Subspace selection for anomaly detection: An information theory approach
2016 IEEE International Conference on the Science of Electrical Engineering (ICSEE)(2016)
摘要
We present a novel subspace selection algorithm for anomaly detection. Our method is based on the observation that it is easier to detect anomalies in subspaces comprise of highly correlative attributes. More specifically, it uses the Rokhlin metric [22] to evaluate the smallest information distance in the case of two attributes, and an extension of the Rokhlin distance in cases where more than two attributes are involved. In order to determine the set of subspaces to use, we apply a variation of the well known agglomerative clustering algorithm with the extended Rokhlin metric as the underlying distance function. An extensive evaluation that we conducted demonstrates that in most cases: (1) Our method outperforms state-of-the-art subspace selection algorithms for anomaly detection. (2) Our method yields significantly fewer subspaces (on average) than the other approaches, and (3) Our method does not require any tuning of parameters.
更多查看译文
关键词
information theory,subspace selection algorithm,anomaly detection,Rokhlin distance,agglomerative clustering algorithm,extended Rokhlin metric,distance function
AI 理解论文
溯源树
样例
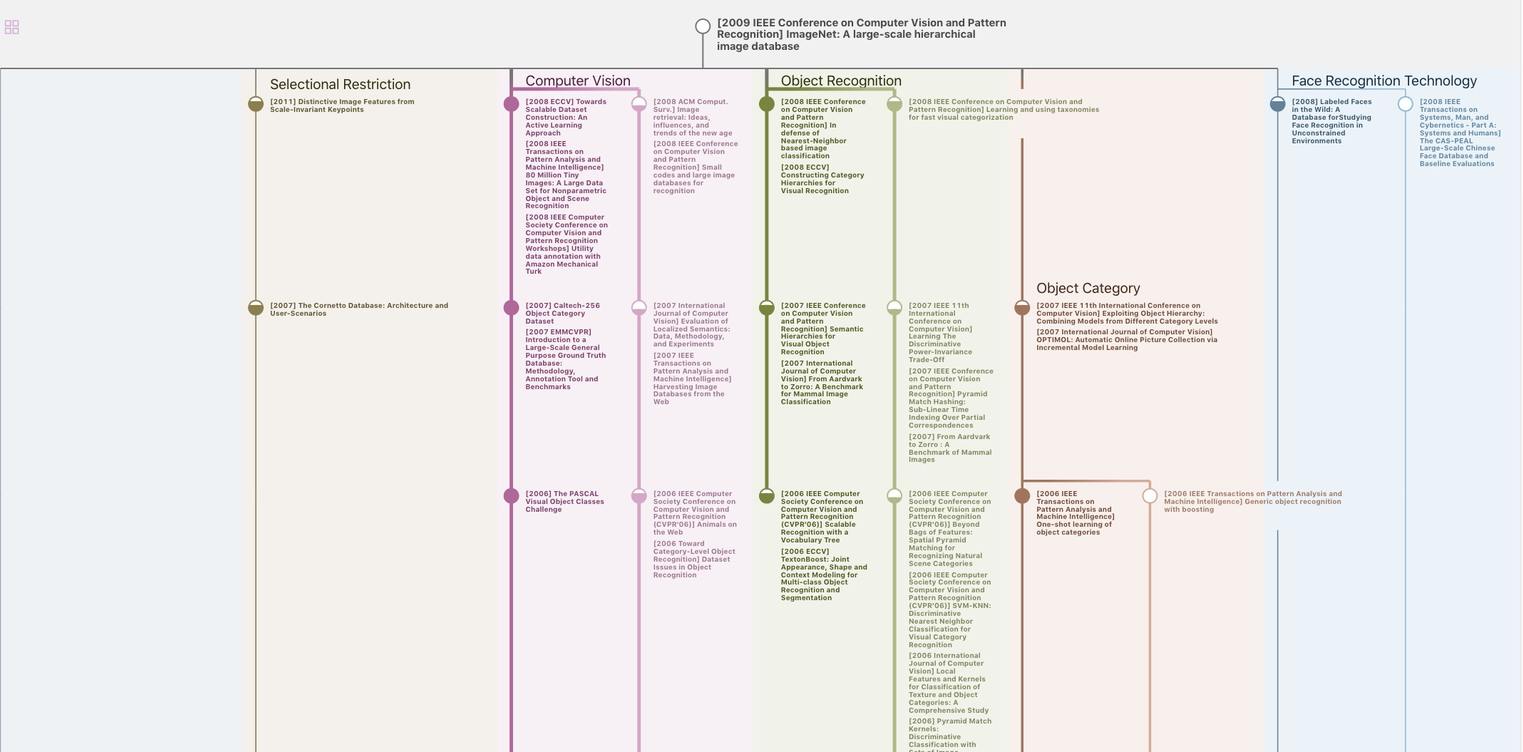
生成溯源树,研究论文发展脉络
Chat Paper
正在生成论文摘要