Multifactor-influenced energy consumption forecasting using enhanced back-propagation neural network
Energy(2017)
摘要
Reliable energy consumption forecasting can provide effective decision-making support for planning development strategies to energy enterprises and for establishing national energy policies. Accordingly, the present study aims to apply a hybrid intelligent approach named ADE–BPNN, the back-propagation neural network (BPNN) model supported by an adaptive differential evolution algorithm, to estimate energy consumption. Most often, energy consumption is influenced by socioeconomic factors. The proposed hybrid model incorporates gross domestic product, population, import, and export data as inputs. An improved differential evolution with adaptive mutation and crossover is utilized to find appropriate global initial connection weights and thresholds to enhance the forecasting performance of the BPNN. A comparative example and two extended examples are utilized to validate the applicability and accuracy of the proposed ADE–BPNN model. Errors of the test data sets indicate that the ADE–BPNN model can effectively predict energy consumption compared with the traditional back-propagation neural network model and other popular existing models. Moreover, mean impact value based analysis is conducted for electrical energy consumption in U.S. and total energy consumption forecasting in China to quantitatively explore the relative importance of each input variable for the improvement of effective energy consumption prediction.
更多查看译文
关键词
Energy consumption,Multifactor-influenced forecasting,Back-propagation neural network,Adaptive differential evolution,Mean impact value
AI 理解论文
溯源树
样例
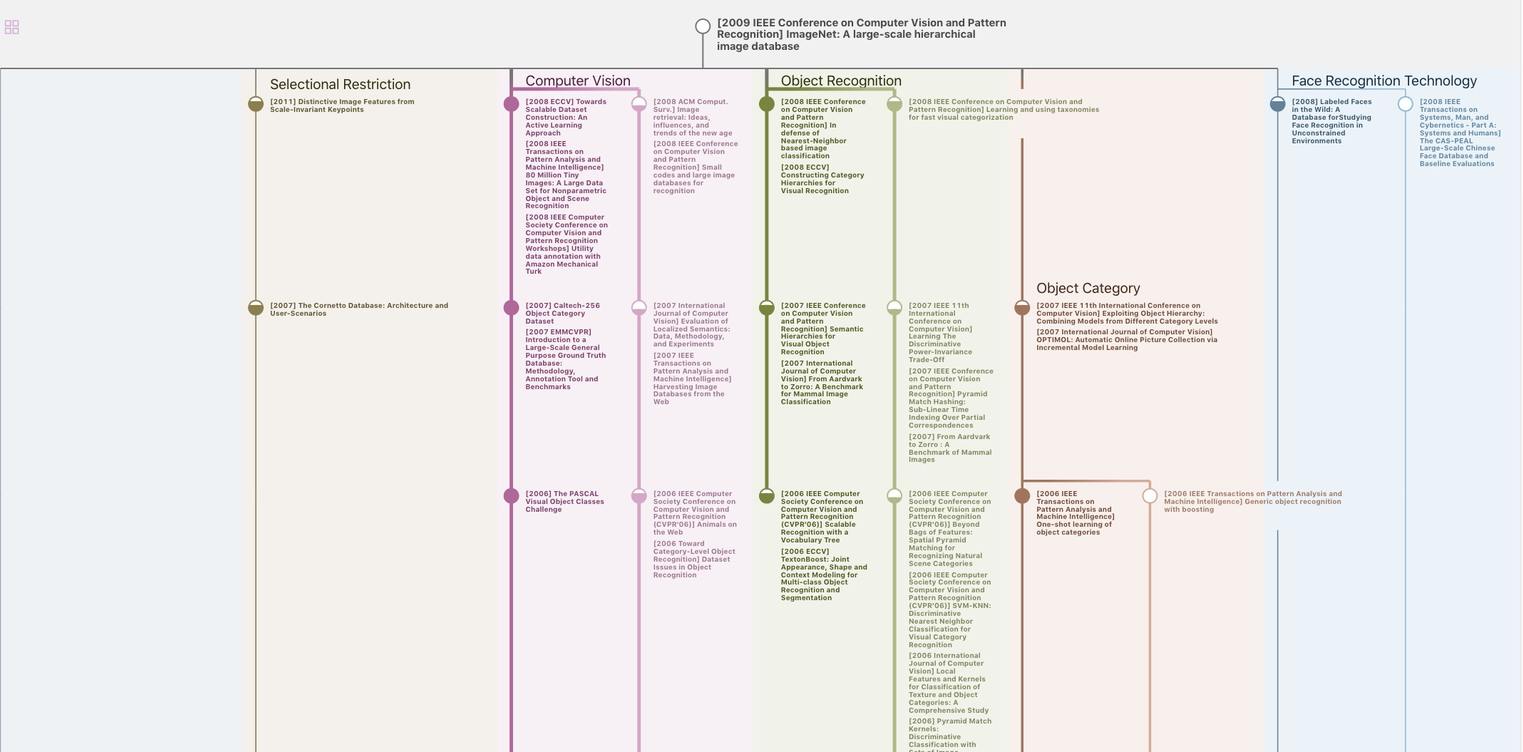
生成溯源树,研究论文发展脉络
Chat Paper
正在生成论文摘要