Deep-Hurricane-Tracker: Tracking And Forecasting Extreme Climate Events
2019 IEEE WINTER CONFERENCE ON APPLICATIONS OF COMPUTER VISION (WACV)(2019)
摘要
Tracking and predicting extreme events in large-scale spatio-temporal climate data are long standing challenges in climate science. In this paper, we propose Convolutional LSTM (ConvLSTM)-based spatio-temporal models to track and predict hurricane trajectories from large-scale climate data; namely, pixel-level spatio-temporal history of tropical cyclones. To address the tracking problem, we model time-sequential density maps of hurricane trajectories, enabling to capture not only the temporal dynamics but also spatial distribution of the trajectories. Furthermore, we introduce a new trajectory prediction approach as a problem of sequential forecasting from past to future hurricane density map sequences. Extensive experiment on actual 20 years record shows that our ConvLSTM-based tracking model significantly outperforms existing approaches, and that the proposed forecasting model achieves successful mapping from predicted density map to ground truth.
更多查看译文
关键词
pixel-level spatio-temporal history,tropical cyclones,tracking problem,model time-sequential density maps,hurricane trajectories,temporal dynamics,trajectory prediction approach,sequential forecasting,future hurricane density map sequences,forecasting model,predicted density map,deep-hurricane-tracker,extreme climate events,large-scale spatio-temporal climate data,climate science,large-scale climate data,convolutional LSTM-based spatio-temporal models,ConvLSTM-based tracking model
AI 理解论文
溯源树
样例
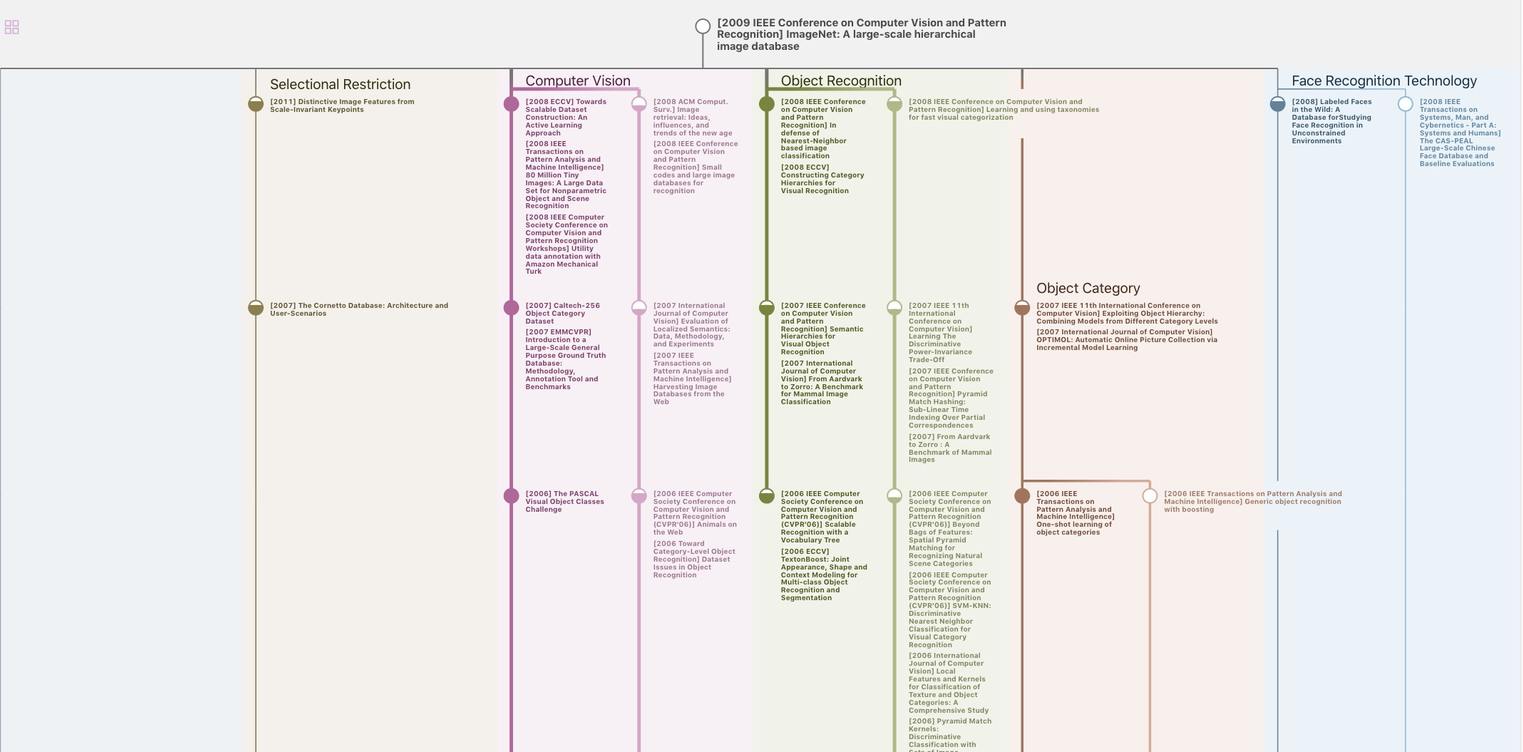
生成溯源树,研究论文发展脉络
Chat Paper
正在生成论文摘要