Context-Based Concurrent Experience Sharing in Multiagent Systems.
AAMAS(2017)
摘要
One of the key challenges for multi-agent learning is scalability. We introduce a technique for speeding up multi-agent learning by exploiting concurrent and incremental experience sharing. This solution adaptively identifies opportunities to transfer experiences between agents and allows for the rapid acquisition of appropriate policies in large-scale, stochastic, multi-agent systems. We introduce an online, supervisor-directed transfer technique for constructing high-level characterizations of an agent's dynamic learning environment---called contexts---which are used to identify groups of agents operating under approximately similar dynamics within a short temporal window. Supervisory agents compute contextual information for groups of subordinate agents, thereby identifying candidates for experience sharing. We show that our approach results in significant performance gains, that it is robust to noise-corrupted or suboptimal context features, and that communication costs scale linearly with the supervisor-to-subordinate ratio.
更多查看译文
关键词
Transfer Learning,Multi-agent Systems,Reinforcement Learning
AI 理解论文
溯源树
样例
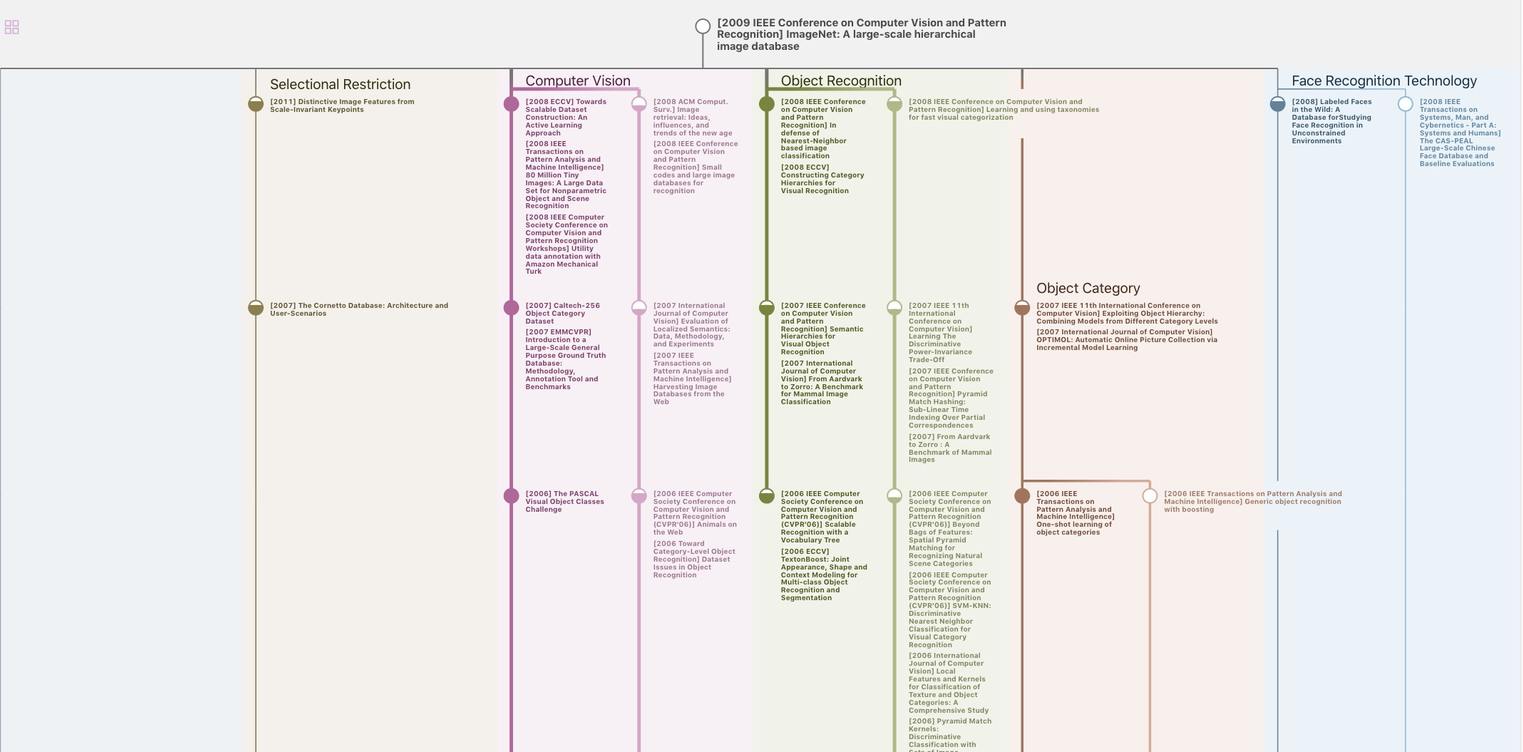
生成溯源树,研究论文发展脉络
Chat Paper
正在生成论文摘要