Memory Warps For Long-Term Online Video Representations And Anticipation
2019 IEEE WINTER CONFERENCE ON APPLICATIONS OF COMPUTER VISION (WACV)(2019)
摘要
We propose a novel memory-based online video representation that is efficient, accurate and predictive. This is in contrast to prior works that often rely on computationally heavy 3D convolutions, ignore motion when aligning features over time, or operate in an off-line mode to utilize future frames. In particular, our memory (i) holds the feature representation, (ii) is spatially warped over time to compensate for observer and scene motions, (iii) can carry long-term information, and (iv) enables predicting feature representations in future frames. By exploring a variant that operates at multiple temporal scales, we efficiently learn across even longer time horizons. We apply our online framework to object detection in videos, obtaining a large 2.3 times speed-up and losing only 0.9% mAP on ImageNet-VID dataset, compared to prior works that even use future frames. Finally, we demonstrate the predictive property of our representation in two novel detection setups, where features are propagated over time to (i) significantly enhance a real-time detector by more than 10% mAP in a multi-threaded online setup and to (ii) anticipate objects in future frames.
更多查看译文
关键词
memory warps,feature representation,observer,scene motions,online video representation,ImageNet-VID dataset,object detection,multithreaded online
AI 理解论文
溯源树
样例
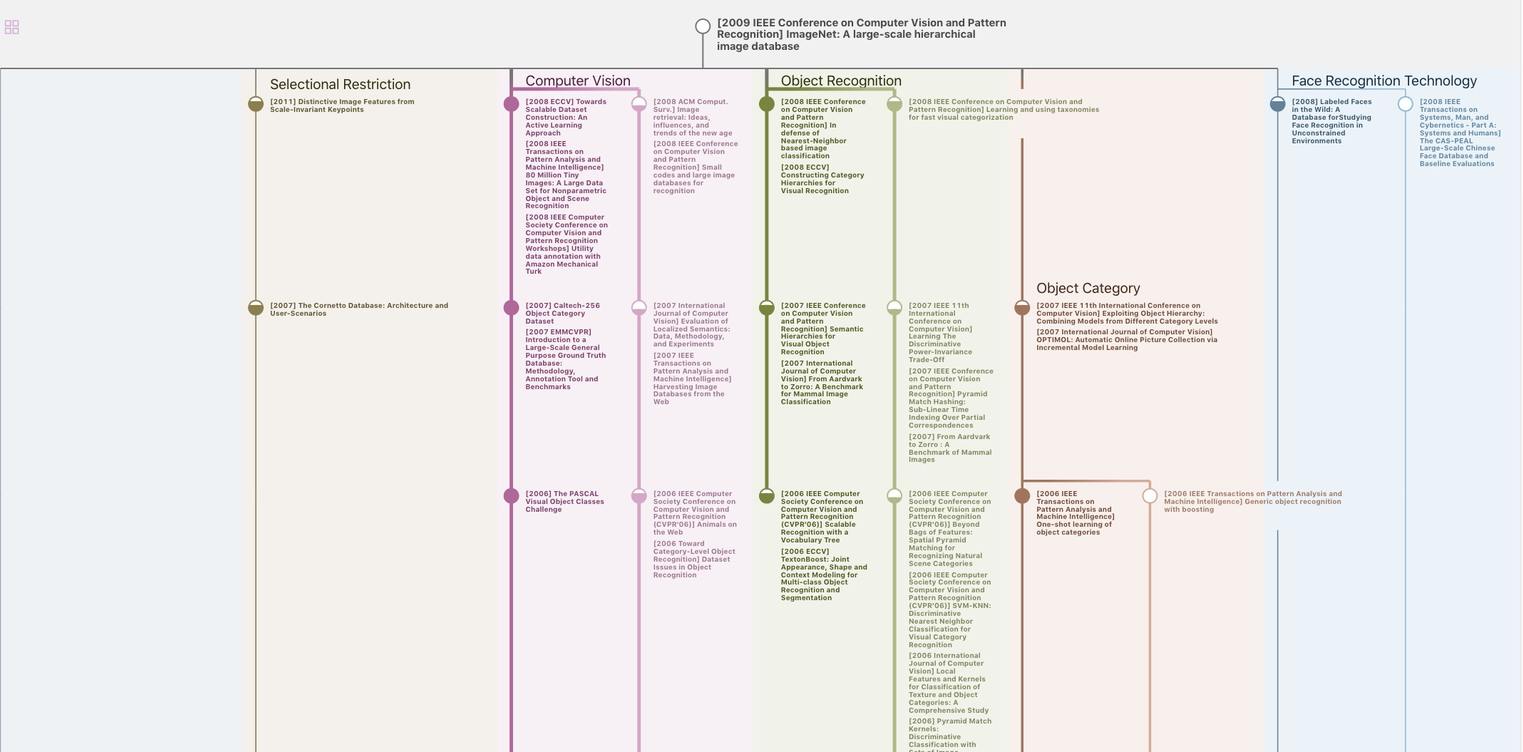
生成溯源树,研究论文发展脉络
Chat Paper
正在生成论文摘要