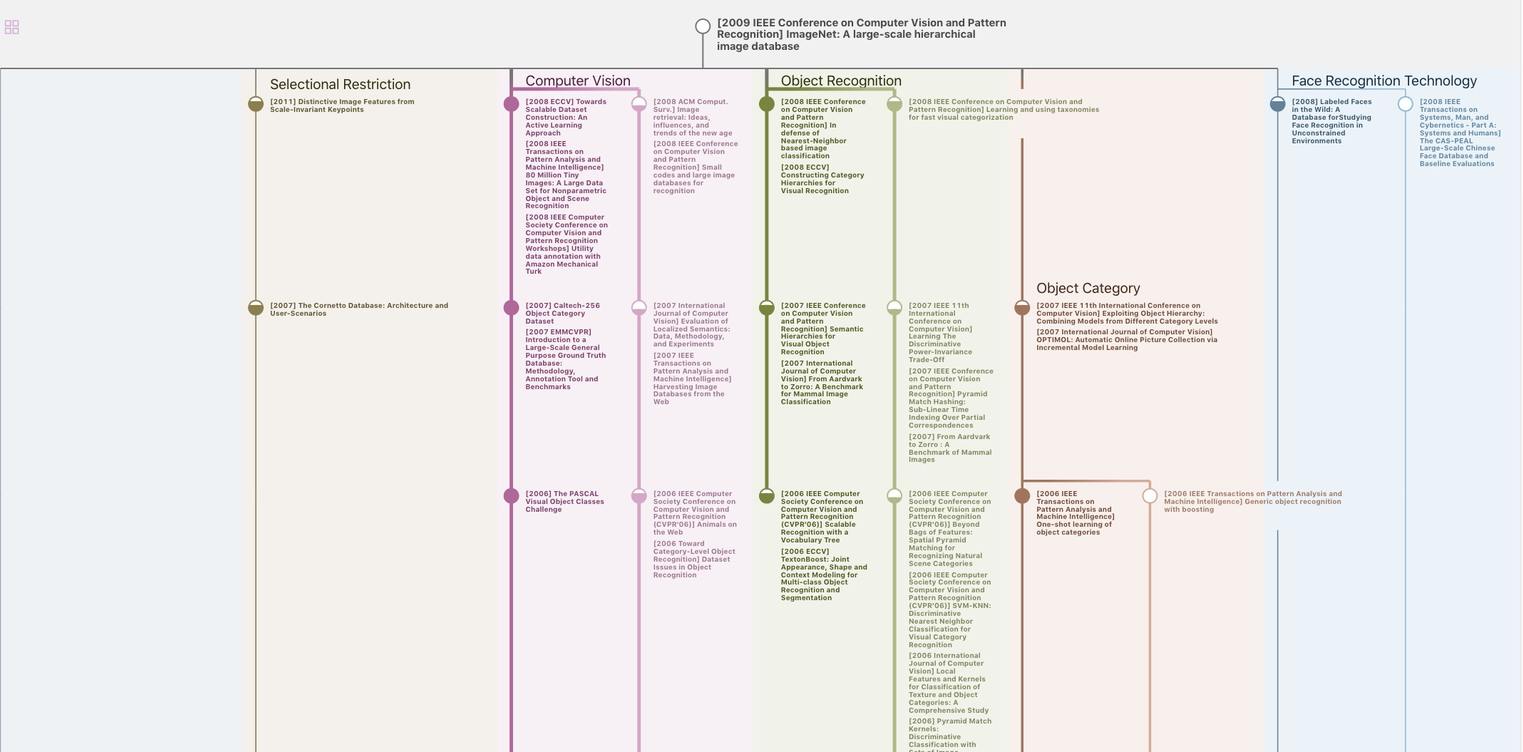
生成溯源树,研究论文发展脉络
Chat Paper
正在生成论文摘要