Predicting virological response to HIV treatment over time: a tool for settings with different definitions of virological response.
JAIDS-JOURNAL OF ACQUIRED IMMUNE DEFICIENCY SYNDROMES(2019)
摘要
Objective: Definitions of virological response vary from <50 up to 1000 copies of HIV-RNA/mL. Our previous models estimate the probability of HIV drug combinations reducing the viral load to <50 copies/mL, with no indication of whether higher thresholds of response may be achieved. Here, we describe the development of models that predict absolute viral load over time. Methods: Two sets of random forest models were developed using 50,270 treatment change episodes from more than 20 countries. The models estimated viral load at different time points following the introduction of a new regimen from variables including baseline viral load, CD4 count, and treatment history. One set also used genotypes in their predictions. Independent data sets were used for evaluation. Results: Both models achieved highly significant correlations between predicted and actual viral load changes (r = 0.67-0.68, mean absolute error of 0.73-0.74 log(10) copies/mL). The models produced curves of virological response over time. Using failure definitions of <100, 400, or 1000 copies/mL, but not 50 copies/mL, both models were able to identify alternative regimens they predicted to be effective for the majority of cases where the new regimen prescribed in the clinic failed. Conclusions: These models could be useful for selecting the optimum combination therapy for patients requiring a change in therapy in settings using any definition of virological response. They also give an idea of the likely response curve over time. Given that genotypes are not required, these models could be a useful addition to the HIV-TRePS system for those in resource-limited settings.
更多查看译文
关键词
HIV/AIDS,antiretroviral therapy,treatment response,viral load,machine learning,data mining
AI 理解论文
溯源树
样例
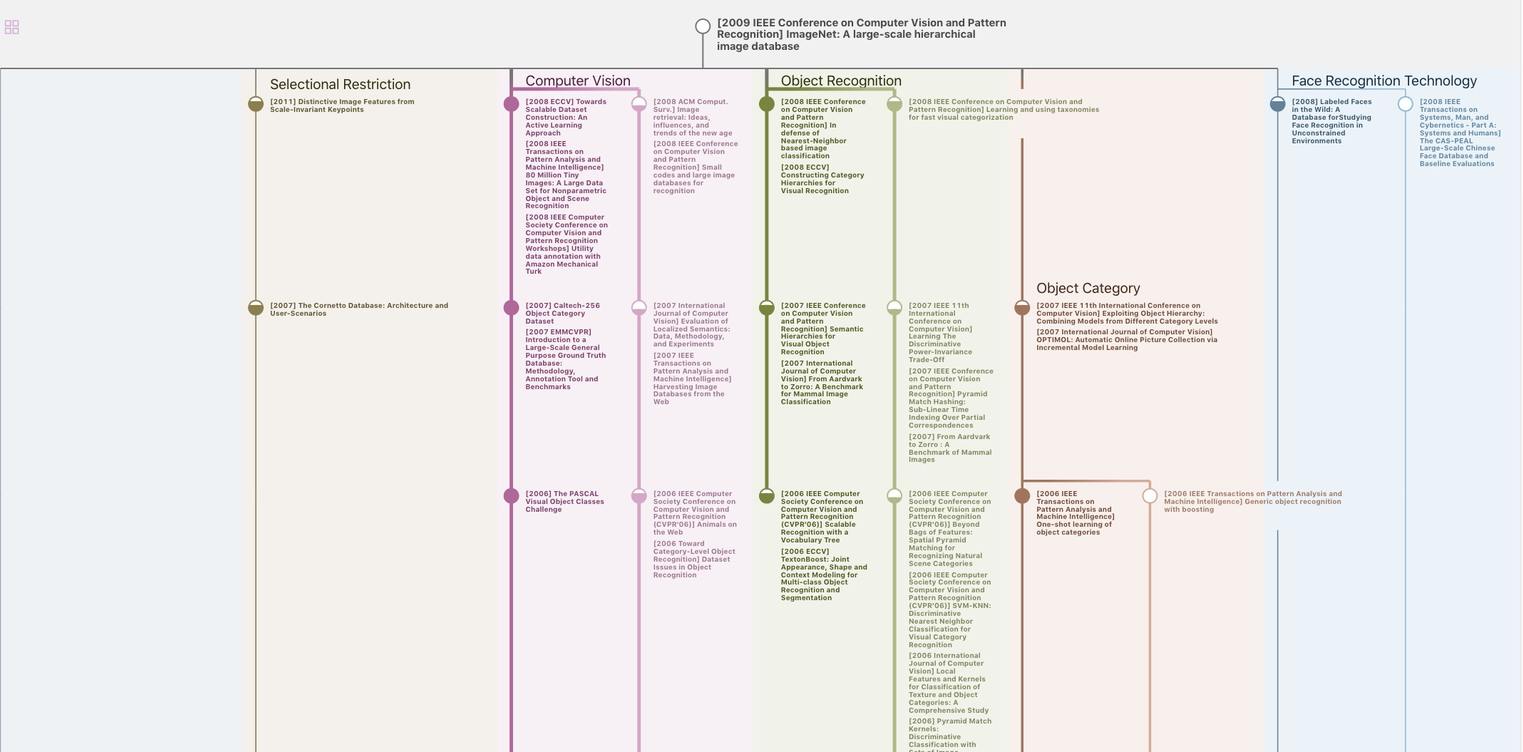
生成溯源树,研究论文发展脉络
Chat Paper
正在生成论文摘要