SLDP: Sequence learning dependency parsing model using long short-term memory
2016 International Conference on Machine Learning and Cybernetics (ICMLC)(2016)
摘要
Recent work on neural network models shows success in dependency parsing. In this paper, we present a sequence learning dependency parsing (SLDP) model using long short-term memory for shift-reduce parser. A feed-forward neural network is used to build greedy model from rich local features. With the features extracted by the local model, we further train a long short-term memory (LSTM) model optimized for global parsing sequences. Our model has the capability of learning not only atomic feature combinations automatically but also the long distance dependent information for dependency parsing. Experiments on English Penn Treebank show that our SLDP model significantly outperforms the baseline, achieving 90.7% unlabeled attachment score and 89.0% labeled attachment score.
更多查看译文
关键词
Dependency parsing,Neural networks,Long short-term memory,Natural language processing,Syntactic parsing
AI 理解论文
溯源树
样例
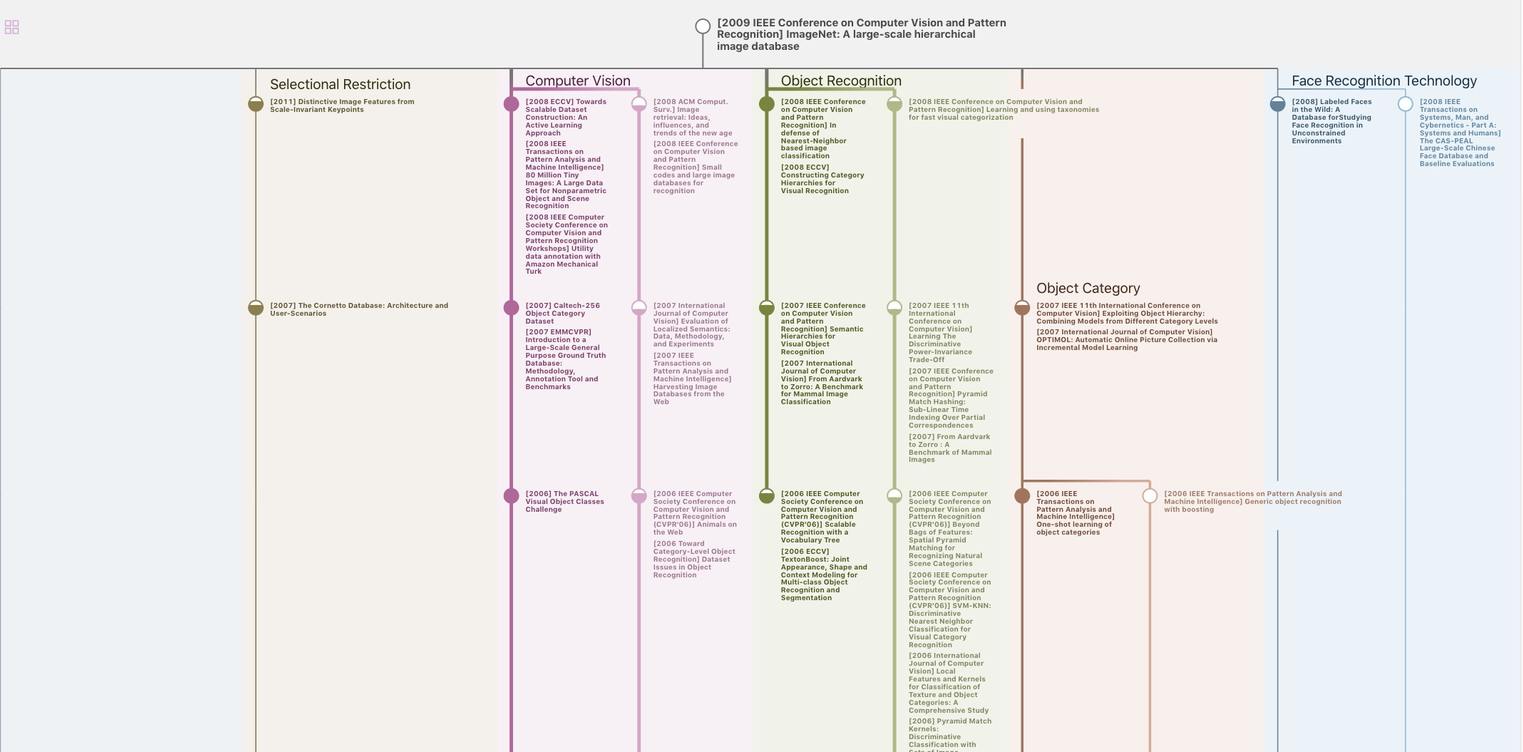
生成溯源树,研究论文发展脉络
Chat Paper
正在生成论文摘要