Optimal knockout strategies in genome-scale metabolic networks using particle swarm optimization
BMC Bioinformatics(2017)
摘要
Background Knockout strategies, particularly the concept of constrained minimal cut sets (cMCSs), are an important part of the arsenal of tools used in manipulating metabolic networks. Given a specific design, cMCSs can be calculated even in genome-scale networks. We would however like to find not only the optimal intervention strategy for a given design but the best possible design too. Our solution (PSOMCS) is to use particle swarm optimization (PSO) along with the direct calculation of cMCSs from the stoichiometric matrix to obtain optimal designs satisfying multiple objectives. Results To illustrate the working of PSOMCS, we apply it to a toy network. Next we show its superiority by comparing its performance against other comparable methods on a medium sized E. coli core metabolic network. PSOMCS not only finds solutions comparable to previously published results but also it is orders of magnitude faster. Finally, we use PSOMCS to predict knockouts satisfying multiple objectives in a genome-scale metabolic model of E. coli and compare it with OptKnock and RobustKnock. Conclusions PSOMCS finds competitive knockout strategies and designs compared to other current methods and is in some cases significantly faster. It can be used in identifying knockouts which will force optimal desired behaviors in large and genome scale metabolic networks. It will be even more useful as larger metabolic models of industrially relevant organisms become available.
更多查看译文
关键词
Dual metabolic network,Knockouts,Metabolic networks,Metabolic pathway analysis,Minimal cut sets,Strain optimization,Systems biology
AI 理解论文
溯源树
样例
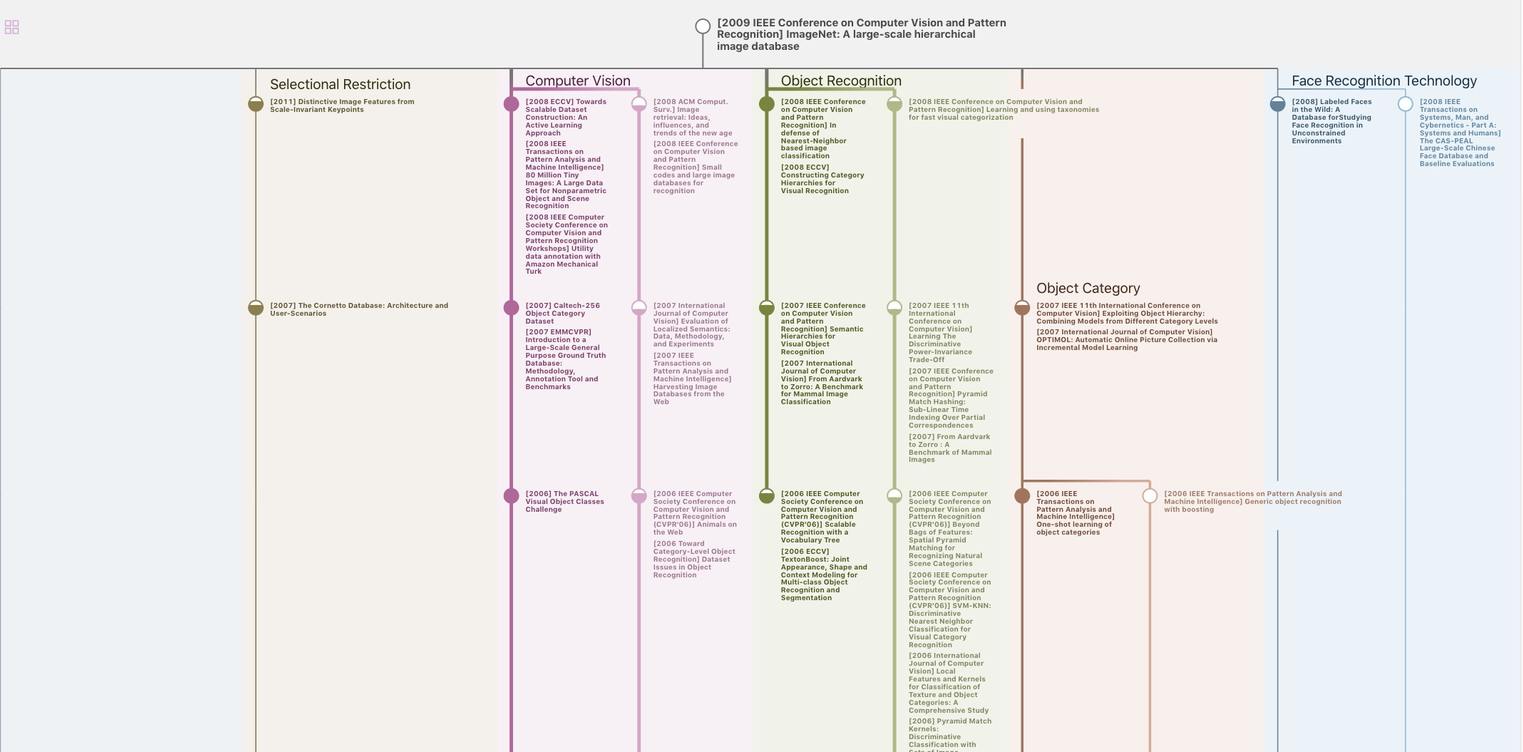
生成溯源树,研究论文发展脉络
Chat Paper
正在生成论文摘要