Dempster-Shafer Evidence Theory-Based CV Model for Renal Lesion Segmentation of Medical Ultrasound Images
JOURNAL OF MEDICAL IMAGING AND HEALTH INFORMATICS(2017)
摘要
In medical image analysis, because of the noises, low contrast, complex textures and inhomogeneities, robust segmentation for objects is a challenging problem. In this paper, a modified variational model is proposed to improve segmentation effects in medical images by separating the regions of interest (ROIs) and backgrounds based on both intensity and texture features. Information from these two sources are fused by the Dempster-Shafer evidence theory and to provide a compelling evidence for object segmentation. Since it can capture the differences of textures as well as intensities between the object and background, the proposed method is effective on segmentation of lesions, even lesion areas that have similar intensities with their surroundings. To prove the efficiency of the proposed method, 52 renal ultrasound images with low contrast and inhomogeneous lesions are utilized in the quantitative evaluations. Compared with the classical CV model, the method using a new distribution metric based on prediction theory, the method using Bayesian theorem to fuse information, the MICO method and RSF method, the proposed method has a mean precision of 94.7%, a mean Dice coefficient of 92.8%, being the highest among all the tested methods, and the mean absolute surface distance (MAD) and the symmetric mean absolute surface distance (SMAD) of this method are 0.11 and 0.13, respectively, which are lower than those of other methods. Comparison results demonstrate that segmentation results by the proposed method are closer to ground truths than those of other methods.
更多查看译文
关键词
Ultrasound Image Segmentation,C-V Model,Gabor Filter,Dempster-Shafer Evidence Theory
AI 理解论文
溯源树
样例
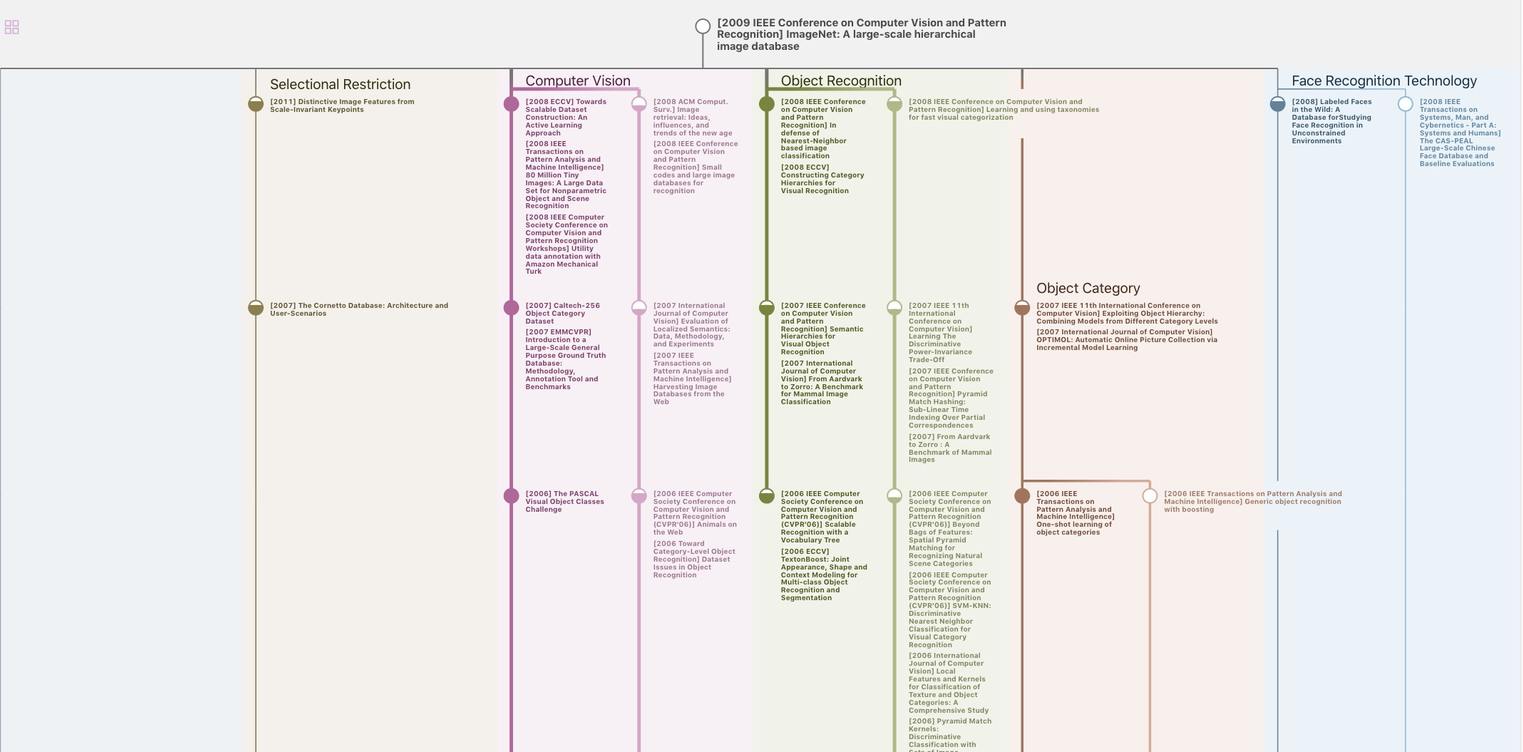
生成溯源树,研究论文发展脉络
Chat Paper
正在生成论文摘要